Mining frequent items from high-dimensional set-valued data under local differential privacy protection
EXPERT SYSTEMS WITH APPLICATIONS(2023)
摘要
Mining frequent items from high-dimensional historical data (set-valued data) from massive users can extract the most routine data, playing a vital role in data mining. However, frequent item mining requires the collection of a large amount of user data, posing the potential risk of leaking user privacy. Local differential privacy is a mainstream privacy-preserving technique. It has been widely used for user privacy protection in various scenarios as it provides strict privacy protection. Mining frequent items from high-dimensional set-valued data under local differential privacy preservation has recently attracted much attention from researchers. Existing works usually use random sampling to reduce the communication cost of publishing perturbed data but hardly guarantee frequent item mining accuracy. This is because they sample minimal items from each user’s high-dimensional set-value data (e.g., LDPMiner samples one item), making it difficult to focus on the scope of frequent item mining. The motivation of this paper to solve the above problem is that the frequent items mined from the data of a portion of users (e.g., half of the users) are similar to those mined from the data of the global users. Therefore, we randomly divide users into two groups and mine the set of candidate frequent items from the first group of users. Then, we focus on the candidate set in the second group of users and mine frequent items from it. Besides, we observe that the larger the sample size of user data, the better the frequent item mining accuracy and, subsequently, the higher the communication cost. Therefore, we randomly group the contents and randomly sample from each group, thus improving the frequent item mining accuracy by publishing more data than existing works. On this basis, we adaptively perturb the sampled group data according to the communication cost to trade off the communication cost and frequent item mining accuracy. Finally, we analyze the privacy and utility of our method theoretically. The experiments with state-of-the-art methods such as FIML, SVIM, and LDPMiner show that our proposed method improves about 15% in accuracy and 10% in utility for mining frequent items in high-dimensional set-valued data.
更多查看译文
关键词
Local differential privacy,Frequent item mining
AI 理解论文
溯源树
样例
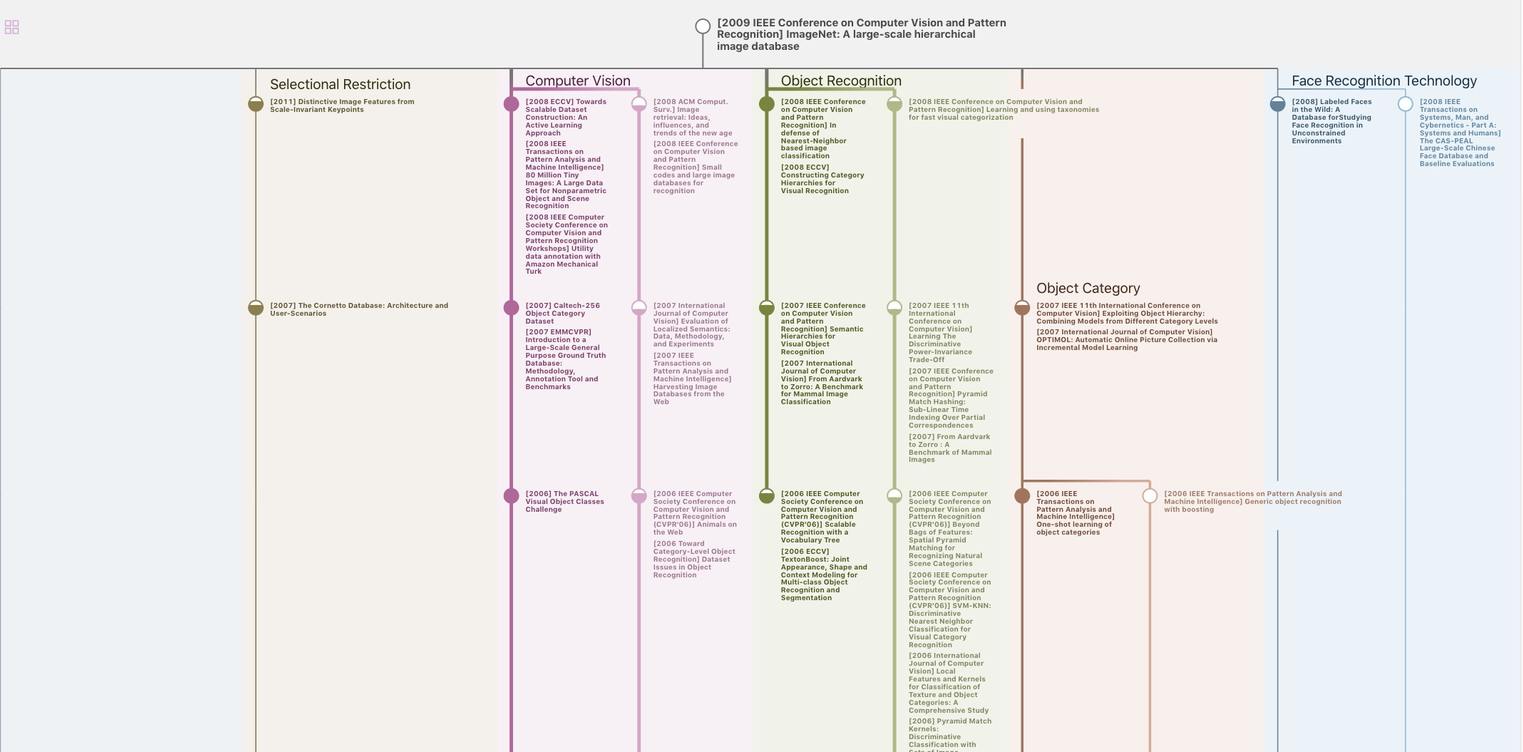
生成溯源树,研究论文发展脉络
Chat Paper
正在生成论文摘要