Multi-Agent Deep Reinforcement Learning for Energy-Efficient UAV-Aided Hybrid NOMA Data Collection
IEEE COMMUNICATIONS LETTERS(2023)
摘要
Non-orthogonal multiple access (NOMA) is regarded as a promising solution to improve the energy efficiency and reduce the latency of the unmanned aerial vehicle (UAV)-aided networks. In this letter, we consider an energy-efficient multi-UAV incorporating hybrid NOMA data collection system. Explicitly, the optimization problem of joint trajectory design and power allocation is formulated for maximizing energy utilization of the system. The optimization problem is a mixed integer non-convex problem and involves continuous variables. To tackle this challenging problem, we utilize a multi-agent deep reinforcement learning (MADRL) approach, i.e., multi-agent Twin Delayed Deep Deterministic Policy Gradient (MATD3), which introduces clipped double Q-learning and deep networks to reduce overestimation bias. Furthermore, a reward shaping method is applied to speed up the learning efficiency and convergence. Corroborated by extensive experiments, the proposed hybrid NOMA enhanced multi-UAV outperforms pure NOMA and OMA cases.
更多查看译文
关键词
Multi-agent deep reinforcement learning,unmanned aerial vehicle,non-orthogonal multiple access,data collection
AI 理解论文
溯源树
样例
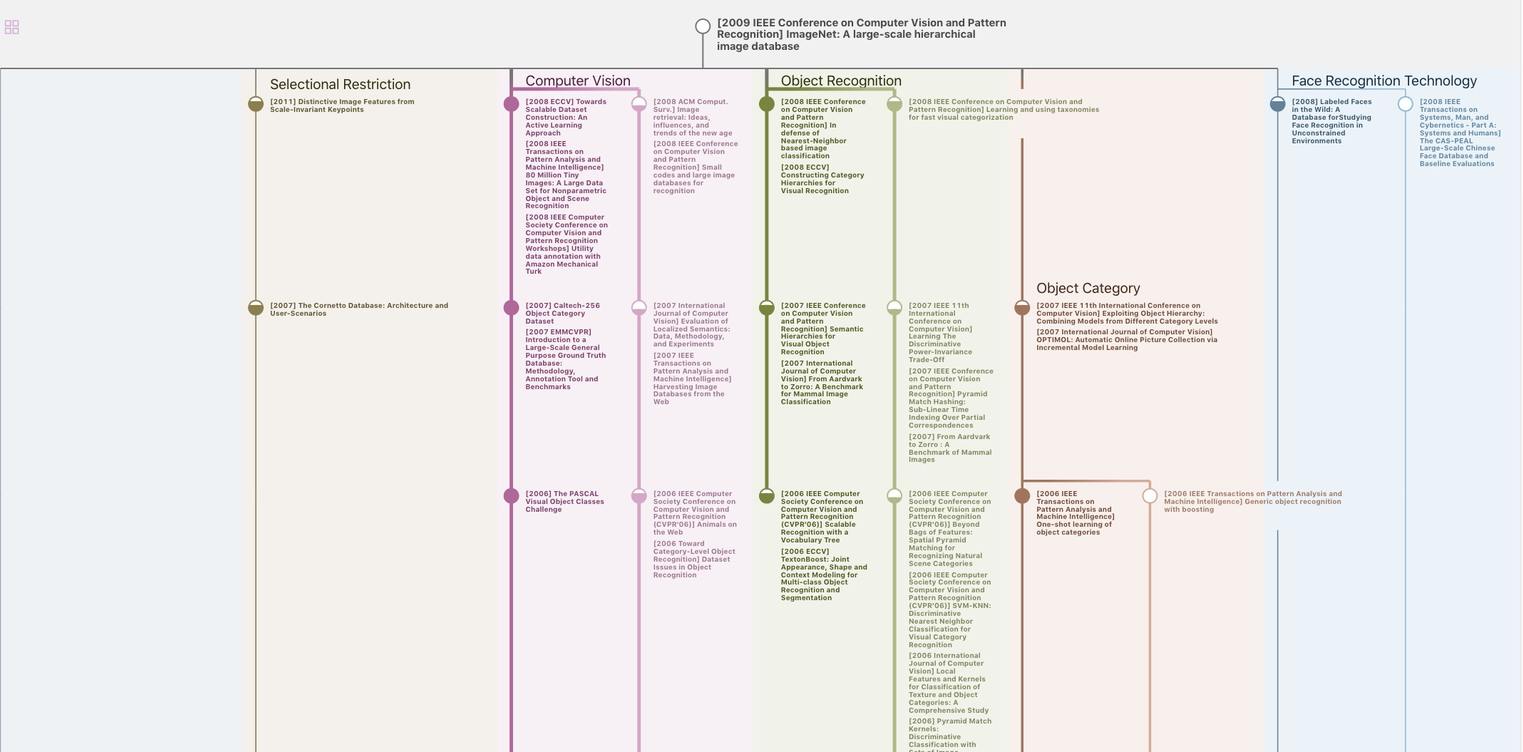
生成溯源树,研究论文发展脉络
Chat Paper
正在生成论文摘要