Anomaly Root Cause Analysis forWind Turbines Based on Denoising Autoencoder and Sparse Estimation
2023 IEEE 12TH DATA DRIVEN CONTROL AND LEARNING SYSTEMS CONFERENCE, DDCLS(2023)
摘要
Wind turbine condition monitoring has been extensively studied to reduce maintenance costs. Although there exist a vast amount of literature on anomaly detection for wind turbine, anomaly root cause analysis has not been fully addressed so far. To cope with this problem, we propose a denoising autoencoder (DAE) based anomaly detector and performs anomaly root cause analysis using sparse estimation. For anomaly detection, deep denoising autoencoder is learned with normal history data, with enhanced robustness compared to the conventional autoencoder. The reconstruction error from the DAE is further evaluated by the exponentially weighted moving average control chart (EWMA) to reduce the false positive rate. After anomaly detection, root cause analysis performs sparse fault estimation, with the assumption that a small number of observed variables are affected under the abnormal condition. The fault estimates are then leveraged to identify the variables most relevant to the underlying anomaly root causes. Real cases on a public dataset demonstrate the effectiveness of the proposed method.
更多查看译文
关键词
anomaly detection,root cause analysis,denoising autoencoder,sparse fault estimation,wind turbine
AI 理解论文
溯源树
样例
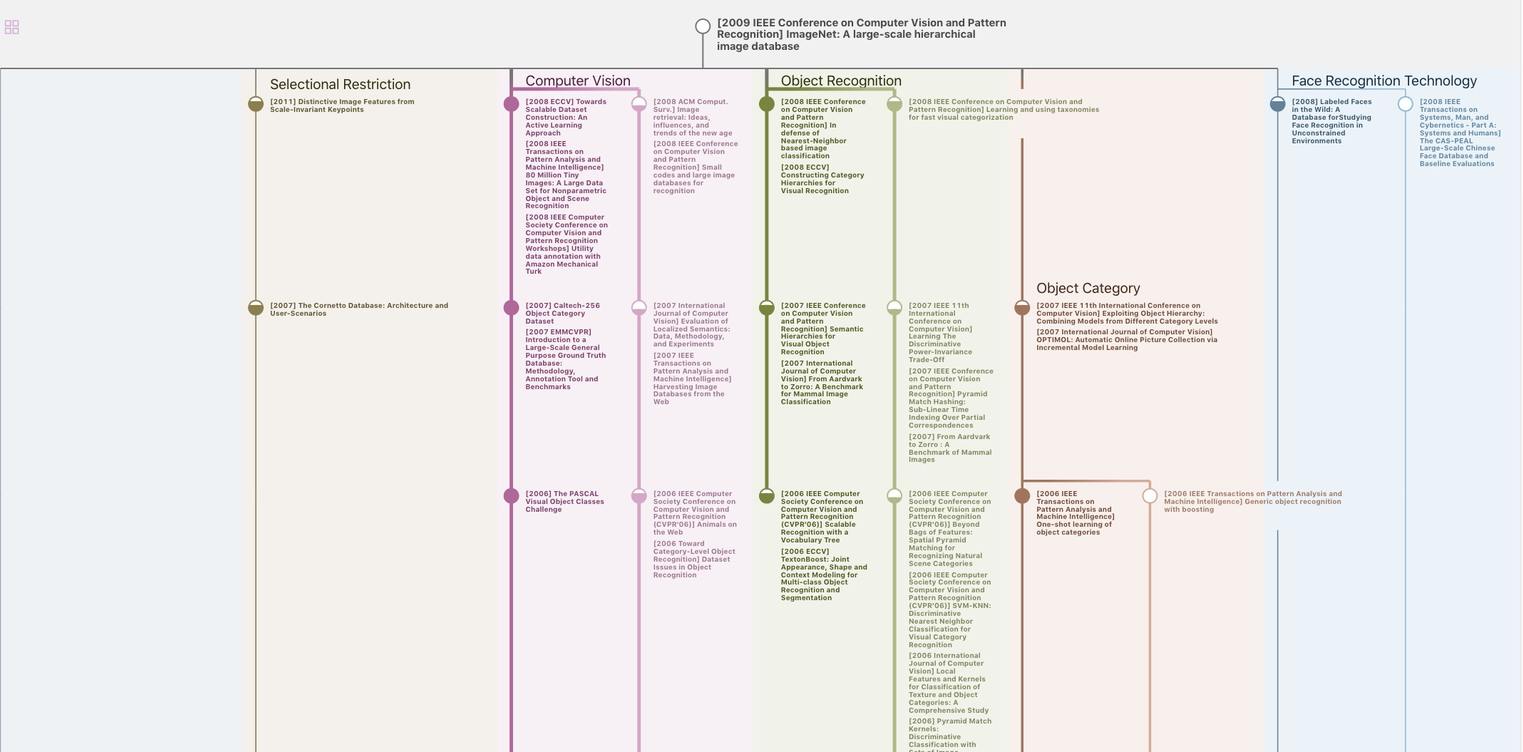
生成溯源树,研究论文发展脉络
Chat Paper
正在生成论文摘要