Influence of data quality, domain shift and measurement uncertainty on the prediction quality of smart sensor systems
TM-TECHNISCHES MESSEN(2023)
摘要
Artificial intelligence (AI) is almost ubiquitous in modern science and is also used in a variety of ways in measurement technology for sensor data fusion and extended signal evaluation in order to record variables that cannot be measured directly. To reliably apply machine learning specifically in an industrial context, data quality plays a crucial role in addition to the algorithms used. In AI research, the focus is on developing increasingly powerful algorithms, but not enough attention is paid to the data itself, its acquisition and annotation, especially in industry. Therefore, in practice, many ML projects fail due to insufficient data quality. To account for measurement uncertainty in the evaluation of measurement data, the GUM calls for error propagation to evaluate the result calculated based on an evaluation model. This concept can also be applied to ML models to estimate the effect of the measurement uncertainty of the input data on the ML result. The paper shows that a consistent application of metrological principles, especially calibration and measurement uncertainty evaluation, significantly improves the quality of AI models for industrial use and that the trend towards increasingly complex AI models, driven from computer science, is often in contradiction to the necessary robustness of the models.
更多查看译文
关键词
AI in metrology, data quality, measurement uncertainty
AI 理解论文
溯源树
样例
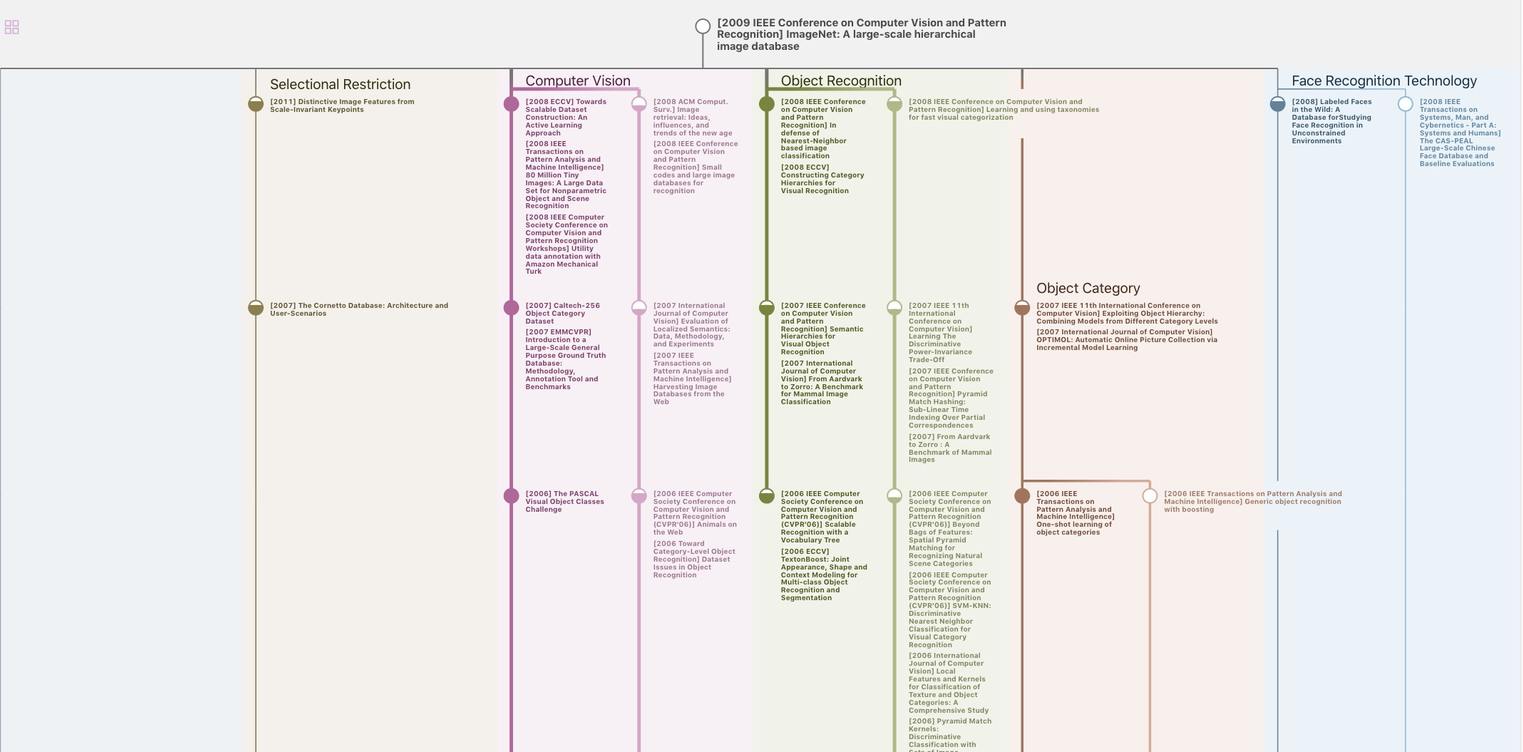
生成溯源树,研究论文发展脉络
Chat Paper
正在生成论文摘要