No-reference shadow detection quality assessment via reference learning and multi-mode exploring
COMPUTERS & GRAPHICS-UK(2023)
摘要
Shadow detection is a common computer vision task that identifies the location of shadows. It has been used as a key primitive for many high-level tasks such as object segmentation and video tracking. Due to the complexity and variety of shadows, it is challenging to design a one-size-fits-all shadow detection algorithm in real-world scenarios. Instead, it is often necessary in practice to pick the best shadow detection algorithm for a particular vision application. While shadow detection algorithms can be assessed by comparing the detected shadow with the ground truth, such ground truth is often unavailable in real-world vision systems consistently generating new data. In this paper, we propose a no-reference shadow detection quality assessment network (NSDQA-Net) to rank shadow detection algorithms without needing the ground truth. For a given image, the proposed assessment network first learns a reference map that compensates the false positives and false negatives of multiple input shadow detection algorithms. The reference map, as well as the original image and the input shadow detection results, are then used to regress the quality scores of the shadow detection methods. Our framework supports the assessment of one, two, or multiple algorithms in a single run, making it easier for deployment in practical scenarios. Experiments results show that the proposed network achieves optimal accuracy in no-reference shadow detection assessment. Relying on it for adaptive algorithm selection also improves the performance of shadow detection using a fixed algorithm on benchmark datasets. & COPY; 2023 Published by Elsevier Ltd.
更多查看译文
关键词
Shadow detection, No-reference quality assessment, Deep learning
AI 理解论文
溯源树
样例
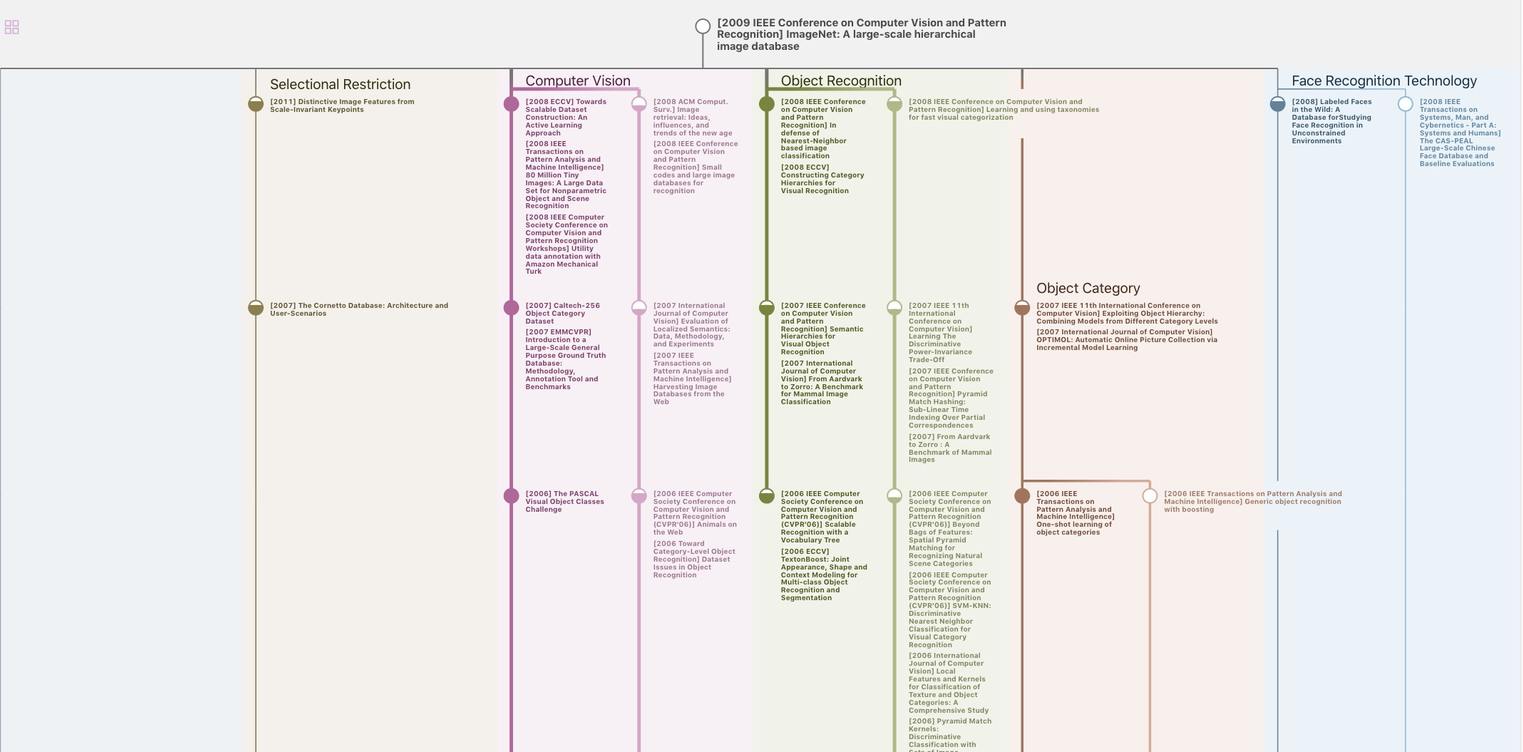
生成溯源树,研究论文发展脉络
Chat Paper
正在生成论文摘要