Knowledge-Associated Embedding for Memory-Aware Knowledge Tracing
IEEE TRANSACTIONS ON COMPUTATIONAL SOCIAL SYSTEMS(2023)
摘要
Knowledge tracing (KT) refers to predicting learners' performance in the future according to their historical learning interactions, which has become an essential task for the computer-aided education (CAE) system. Recent studies alleviate the data sparsity problem by mining higher-order information between questions and skills. However, the effect of multiple skills in the question is not distinguished, and various learning behaviors need to be better modeled. In this article, we propose a knowledge-associated embedding for the memory-aware KT (KMKT) framework. Specifically, we first construct a question-skill bipartite graph with attribute features. A knowledge-associated embedding (KAE) module is proposed to capture the distinctiveness of multiskills via the process of knowledge propagation and knowledge aggregation based on predefined knowledge-paths. Then, to simulate the memory recall phenomenon of the learners in KT, we design a memory-aware module for long short-term memory (MA-LSTM) networks. A temporal attention layer in MA-LSTM is proposed to learn the forgetting mechanism of the human brain. Finally, we introduce a learning-gain (LG) layer to obtain learners' benefits after each exercise. Extensive experiments on four real-world datasets illustrate that our KMKT model performs better than the other baseline models, which verifies the effectiveness of our work.
更多查看译文
关键词
Computer-aided education (CAE) system,knowledge tracing (KT),learner modeling,memory networks
AI 理解论文
溯源树
样例
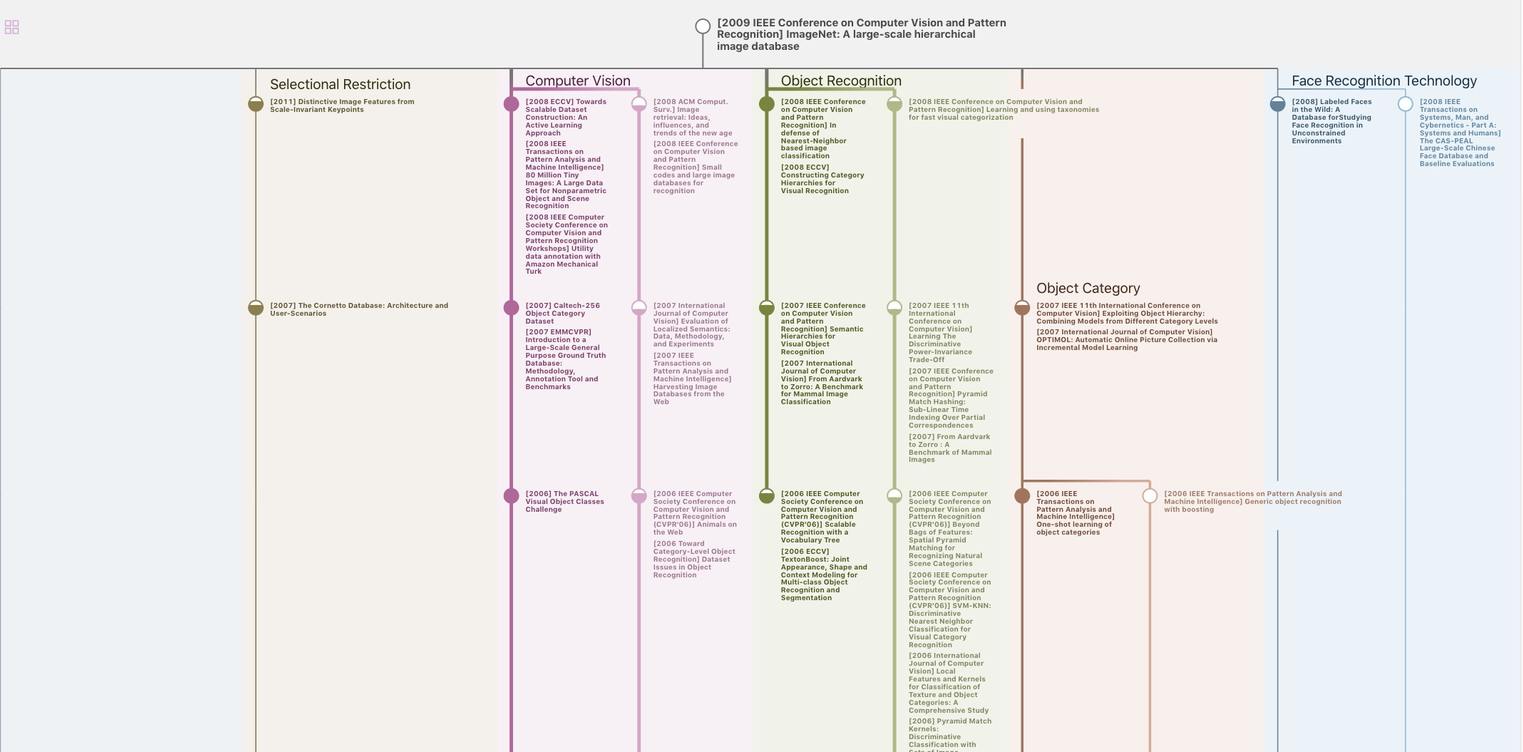
生成溯源树,研究论文发展脉络
Chat Paper
正在生成论文摘要