Guest Editorial: Spectral imaging powered computer vision
IET Computer Vision(2023)
摘要
The increasing accessibility and affordability of spectral imaging technology have revolutionised computer vision, allowing for data capture across various wavelengths beyond the visual spectrum. This advancement has greatly enhanced the capabilities of computers and AI systems in observing, understanding, and interacting with the world. Consequently, new datasets in various modalities, such as infrared, ultraviolet, fluorescent, multispectral, and hyperspectral, have been constructed, presenting fresh opportunities for computer vision research and applications. Although significant progress has been made in processing, learning, and utilising data obtained through spectral imaging technology, several challenges persist in the field of computer vision. These challenges include the presence of low-quality images, sparse input, high-dimensional data, expensive data labelling processes, and a lack of methods to effectively analyse and utilise data considering their unique properties. Many mid-level and high-level computer vision tasks, such as object segmentation, detection and recognition, image retrieval and classification, and video tracking and understanding, still have not leveraged the advantages offered by spectral information. Additionally, the problem of effectively and efficiently fusing data in different modalities to create robust vision systems remains unresolved. Therefore, there is a pressing need for novel computer vision methods and applications to advance this research area. This special issue aims to provide a venue for researchers to present innovative computer vision methods driven by the spectral imaging technology. This special issue has received 11 submissions. Among them, five papers have been accepted for publication, indicating their high quality and contribution to spectral imaging powered computer vision. Four papers have been rejected and sent to a transfer service for consideration in other journals or invited for re-submission after revision based on reviewers’ feedback. The accepted papers can be categorised into three main groups based on the type of adopted data, that is, hyperspectral, multispectral, and X-ray images. Hyperspectral images provide material information about the scene and enable fine-grained object class classification. Multispectral images provide high spatial context and information beyond visible spectrum, such as infrared, providing enriched clues for visual computation. X-ray images can penetrate the surface of objects and provide internal structural information of targets, empowering medical applications, such as rib detection as exemplified by Tsai et al. Below is a brief summary of each paper in this special issue. Zhong et al. proposed a lightweight criss-cross large kernel (CCLK) convolutional neural network for hyperspectral classification. The key component of this network is a CCLK module, which incorporates large kernels within the 1D convolutional layers and computes self-attention in orthogonal directions. Due to the large kernels and multiple stacks of the CCLK modules, the network can effectively capture long-range contextual features with a compact model size. The experimental results show that the network achieves enhanced classification performance and generalisation capability compared to alternative lightweight deep learning methods. Fewer parameters also make it suitable for deployment on devices with limited resources. Ye et al. developed a domain-invariant attention network to address heterogeneous transfer learning in cross-scene hyperspectral classification. The network includes a feature-alignment convolutional neural networks (FACNN) and domain-invariant attention block (DIAB). FACNN extracts features from source and target scenes and projects the heterogeneous features from two scenes into a shared low-dimensional subspace, guaranteeing the class consistency between scenes. DIAB gains cross-domain consistency with a specially designed class-specific domain-invariance loss to obtain domain-invariant and discriminative attention weights for samples, reducing the domain shift. In this way, the knowledge of source scene is successfully transferred to the target scene, alleviating the small training samples in hyperspectral classification. The experiments prove that the network achieves promising hyperspectral classification. Zuo et al. developed a method for multispectral pedestrian detection, focusing on scale-aware permutation attention and adjacent feature aggregation. The scale-aware permutated attention module uses both local and global attention to enhance pedestrian features of different scales in the feature pyramid, improving the quality of feature fusion. The adjacent-branch feature aggregation module considers both semantic context and spatial resolution, leading to improved detection accuracy for small-sized pedestrians. Extensive experimental evaluations showcase notable improvements in both efficiency and accuracy compared to several existing methods. Guo et al. introduced a model called spatial-temporal-meteorological/long short-term memory network (STM-LSTM) to predict photovoltaic power generation. The proposed method integrates satellite image, historical meteorological data and historical power generation data, and uses cloud motion-aware learning to account for cloud movement and an attention mechanism to weigh the images in different bands from satellite cloud maps. The LSTM model combines the historical power generation sequence and meteorological change information for better accuracy. Experimental results show that the STM-LSTM model outperforms the baseline model to a certain margin, indicating its effectiveness in photovoltaic power generation prediction. Tsai et al. created a fully annotated EDARib-CXR dataset for the identification and localization of fractured ribs in frontal and oblique chest X-ray images. The dataset consists of 369 frontal and 829 oblique chest X rays, providing valuable resources for research in this field. Based on YOLOv5, two detection models, namely AB-YOLOv5 and PB-YOLOv5, were introduced. AB-YOLOv5 incorporates an auxiliary branch that enhances the resolution of extracted feature maps in the final convolutional network layer, facilitating the determination of fracture location when relevant characteristics are identified in the data. On the other hand, PB-YOLOv5 employs image patches instead of the entire image to preserve the features of small objects in downsampled images during training, enabling the detection of subtle lesion features. Moreover, the researchers implemented a two-stage cascade detector that effectively integrates these two models to further improve the detection performance. Experimental results demonstrated superior performance of the introduced methods, providing an applicability in reducing diagnostic time and alleviating the heavy workload faced by clinicians. Spectral imaging powered computer vision is still an emerging research area with great potential of creating new knowledge and methods. All of the accepted papers in this special issue highlight the crucial need for techniques that leverage information beyond the visual spectrum to help understand the world through spectral imaging devices. The rapid advancements in spectral imaging technology have paved the way for new opportunities and tasks in computer vision research and applications. We expect that more researchers will join this exciting area and develop solutions to handle tasks that cannot be solved well by traditional computer vision. Jun Zhou and Fengchao Xiong led the organization of this special issue, including compiling the potential author’s list, calling for papers, handling paper reviews, and drafting the editorial. Lei Tong, Naoto Yokoya and Pedram Ghamisi provided valuable input on the scope of this special issue, promoted this special issue to potential authors, and gave feedback to the editorial. The authors of this editorial would like to express our gratitude to all the special issue authors for their valuable contributions and significant scientific results presented in their articles. Their innovative research has added depth and breadth to the field of computer vision, particularly in the context of spectral imaging technology. The authors would also like to acknowledge the anonymous reviewers for their professional expertise and constructive comments, which undoubtedly ensured the quality and value of this special issue. Additionally, the authors extend their appreciation to the editorial team of IET Computer Vision for their continuous support and guidance throughout the entire process. The authors hope that readers will find this collection of papers informative, inspiring, and thought-provoking. It is our sincere desire that this special issue will serve as a catalyst for further research and development in the field of spectral imaging technology within the realm of computer vision. The authors thank the National Natural Science Foundation of China (Grant No. 62002169) for supporting Fengchao Xiong's contribution to this special issue. Jun Zhou received the B.S. degree in computer science from Nanjing University of Science and Technology, Nanjing, China, the M.S. degree in computer science from Concordia University, Montreal, Canada, and the Ph.D. degree from the University of Alberta, Edmonton, Canada. In June 2012, he joined the School of Information and Communication Technology, Griffith University, Nathan, Australia, where he is currently a professor. Prior to Griffith University, he was a research fellow with the Research School of Computer Science, Australian National University, Canberra, Australia, and a researcher with the Canberra Research Laboratory, NICTA, Canberra. His research interests include pattern recognition, computer vision, and spectral imaging with their applications in remote sensing and environmental informatics. He is an Associate Editor of IET Computer Vision, IEEE Transactions on Geoscience and Remote Sensing and Pattern Recognition journal. Fengchao Xiong received the B.E. degree in software engineering from Shandong University, Jinan, China, in 2014, and the Ph.D. degree from the College of Computer Science, Zhejiang University, Hangzhou, China, in 2019. He visited Wuhan University from 2011 to 2012 and Griffith University from 2017 to 2018. He is currently an associate professor with the School of Computer Science and Engineering, Nanjing University of Science and Technology, Nanjing, China. His research interests include hyperspectral image processing, machine learning, and pattern recognition. Besides this appointment, he is also a Post-Doctoral Fellow with the State Key Laboratory of Internet of Things for Smart City, Department of Computer and Information Science, University of Macau, Macau, China. He currently serves as a Topical Associate Editor for the IEEE Transactions on Geoscience and Remote Sensing. Lei Tong received the B.E. degree in measurement and control technology and instrumentation and the M.E. degree in measurement technology and automation devices from Beijing Jiaotong University, Beijing, China, in 2010 and 2012, respectively. He received the Ph.D. degree in Engineering from Griffith University, Brisbane, Australia, in 2016. Currently, he is an associate professor with Faculty of Information Technology, Beijing University of Technology, Beijing, China. His current research interests include signal and image processing, pattern recognition, and remote sensing. Naoto Yokoya received the M.Eng. and Ph.D. degrees from the Department of Aeronautics and Astronautics, The University of Tokyo, Tokyo, Japan, in 2010 and 2013, respectively. He currently holds the position of Associate Professor at The University of Tokyo and serves as the Team Leader of the Geoinformatics Team at the RIKEN Center for Advanced Intelligence Project. His research focuses on image processing, data fusion, and machine learning for understanding remote sensing images with applications to disaster management and environmental monitoring. He is an associate editor of IEEE Transactions on Geoscience and Remote Sensing. For further information, please visit https://naotoyokoya.com/. Pedram Ghamisi received the B.Sc. degree in civil (survey) engineering from the Tehran South Campus of Azad University, Tehran, Iran, in 2008, the M.Sc. degree (Hons.) in remote sensing from the K. N. Toosi University of Technology, Tehran, in 2012, and the Ph.D. degree in electrical and computer engineering from the University of Iceland, University of Iceland, Reykjavík, Iceland, in 2015. He currently holds the position of (1) Head of the Machine Learning Group at a Helmholtz institute in Germany and (2) Research Professor and Senior PI (leading AI4RS) at the Institute of Advanced Research in Artificial Intelligence (IARAI) in Austria. He also holds a visiting professorship at Lancaster University in England, UK. His research has earned him several honours and awards and has also been acknowledged multiple times in the Stanford University list of the top 2% of scientists and academics, as well as in the list of the top 1% most cited researchers published by Clarivate. His research interests encompass interdisciplinary investigations in deep learning, with a sharp focus on remote sensing and health applications. He serves as an Associate Editor for the IEEE Geoscience and Remote Sensing Letters and the Remote Sensing journal. For more detailed information, please visit https://www.ai4rs.com/.
更多查看译文
关键词
computer vision,imaging,spectral
AI 理解论文
溯源树
样例
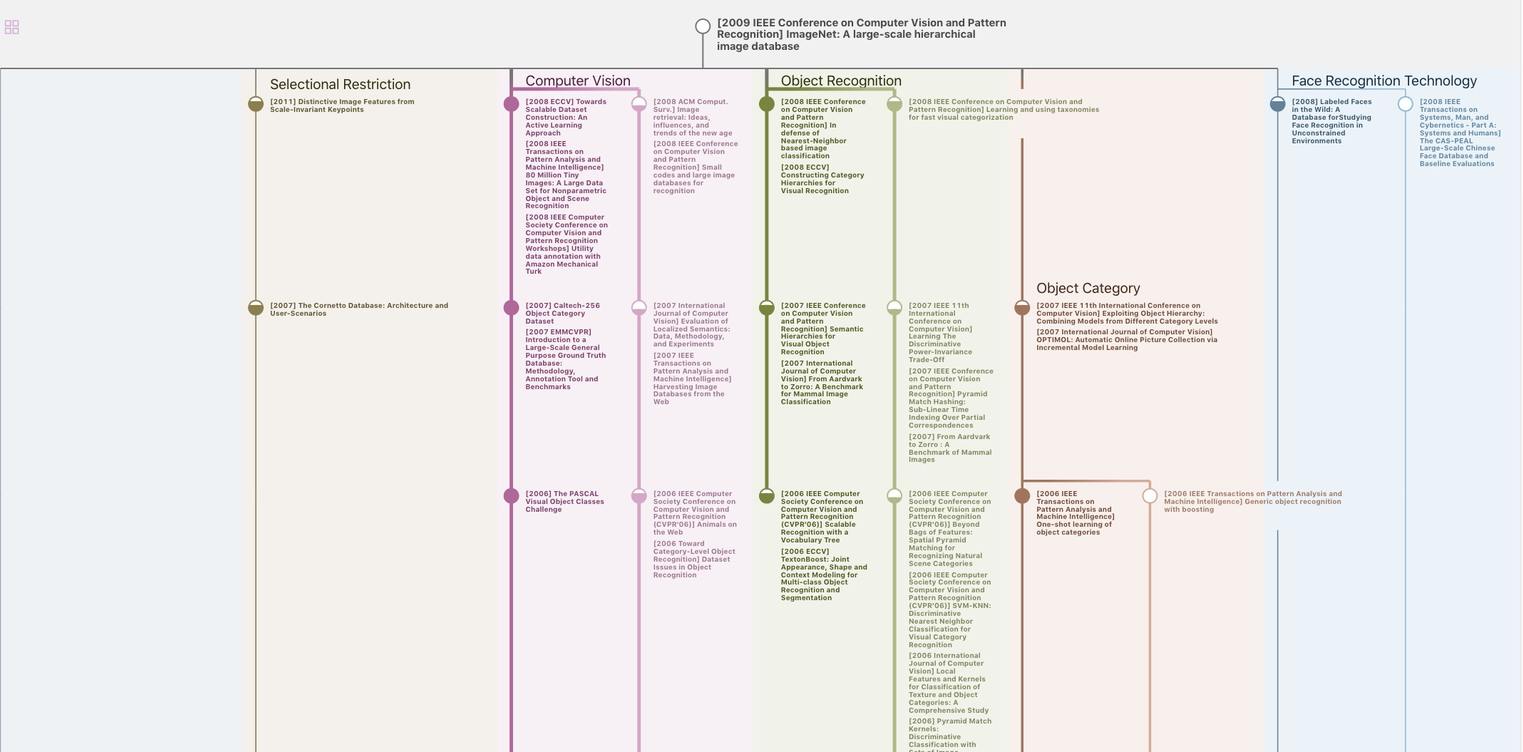
生成溯源树,研究论文发展脉络
Chat Paper
正在生成论文摘要