Geometrically Aligned Transfer Encoder for Inductive Transfer in Regression Tasks
ICLR 2024(2023)
摘要
Transfer learning is a crucial technique for handling a small amount of data that is potentially related to other abundant data. However, most of the existing methods are focused on classification tasks using images and language datasets. Therefore, in order to expand the transfer learning scheme to regression tasks, we propose a novel transfer technique based on differential geometry, namely the Geometrically Aligned Transfer Encoder (GATE). In this method, we interpret the latent vectors from the model to exist on a Riemannian curved manifold. We find a proper diffeomorphism between pairs of tasks to ensure that every arbitrary point maps to a locally flat coordinate in the overlapping region, allowing the transfer of knowledge from the source to the target data. This also serves as an effective regularizer for the model to behave in extrapolation regions. In this article, we demonstrate that GATE outperforms conventional methods and exhibits stable behavior in both the latent space and extrapolation regions for various molecular graph datasets.
更多查看译文
关键词
Transfer Learning,Inductive Transfer,Geometrical Deeplearning,Regression
AI 理解论文
溯源树
样例
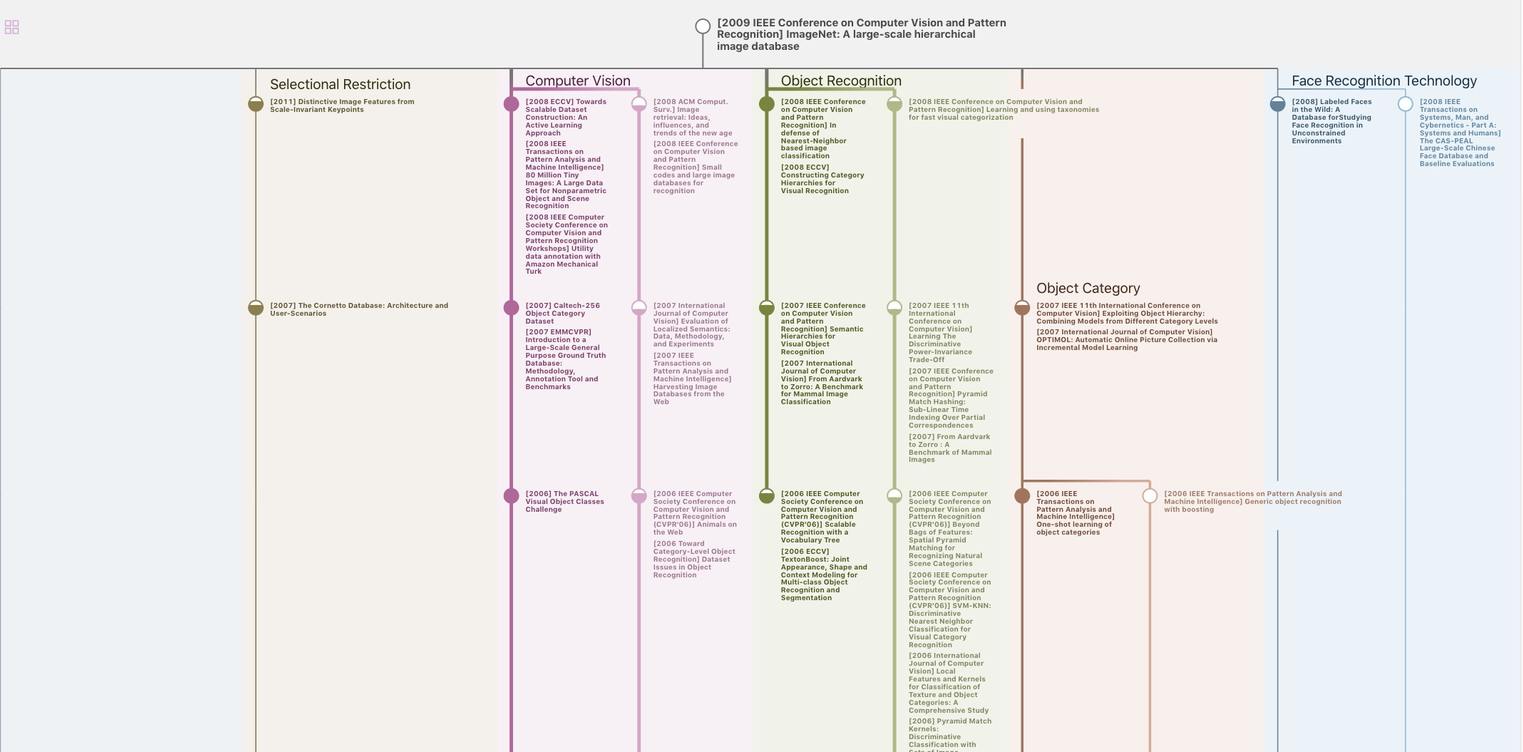
生成溯源树,研究论文发展脉络
Chat Paper
正在生成论文摘要