MDSR-NMF: Multiple deconstruction single reconstruction deep neural network model for non-negative matrix factorization.
Network (Bristol, England)(2023)
摘要
Dimension reduction is one of the most sought-after strategies to cope with high-dimensional ever-expanding datasets. To address this, a novel deep-learning architecture has been designed with multiple deconstruction and single reconstruction layers for non-negative matrix factorization aimed at low-rank approximation. This design ensures that the reconstructed input matrix has a unique pair of factor matrices. The two-stage approach, namely, pretraining and stacking, aids in the robustness of the architecture. The sigmoid function has been adjusted in such a way that fulfils the non-negativity criteria and also helps to alleviate the data-loss problem. Xavier initialization technique aids in the solution of the exploding or vanishing gradient problem. The objective function involves regularizer that ensures the best possible approximation of the input matrix. The superior performance of MDSR-NMF, over six well-known dimension reduction methods, has been demonstrated extensively using five datasets for classification and clustering. Computational complexity and convergence analysis have also been presented to establish the model.
更多查看译文
关键词
single reconstruction,deep neural network model,deep neural network,multiple deconstruction,mdsr-nmf,non-negative
AI 理解论文
溯源树
样例
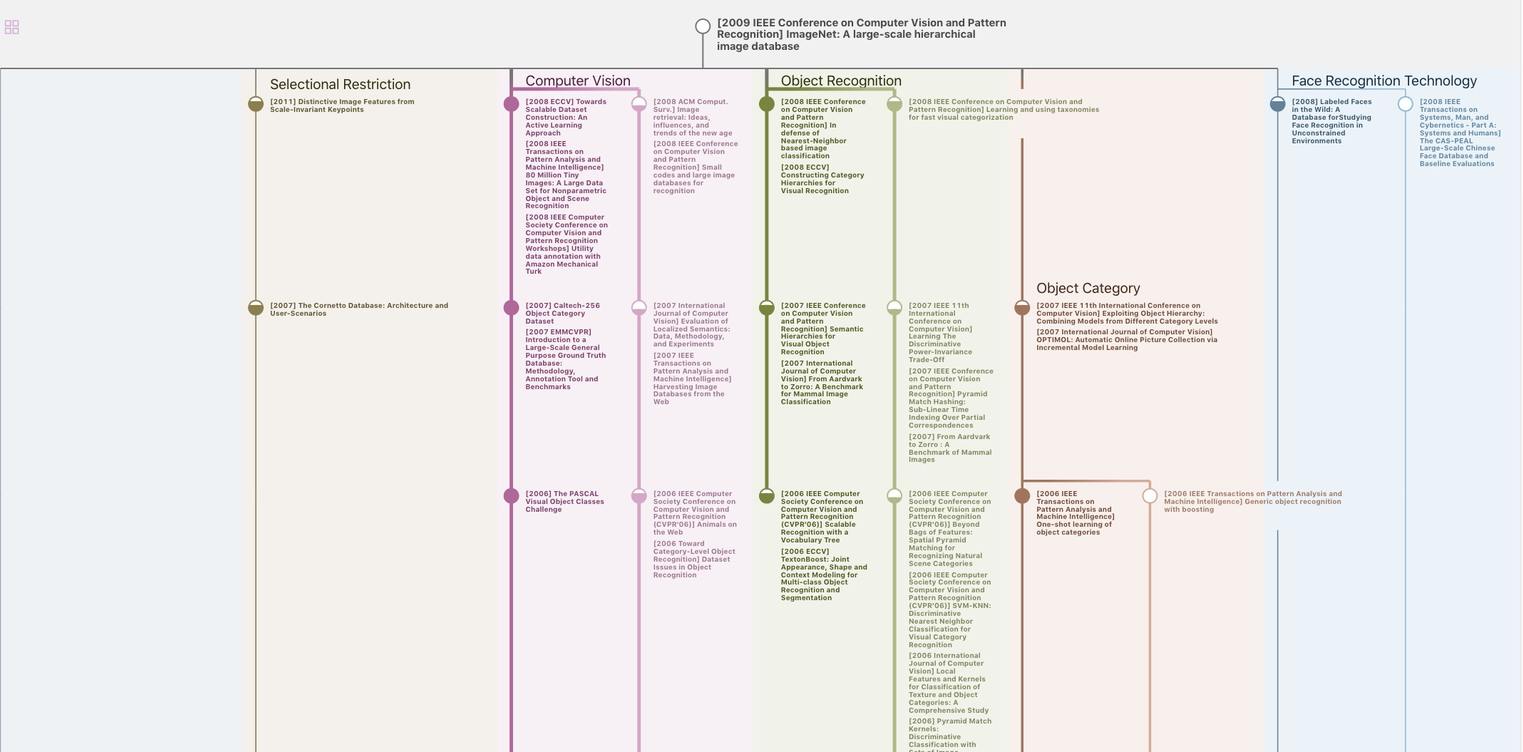
生成溯源树,研究论文发展脉络
Chat Paper
正在生成论文摘要