How Much Unlabeled Data is Really Needed for Effective Self-Supervised Human Activity Recognition?
ISWC '23: Proceedings of the 2023 International Symposium on Wearable Computers(2023)
摘要
The prospect of learning effective representations from unlabeled data alone has led to a boost in developing self-supervised learning (SSL) methods for sensor-based Human Activity Recognition (HAR). Typically, (large-scale) unlabeled data are used for pre-training, with the learned weights being used as feature extractors for recognizing activities. While prior works have focused on the impact of increased data scale on performance, instead, we aim to discover the pre-training data efficiency of self-supervised methods. We empirically determine the minimal quantities of unlabeled data required for obtaining comparable performance to using all available data. We investigate three established SSL methods for HAR on three target datasets. Out of these three methods, we discover that Contrastive Predictive Coding (CPC) is the most efficient in terms of pre-training data requirements: just 15 minutes of sensor data across participants is sufficient to obtain competitive activity recognition performance. Further, around 5 minutes of source data is enough when there are sufficient amounts of target application data available. These findings can serve as starting point for more efficient data collection practices.
更多查看译文
AI 理解论文
溯源树
样例
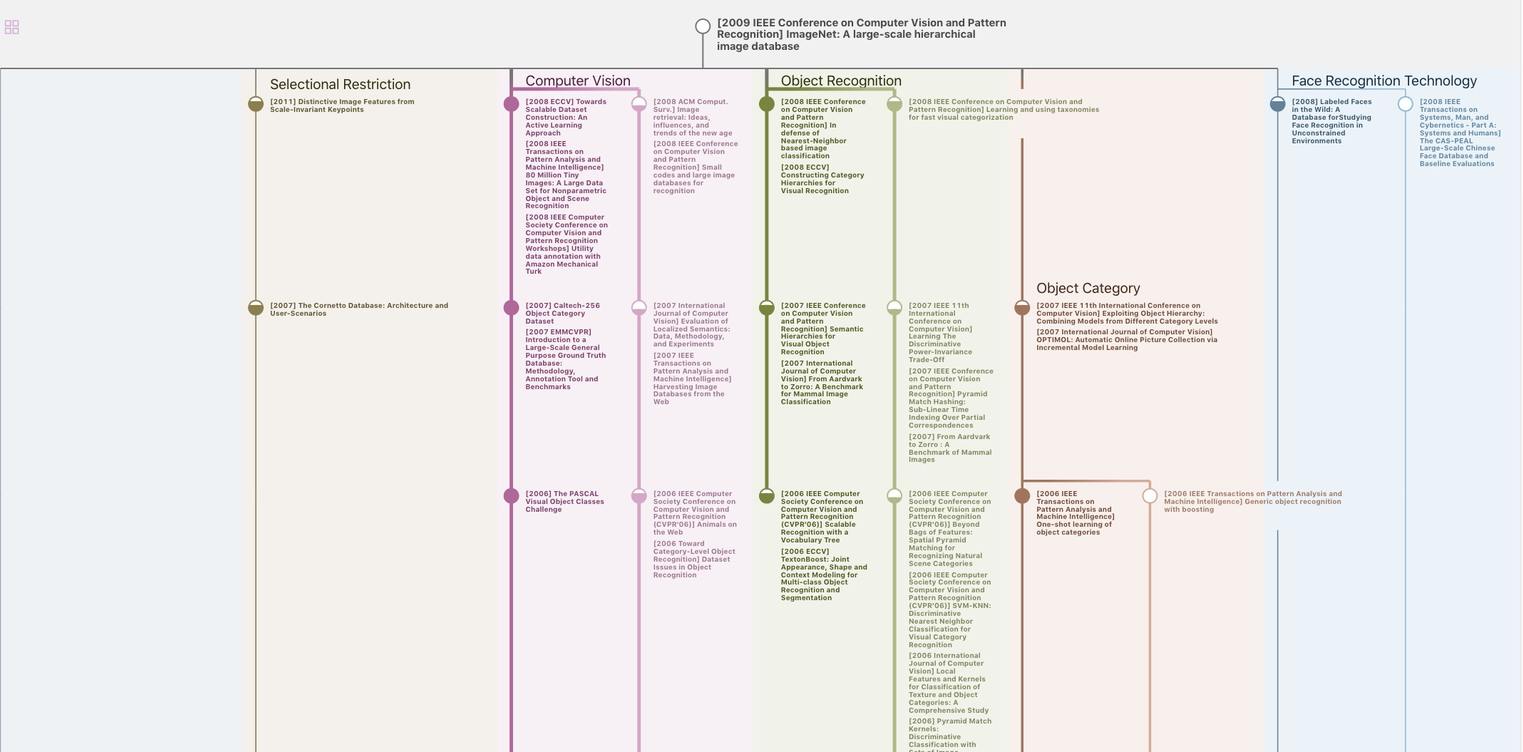
生成溯源树,研究论文发展脉络
Chat Paper
正在生成论文摘要