A LSTM Model with Attention Mechanism for Soft Sensor of SO2 Conversion Rate in Flue Gas Acid Production Process
2023 IEEE 12TH DATA DRIVEN CONTROL AND LEARNING SYSTEMS CONFERENCE, DDCLS(2023)
摘要
The sulfur dioxide (SO2) concentration of flue gas in metal smelting industry is high and difficult to recycle. The commonly used SO2 desulfurization treatment is acid production from flue gas, however, it is difficult to directly measure the conversion rate of SO2. In this paper, we present a soft sensor method based on Long Short-Term Memory (LSTM) which integrates the attention mechanism for predicting SO2 conversion rate to tackle such problem. Considering that the change of SO2 conversion rate is affected by many external factors, the attention mechanism is used to quickly and accurately predict the change results by considering the system data of several past moments. The proposed attention mechanism uses LSTM units to encode the hidden state of the look back time data, obtains different attention weights, and then decodes and predicts SO2 conversion rate according to the hidden state. The experimental results indicate that LSTM model with attention mechanism has lower training cost compared with LSTM model. The training accuracy and soft sensor accuracy are also improved owing to the attention mechanism. It is instructive for SO2 conversion rate soft sensor in acid production from flue gas.
更多查看译文
关键词
Acid production from flue gas,soft sensor,Long Short-Term Memory neural network,attention mechanism
AI 理解论文
溯源树
样例
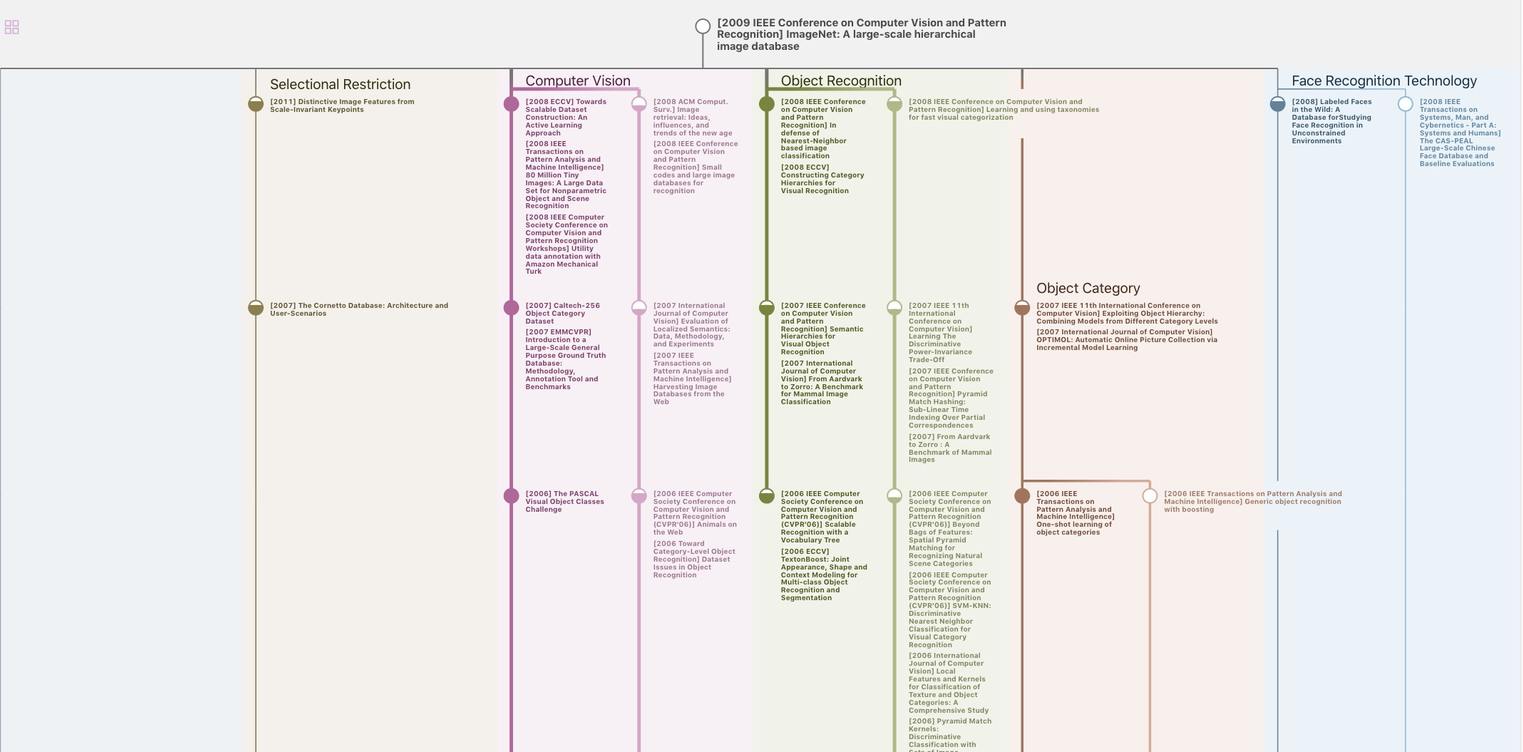
生成溯源树,研究论文发展脉络
Chat Paper
正在生成论文摘要