Attention-Based Fusion Factor to FPN for Small Object Detection
2023 8th International Conference on Image, Vision and Computing (ICIVC)(2023)
摘要
FPN-based detectors have made notable progress in general object detection, but the performance and efficiency of detecting small objects are far from satisfactory. Even through FPN can fuse semantic information from deep layers to shallow layers, it also brings noise containing spatial information of large objects to shallow layers. To address this challenge, we propose an attention-based fusion factor (AFF) consisting of two components,
$\boldsymbol{\alpha}-\mathbf{CBAM}$
and
$\boldsymbol{\alpha}-\mathbf{Query}$
, to enhance the semantic information of small objects in the fusion process. The
$\boldsymbol{\alpha}-\mathbf{CBAM}$
can be used to learn the vertical fusion factor of the FPN to control the information passed from deep to shallow layers. The
$\boldsymbol{\alpha}-\mathbf{Query}$
acts as an fusion factor to control the horizontal transfer impact of FPN.
$\boldsymbol{\alpha}-\mathbf{Query}$
first predicts the coarse locations of small objects in low-resolution feature maps and then increases their weights in high-resolution feature maps. Ablation studies on the publicly available dataset PASCAL VOC are conducted to demonstrate the effectiveness of
$\boldsymbol{\alpha}-\mathbf{CBAM}$
and
$\boldsymbol{\alpha}-\mathbf{Query}$
. Moreover, experimental results on the datasets MS COCO and VisDrone show that the baseline model with additional AFF evidently outperforms itself in almost all the related metrics.
更多查看译文
关键词
Attention-based fusion factor,FPN,fusion factor,$\boldsymbol{\alpha}-\mathbf{CBAM}$,$\boldsymbol{\alpha}-\mathbf{Query}$
AI 理解论文
溯源树
样例
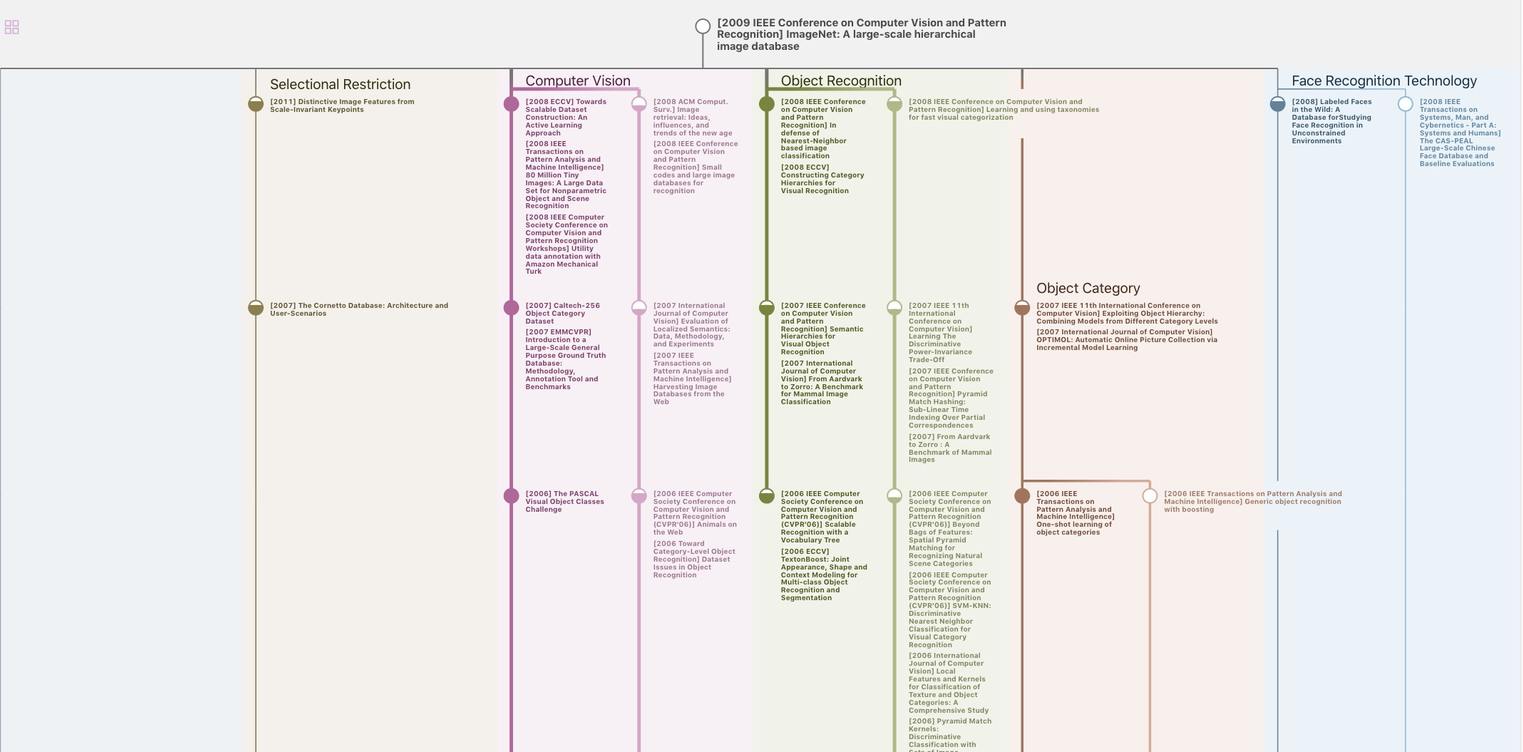
生成溯源树,研究论文发展脉络
Chat Paper
正在生成论文摘要