DomainNet: Homograph Detection and Understanding in Data Lake Disambiguation
ACM Transactions on Database Systems(2023)
摘要
Modern data lakes are heterogeneous in the vocabulary that is used to describe data. We study a problem of disambiguation in data lakes: How can we determine if a data value occurring more than once in the lake has different meanings and is therefore a homograph? While word and entity disambiguation have been well studied in computational linguistics, data management, and data science, we show that data lakes provide a new opportunity for disambiguation of data values, because tables implicitly define a massive network of interconnected values. We introduce DomainNet , which efficiently represents this network, and investigate to what extent it can be used to disambiguate values without requiring any supervision. DomainNet leverages network-centrality measures on a bipartite graph whose nodes represent data values and attributes to determine if a value is a homograph. A thorough experimental evaluation demonstrates that state-of-the-art techniques in domain discovery cannot be re-purposed to compete with our method. Specifically, using a domain discovery method to identify homographs achieves an F1-score of 0.38 versus 0.69 for DomainNet , which separates homographs well from data values that have a unique meaning. On a real data lake, our top-100 precision is 93%. Given a homograph, we also present a novel method for determining the number of meanings of the homograph and for assigning its data lake attributes to a meaning. We show the influence of homographs on two downstream tasks: entity-matching and domain discovery.
更多查看译文
关键词
homograph detection,data
AI 理解论文
溯源树
样例
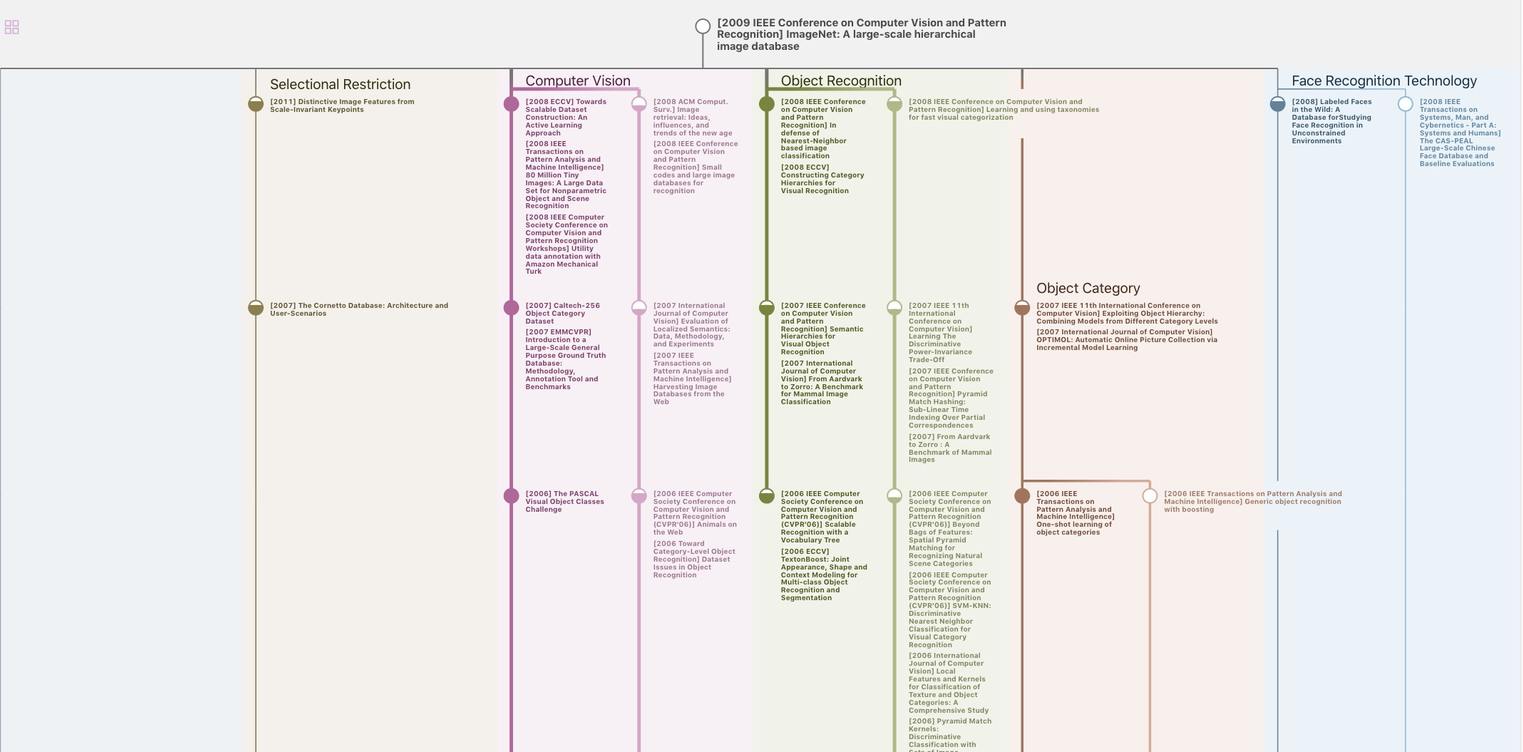
生成溯源树,研究论文发展脉络
Chat Paper
正在生成论文摘要