Attribute Localization and Revision Network for Zero-Shot Learning
CoRR(2023)
摘要
Zero-shot learning enables the model to recognize unseen categories with the aid of auxiliary semantic information such as attributes. Current works proposed to detect attributes from local image regions and align extracted features with class-level semantics. In this paper, we find that the choice between local and global features is not a zero-sum game, global features can also contribute to the understanding of attributes. In addition, aligning attribute features with class-level semantics ignores potential intra-class attribute variation. To mitigate these disadvantages, we present Attribute Localization and Revision Network in this paper. First, we design Attribute Localization Module (ALM) to capture both local and global features from image regions, a novel module called Scale Control Unit is incorporated to fuse global and local representations. Second, we propose Attribute Revision Module (ARM), which generates image-level semantics by revising the ground-truth value of each attribute, compensating for performance degradation caused by ignoring intra-class variation. Finally, the output of ALM will be aligned with revised semantics produced by ARM to achieve the training process. Comprehensive experimental results on three widely used benchmarks demonstrate the effectiveness of our model in the zero-shot prediction task.
更多查看译文
关键词
revision network,learning,localization,zero-shot
AI 理解论文
溯源树
样例
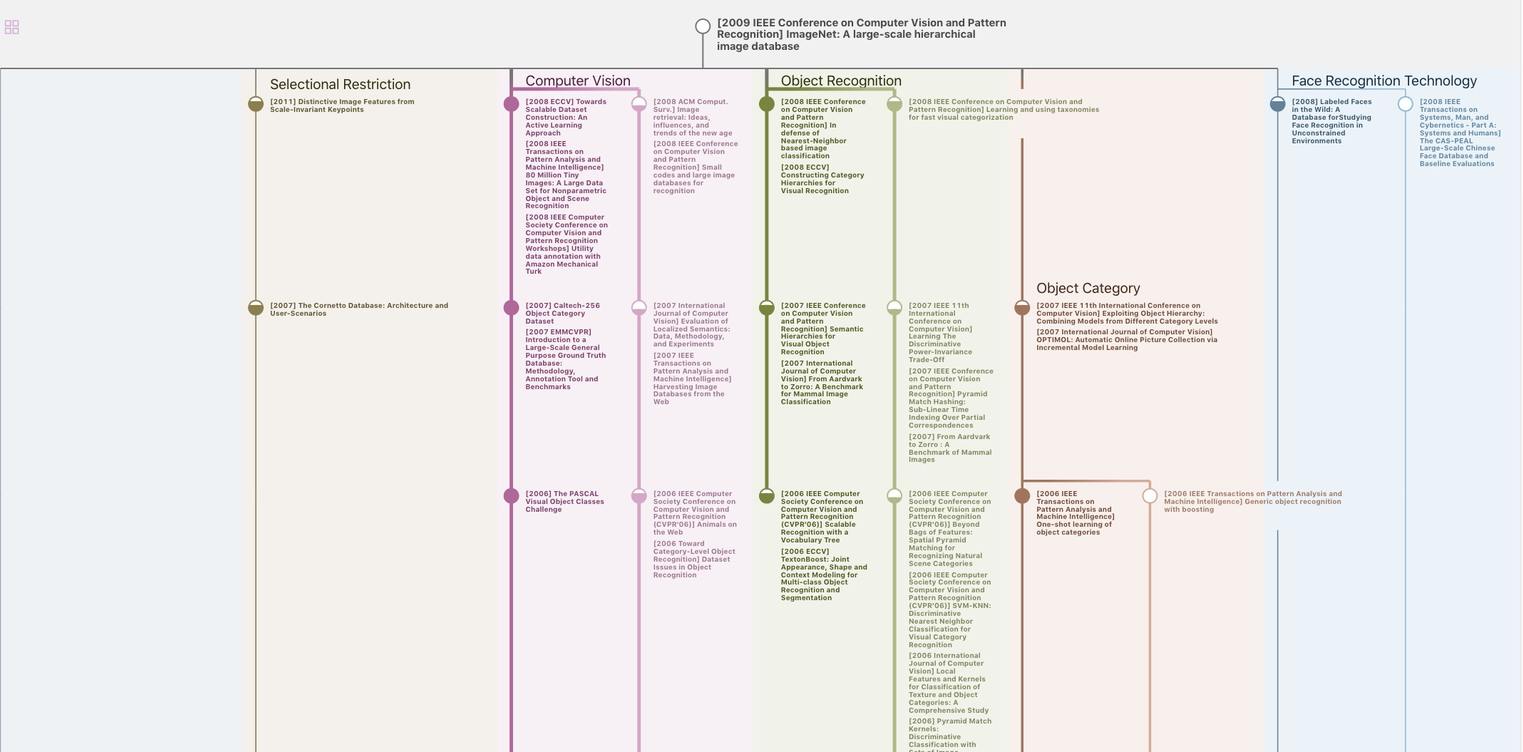
生成溯源树,研究论文发展脉络
Chat Paper
正在生成论文摘要