Modeling the Relative Visual Tempo for Self-supervised Skeleton-based Action Recognition.
Proceedings of the IEEE/CVF International Conference on Computer Vision (ICCV)(2023)
摘要
Visual tempo characterizes the dynamics and the temporal evolution, which helps describe actions. Recent approaches directly perform visual tempo prediction on skeleton sequences, which may suffer from insufficient feature representation issue. In this paper, we observe that relative visual tempo is more in line with human intuition, and thus providing more effective supervision signals. Based on this, we propose a novel Relative Visual Tempo Contrastive Learning framework for skeleton action Representation (RVTCLR). Specifically, we design a Relative Visual Tempo Learning (RVTL) task to explore the motion information in intra-video clips, and an Appearance-Consistency (AC) task to learn appearance information simultaneously, resulting in more representative spatiotemporal features. Furthermore, skeleton sequence data is much sparser than RGB data, making the network learn shortcuts, and overfit to low-level information such as skeleton scales. To learn high-order semantics, we further design a new Distribution-Consistency (DC) branch, containing three components: Skeleton-specific Data Augmentation (S-DA), Fine-grained Skeleton Encoding Module (FSEM), and Distribution-aware Diversity (DD) Loss. We term our entire method (RVTCLR with DC) as RVTCLR+. Extensive experiments on NTU RGB+D 60 and NTU RGB+D 120 datasets demonstrate that our RVTCLR+ can achieve competitive results over the state-of-the-art methods. Code is available at https://github.com/Zhuysheng/RVTCLR.
更多查看译文
AI 理解论文
溯源树
样例
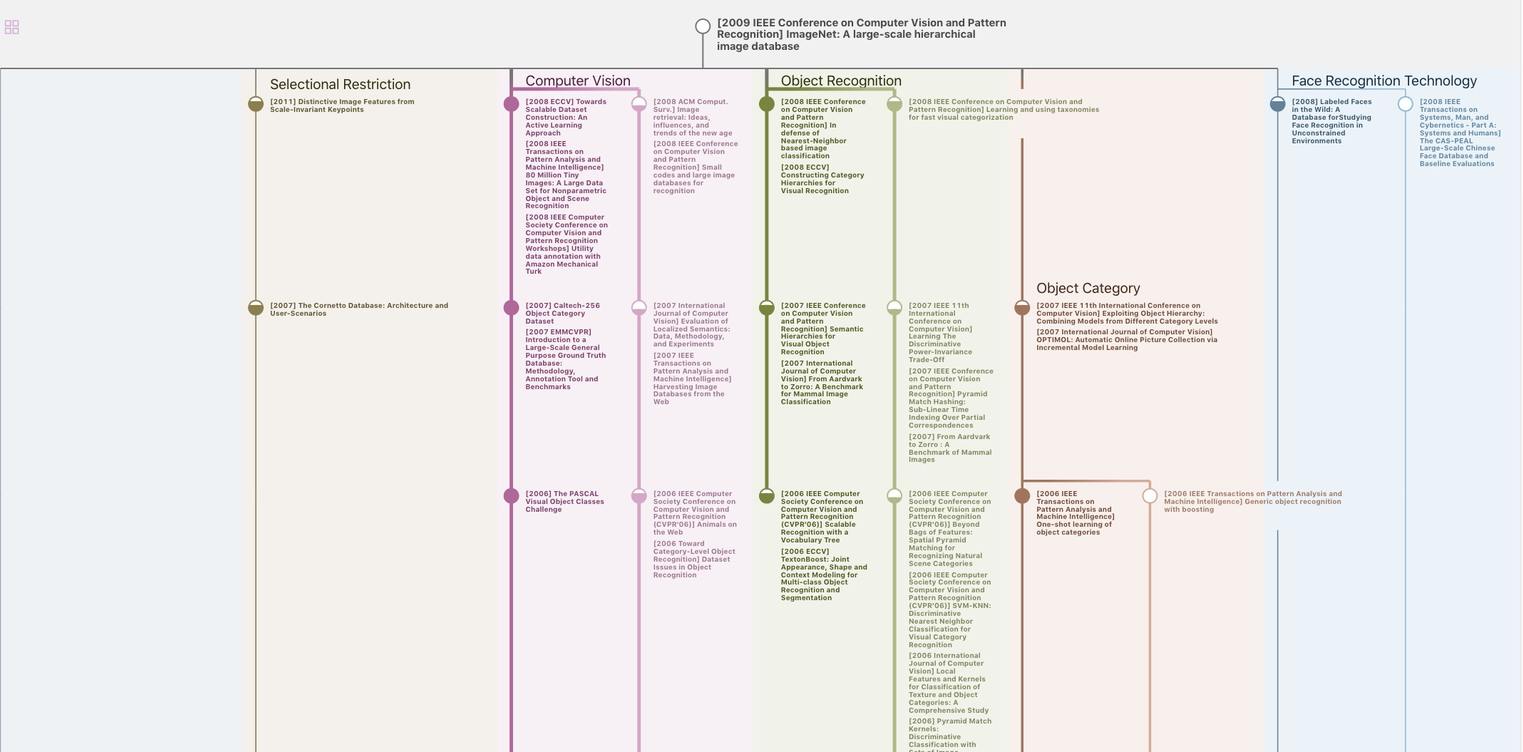
生成溯源树,研究论文发展脉络
Chat Paper
正在生成论文摘要