Orthogonal Random Features: Explicit Forms and Sharp Inequalities
CoRR(2023)
摘要
Random features have been introduced to scale up kernel methods via randomization techniques. In particular, random Fourier features and orthogonal random features were used to approximate the popular Gaussian kernel. The former is performed by a random Gaussian matrix and leads exactly to the Gaussian kernel after averaging. In this work, we analyze the bias and the variance of the kernel approximation based on orthogonal random features which makes use of Haar orthogonal matrices. We provide explicit expressions for these quantities using normalized Bessel functions and derive sharp exponential bounds supporting the view that orthogonal random features are more informative than random Fourier features.
更多查看译文
关键词
orthogonal random features,explicit forms
AI 理解论文
溯源树
样例
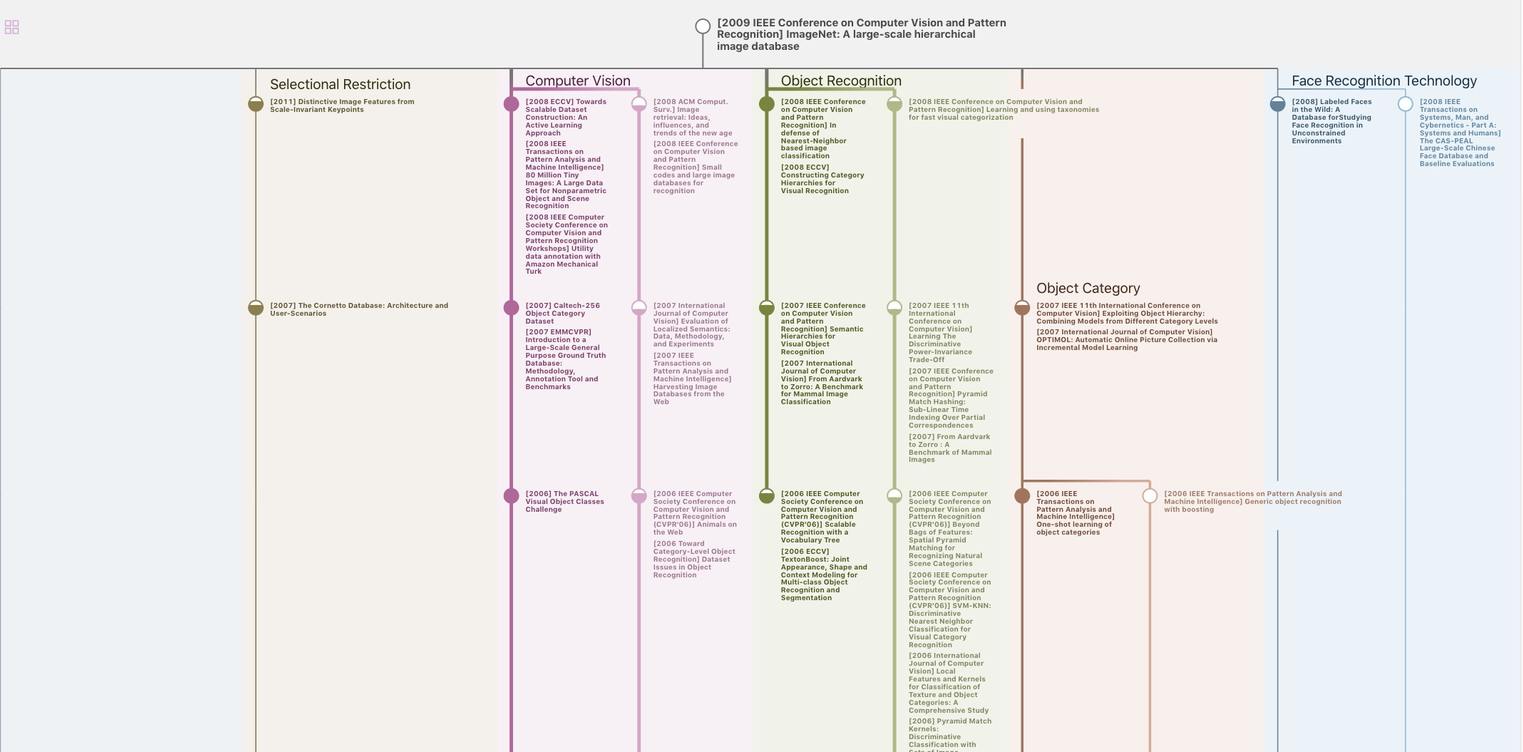
生成溯源树,研究论文发展脉络
Chat Paper
正在生成论文摘要