On Reducing User Interaction Data for Personalization
ACM Transactions on Recommender Systems(2023)
摘要
Most recommender systems rely on user interaction data for personalization. Usually, the recommendation quality improves with more data. In this work, we study the quality implications when limiting user interaction data for personalization purposes. We formalize this problem and provide algorithms for selecting a smaller subset of user interaction data. We propose a selection method that picks the subset of a user’s history items that maximizes the expected recommendation quality. We show on well-studied benchmarks that it is possible to achieve high-quality results with small subsets of less than 10 items per user.
更多查看译文
关键词
Item recommendation,data efficiency
AI 理解论文
溯源树
样例
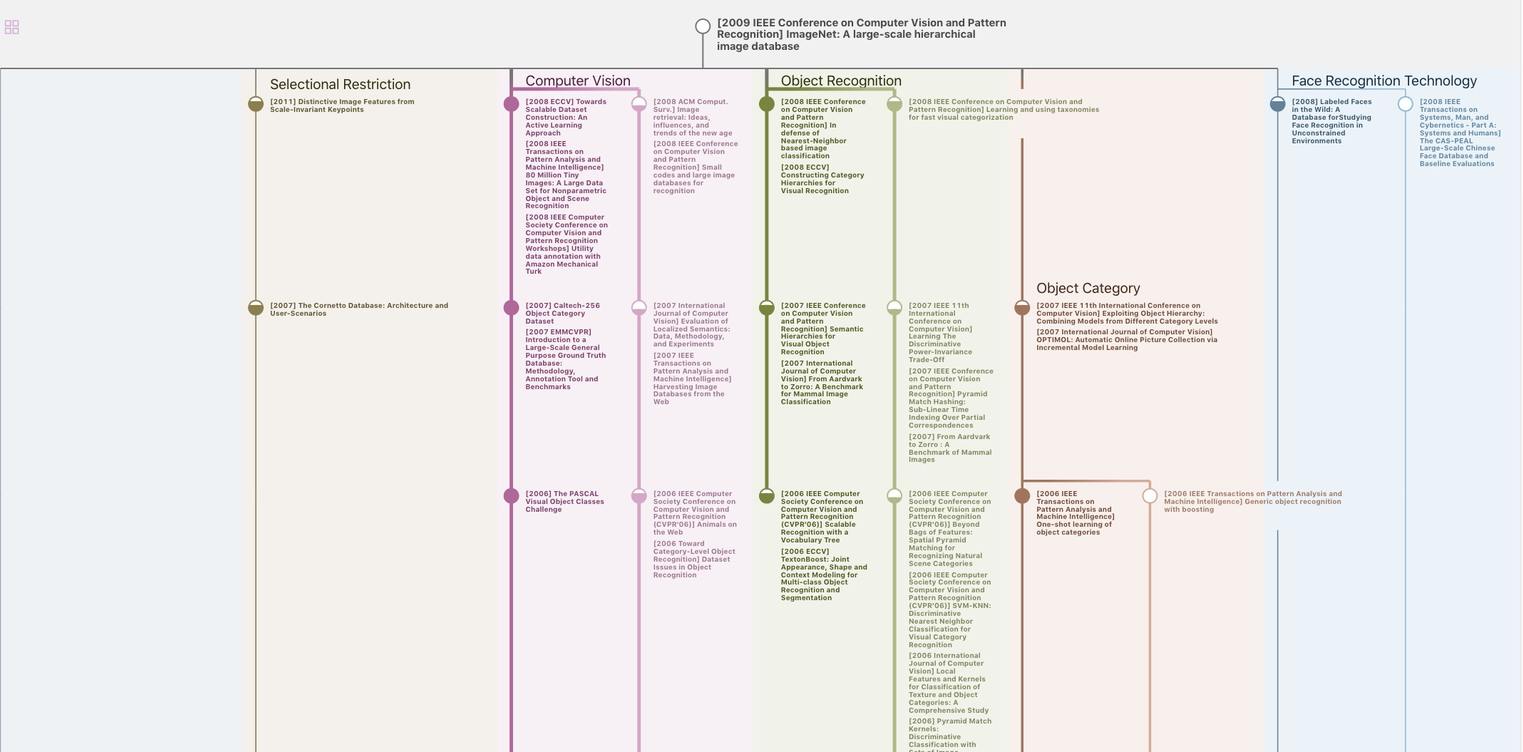
生成溯源树,研究论文发展脉络
Chat Paper
正在生成论文摘要