MGGAN: A multi-generator generative adversarial network for breast cancer immunohistochemical image generation
HELIYON(2023)
摘要
The immunohistochemical technique (IHC) is widely used for evaluating diagnostic markers, but it can be expensive to obtain IHC-stained section. Translating the cheap and easily available hematoxylin and eosin (HE) images into IHC images provides a solution to this challenge. In this paper, we propose a multi-generator generative adversarial network (MGGAN) that can generate high-quality IHC images based on the HE of breast cancer. Our MGGAN approach combines the low-frequency and high-frequency components of the HE image to improve the translation of breast cancer image details. We use the multi-generator to extract semantic information and a U-shaped architecture and patch-based discriminator to collect and optimize the low-frequency and high-frequency components of an image. We also include a cross-entropy loss as a regularization term in the loss function to ensure consistency between the synthesized image and the real image. Our experimental and visualization results demonstrate that our method outperforms other state -of-the-art image synthesis methods in terms of both quantitative and qualitative analysis. Our approach provides a cost-effective and efficient solution for obtaining high-quality IHC images.
更多查看译文
关键词
Immunohistochemical techniques,Hematoxylin and eosin,Generative adversarial network,Multi-generator,Patch-based discriminator
AI 理解论文
溯源树
样例
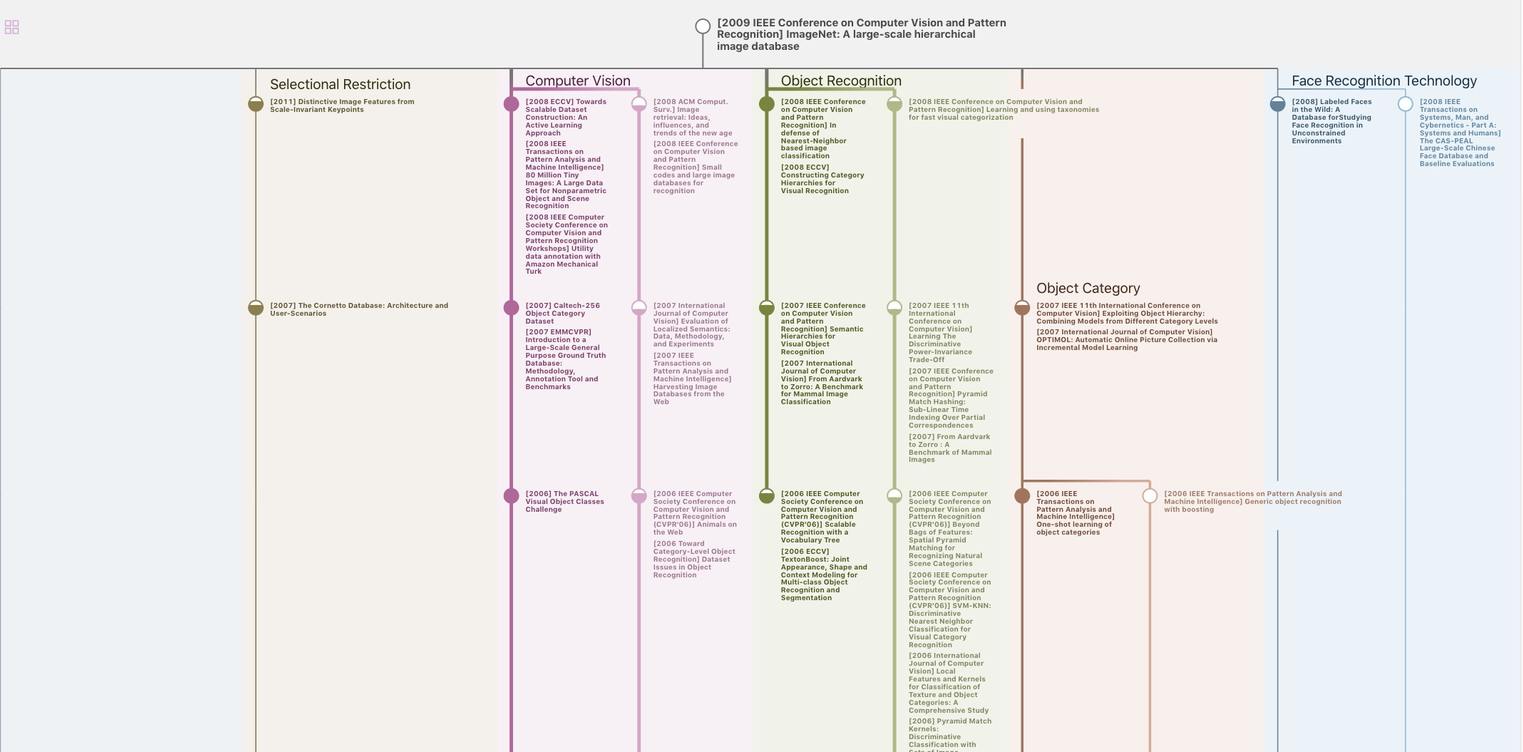
生成溯源树,研究论文发展脉络
Chat Paper
正在生成论文摘要