Fingerprint Attack: Client De-Anonymization in Federated Learning
ECAI 2023(2023)
摘要
Federated Learning allows collaborative training without data sharing in settings where participants do not trust the central server and one another. Privacy can be further improved by ensuring that communication between the participants and the server is anonymized through a shuffle; decoupling the participant identity from their data. This paper seeks to examine whether such a defense is adequate to guarantee anonymity, by proposing a novel fingerprinting attack over gradients sent by the participants to the server. We show that clustering of gradients can easily break the anonymization in an empirical study of learning federated language models on two language corpora. We then show that training with differential privacy can provide a practical defense against our fingerprint attack.
更多查看译文
关键词
de-anonymization
AI 理解论文
溯源树
样例
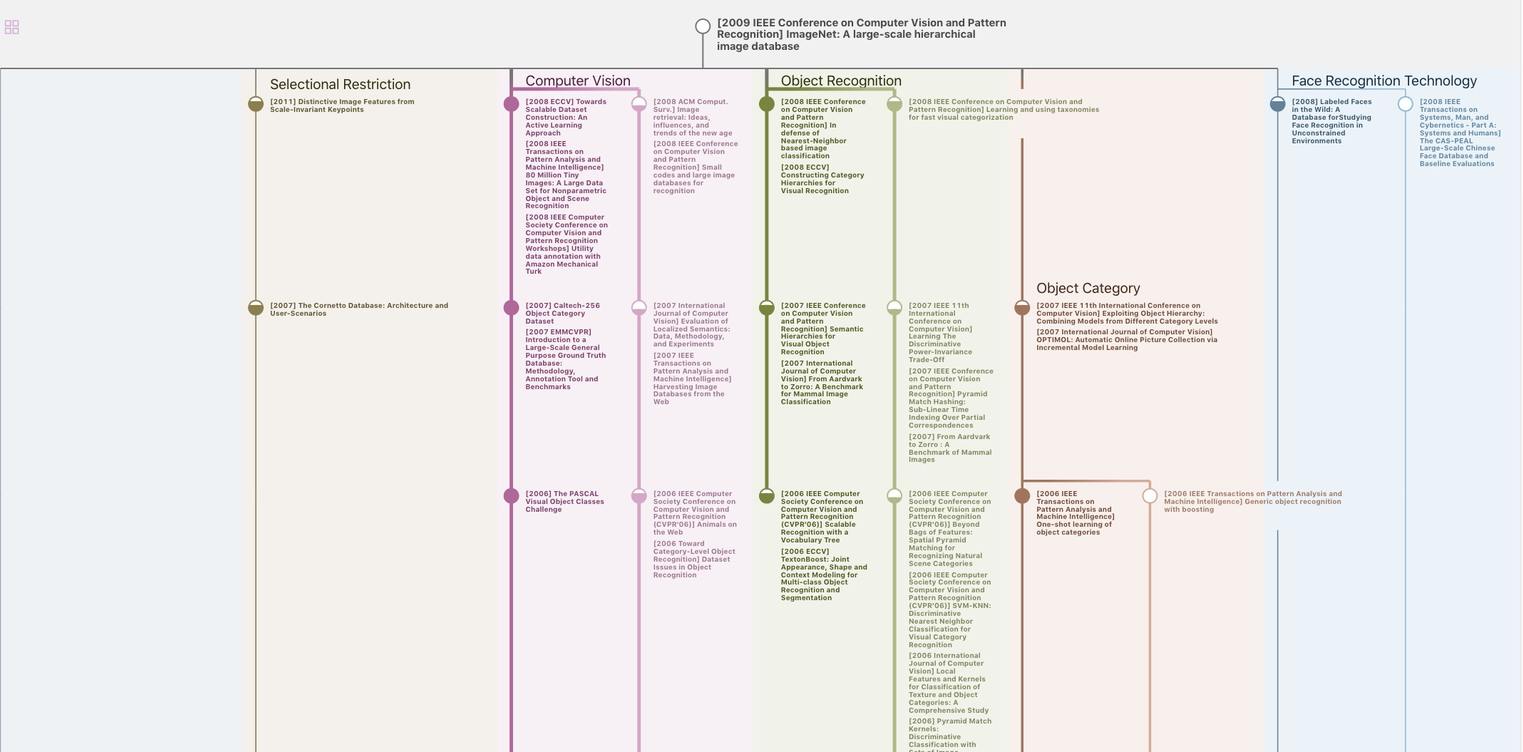
生成溯源树,研究论文发展脉络
Chat Paper
正在生成论文摘要