Tight Time-Space Lower Bounds for Constant-Pass Learning
2023 IEEE 64TH ANNUAL SYMPOSIUM ON FOUNDATIONS OF COMPUTER SCIENCE, FOCS(2023)
摘要
In his breakthrough paper, Raz showed that any parity learning algorithm requires either quadratic memory or an exponential number of samples [FOCS'16, JACM'19]. A line of work that followed extended this result to a large class of learning problems. Until recently, all these results considered learning in the streaming model, where each sample is drawn independently, and the learner is allowed a single pass over the stream of samples. Garg, Raz, and Tal [CCC'19] considered a stronger model, allowing multiple passes over the stream. In the 2-pass model, they showed that learning parities of size n requires either a memory of size n(1.5) or at least 2(root n) samples. (Their result also generalizes to other learning problems.) In this work, for any constant q, we prove tight memory-sample lower bounds for any parity learning algorithm that makes q passes over the stream of samples. We show that such a learner requires either Omega(n(2)) memory size or at least 2(Omega(n)) samples. Beyond establishing a tight lower bound, this is the first non-trivial lower bound for q-pass learning for any q >= 3. Similar to prior work, our results extend to any learning problem with many nearly-orthogonal concepts. We complement the lower bound with an upper bound, showing that parity learning with q passes can be done efficiently with O(n(2)/log q) memory.
更多查看译文
关键词
Multi-pass,streaming,parity learning
AI 理解论文
溯源树
样例
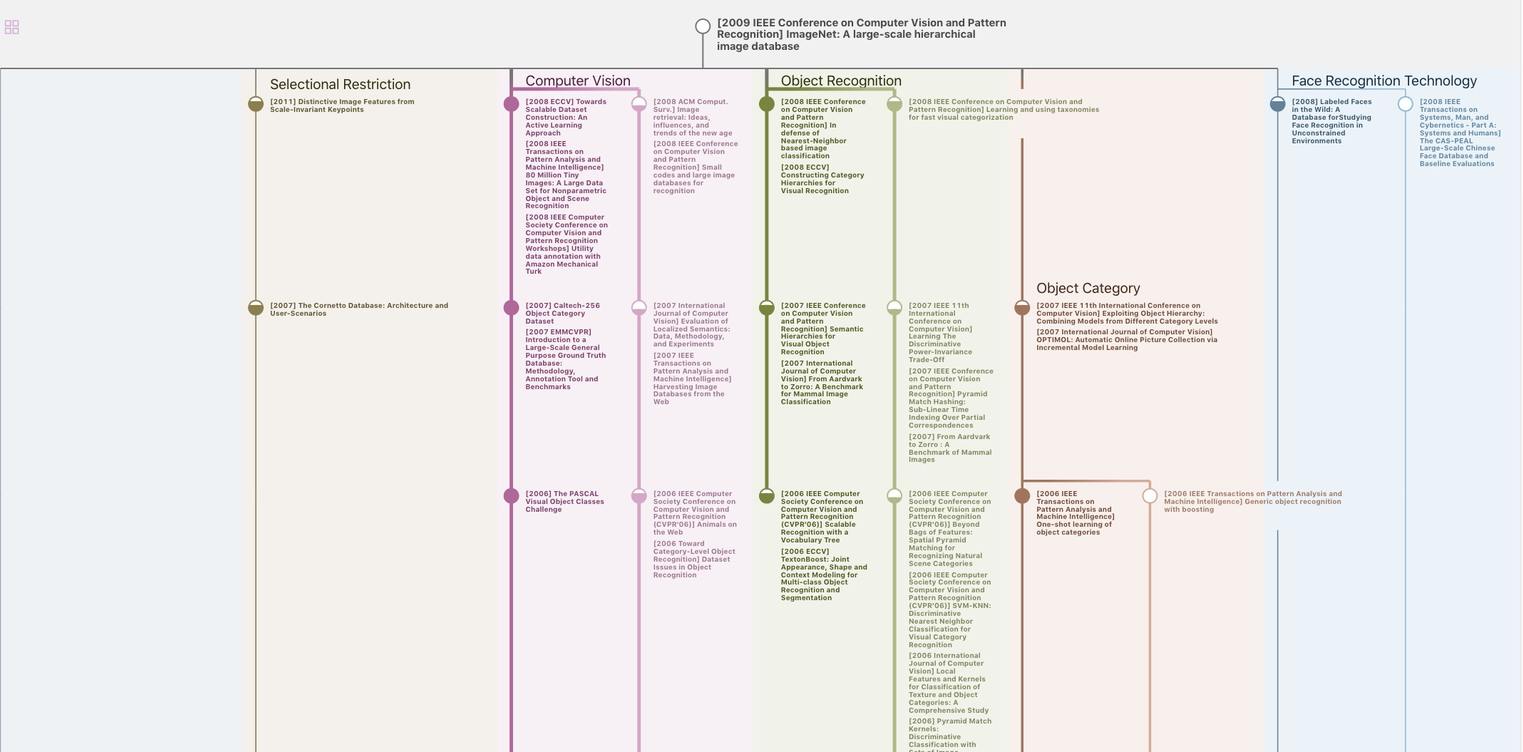
生成溯源树,研究论文发展脉络
Chat Paper
正在生成论文摘要