FairTune: Optimizing Parameter Efficient Fine Tuning for Fairness in Medical Image Analysis
ICLR 2024(2023)
摘要
Training models with robust group fairness properties is crucial in ethically
sensitive application areas such as medical diagnosis. Despite the growing body
of work aiming to minimise demographic bias in AI, this problem remains
challenging. A key reason for this challenge is the fairness generalisation
gap: High-capacity deep learning models can fit all training data nearly
perfectly, and thus also exhibit perfect fairness during training. In this
case, bias emerges only during testing when generalisation performance differs
across subgroups. This motivates us to take a bi-level optimisation perspective
on fair learning: Optimising the learning strategy based on validation
fairness. Specifically, we consider the highly effective workflow of adapting
pre-trained models to downstream medical imaging tasks using
parameter-efficient fine-tuning (PEFT) techniques. There is a trade-off between
updating more parameters, enabling a better fit to the task of interest vs.
fewer parameters, potentially reducing the generalisation gap. To manage this
tradeoff, we propose FairTune, a framework to optimise the choice of PEFT
parameters with respect to fairness. We demonstrate empirically that FairTune
leads to improved fairness on a range of medical imaging datasets. The code is
available at https://github.com/Raman1121/FairTune
更多查看译文
关键词
Fairness,PEFT,Hyperparameter Optimization,Medical Imaging
AI 理解论文
溯源树
样例
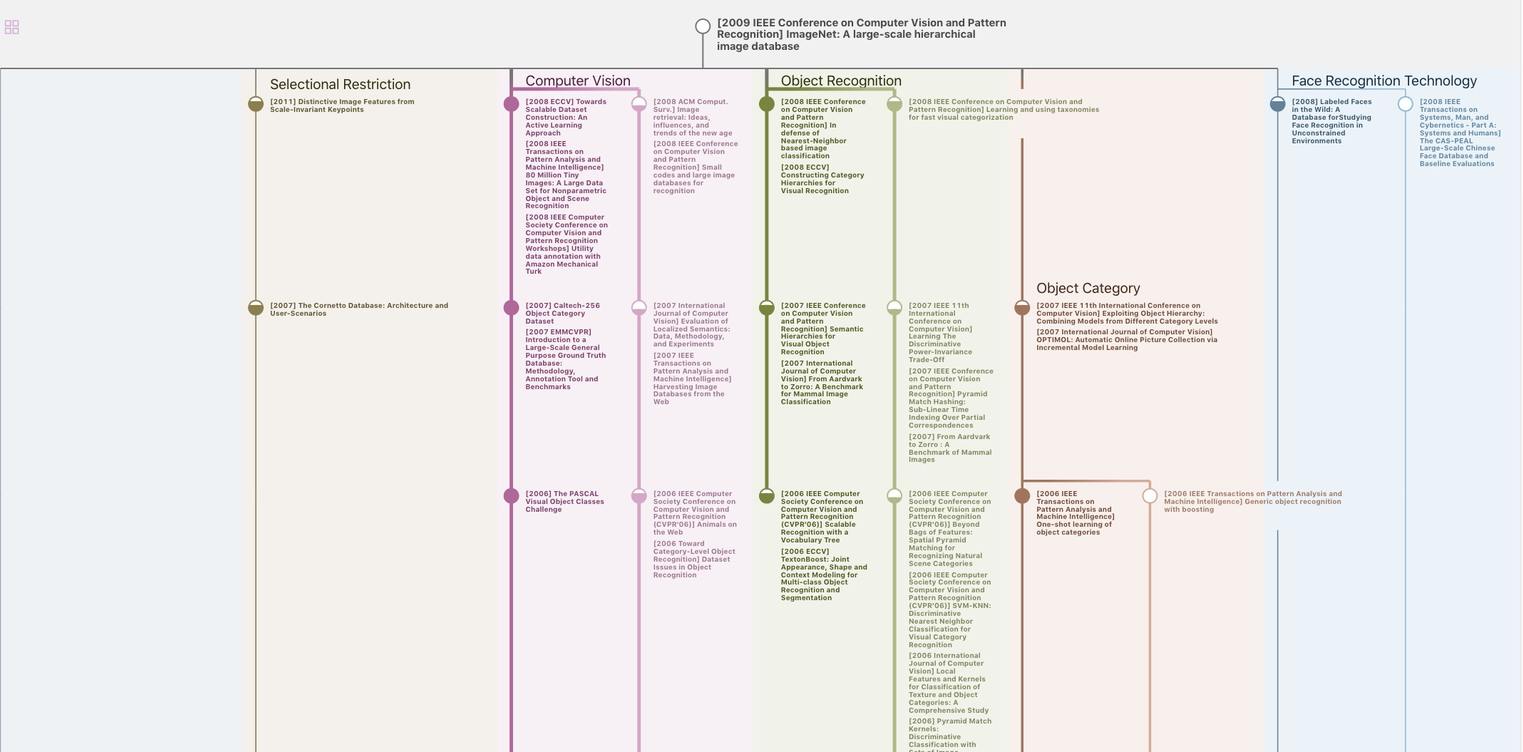
生成溯源树,研究论文发展脉络
Chat Paper
正在生成论文摘要