Few-Shot Common Action Localization via Cross-Attentional Fusion of Context and Temporal Dynamics.
Proceedings of the IEEE/CVF International Conference on Computer Vision (ICCV)(2023)
摘要
The goal of this paper is to localize action instances in a long untrimmed query video using just meager trimmed support videos representing a common action whose class information is not given. In this task, it is crucial to mine reliable temporal cues representing a common action from handful support videos. In our work, we develop an attention mechanism using cross-correlation. Based on this cross-attention, we first transform the support videos into query video’s context to emphasize query-relevant important frames, and suppress less relevant ones. Next, we summarize sub-sequences of support video frames to represent temporal dynamics in coarse temporal granularity, which is then propagated to the fine-grained support video features through the cross-attention. In each case, the cross-attentions are applied to each support video in the individual-to-all strategy to balance heterogeneity and compatibility of the support videos. In contrast, the candidate instances in the query video are lastly attended by the resulting support video features, at once. In addition, we also develop a relational classifier head based on the query and support video representations. We show the effectiveness of our work with the state-of-the-art (SOTA) performance in benchmark datasets (ActivityNet1.3 and THUMOS14), and analyze each component extensively.
更多查看译文
AI 理解论文
溯源树
样例
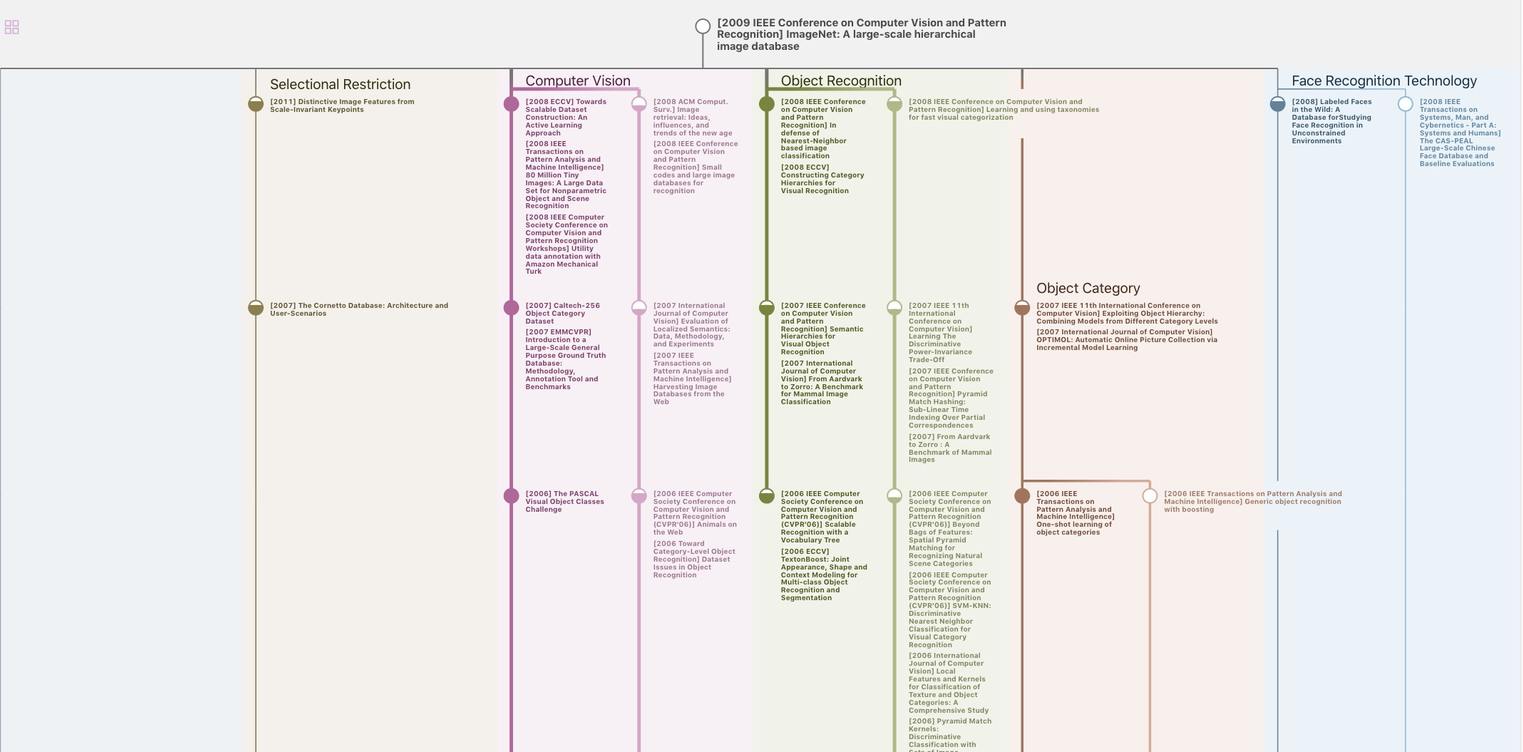
生成溯源树,研究论文发展脉络
Chat Paper
正在生成论文摘要