SmartEM: machine-learning guided electron microscopy
bioRxiv (Cold Spring Harbor Laboratory)(2023)
摘要
Connectomics provides essential nanometer-resolution, synapse-level maps of neural circuits to understand brain activity and behavior. However, few researchers have access to the high-throughput electron microscopes to rapidly generate the very large datasets needed for reconstructing whole circuits or brains. To date, machine-learning methods have been used after the collection of images by electron microscopy (EM) to accelerate and improve neuronal segmentation, synapse reconstruction and other data analysis. With the computational improvements in processing EM images, acquiring EM images has now become the rate-limiting step. Here, in order to speed up EM imaging, we integrate machine-learning into real-time image acquisition in a single-beam scanning electron microscope. This SmartEM approach allows an electron microscope to perform intelligent, data-aware imaging of specimens. SmartEM allocates the proper imaging time for each region of interest - scanning all pixels as rapidly, but then re-scanning small subareas more slowly where a higher quality signal is required in order to guarantee uniform segmentability of the entire field of view but with a significant time savings. We demonstrate that this pipeline achieves a 7-fold acceleration of image acquisition time for connectomics using a commercial single-beam SEM. We apply SmartEM to reconstruct a portion of mouse cortex with the same accuracy as traditional microscopy but in less time.
### Competing Interest Statement
P.P., M.P. and R.S. are employees of Thermo Fisher Scientific.
更多查看译文
关键词
electron microscopy,smartem,machine-learning
AI 理解论文
溯源树
样例
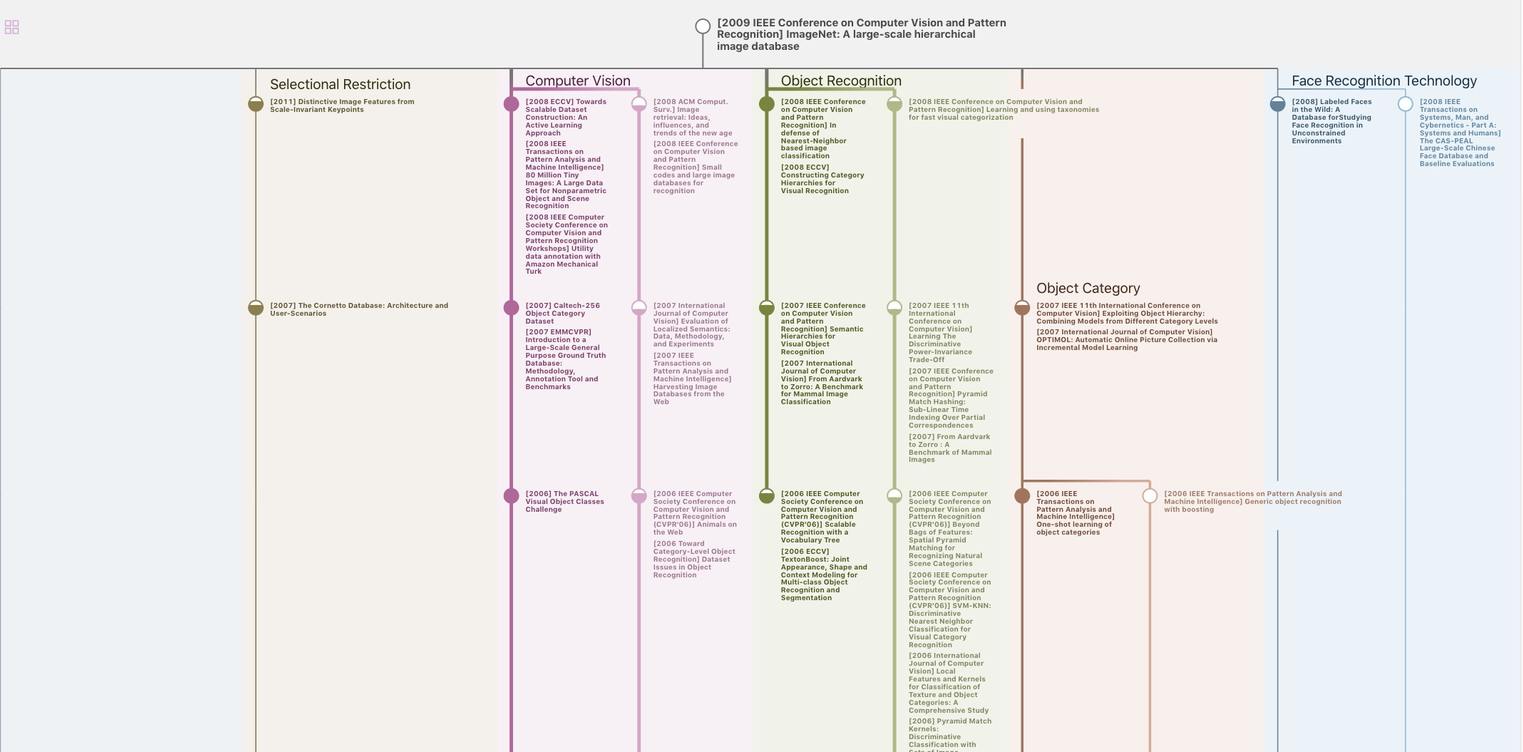
生成溯源树,研究论文发展脉络
Chat Paper
正在生成论文摘要