HFENet: Hybrid Feature Enhancement Network for Detecting Texts in Scenes and Traffic Panels
IEEE TRANSACTIONS ON INTELLIGENT TRANSPORTATION SYSTEMS(2023)
摘要
Text detection in complex scene images is a challenging task for intelligent transportation. Existing scene text detection methods often adopt multi-scale feature learning strategies to extract informative feature representations for covering objects of various sizes. However, the sampling operation inherent in multi-scale feature generation can easily impair high-frequency details (e.g., textures and boundaries), which are critical for text detection. In this work, we propose an innovative Hybrid Feature Enhancement Network (dubbed HFENet) to explicitly improve the quality of high-frequency information for detecting texts in scenes and traffic panels. To be concrete, we propose a simple yet effective self-guided feature enhancement module (SFEM) for globally lifting feature representations to highly discriminative and high-frequency abundant ones. Notably, our SFEM is pluggable and will be removed after training without introducing extra computational costs. In addition, due to the challenge and importance of accurately predicting boundaries for text detection, we propose a novel boundary enhancement module (BEM) to explicitly strengthen local feature representations in the guidance of boundary annotation for accurate localization. Extensive experiments on multiple publicly available datasets (i.e., MSRA-TD500, CTW1500, Total-Text, Traffic Guide Panel Dataset, Chinese Road Plate Dataset, and ASAYAR_TXT) verify the state-of-the-art performance of our method.
更多查看译文
关键词
Scene text detection,arbitrary-shaped text,feature enhancement,boundary enhancement
AI 理解论文
溯源树
样例
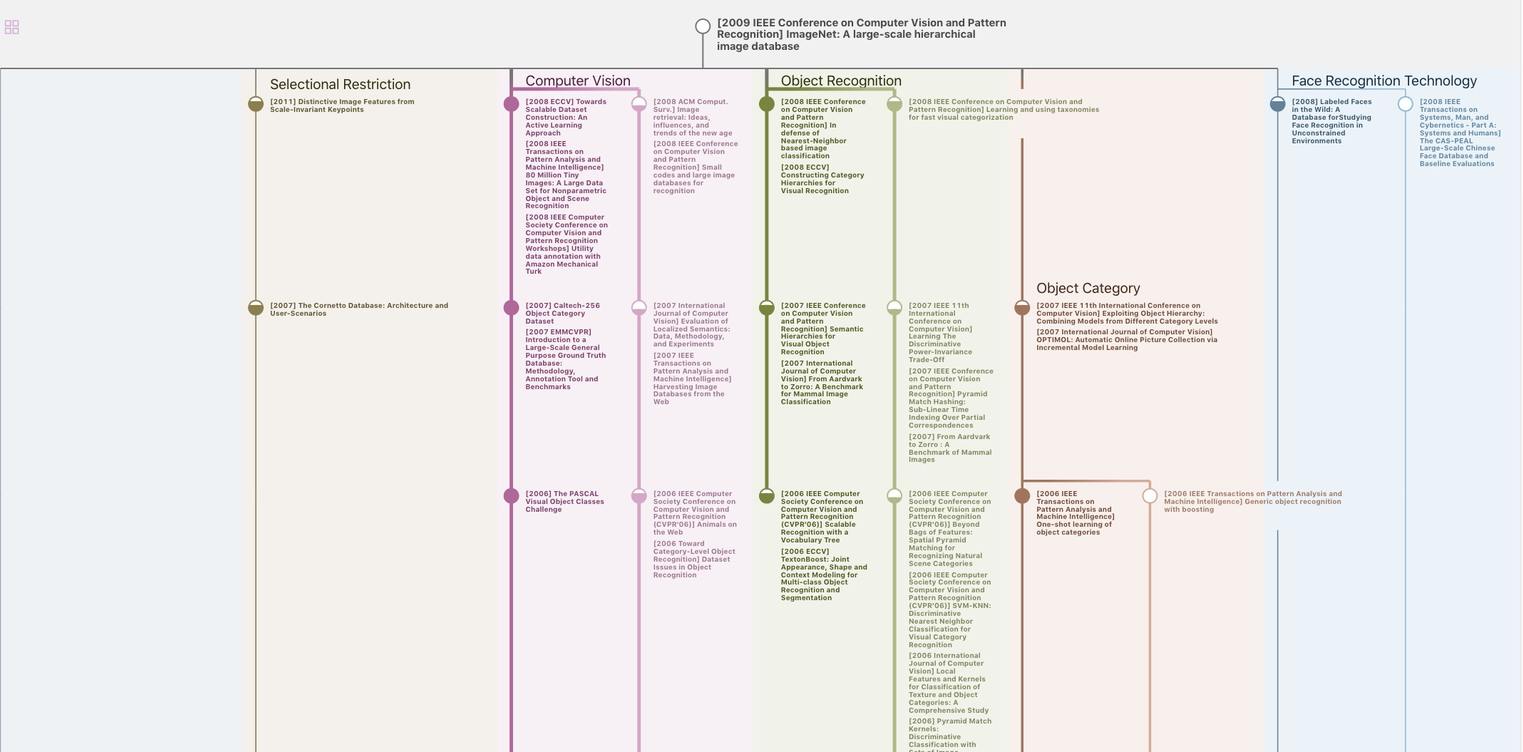
生成溯源树,研究论文发展脉络
Chat Paper
正在生成论文摘要