Global-and-local aware network for low-light image enhancement
ENGINEERING APPLICATIONS OF ARTIFICIAL INTELLIGENCE(2023)
摘要
Photos taken under nighttime or backlit conditions often suffer from complex and unpredictable degradation, such as low visibility, messy noise, and distorted color. Previous methods mainly focused on global brightness and contrast while ignoring structural and textural details, or they handled the fusion of features without adequately considering their intrinsic association, resulting in incomplete feature representations. To address this issue, we propose a global-and-local aware network (GLAN) by projecting the features into the frequency domain and incorporating them in a knowledge-sharing manner. This method effectively integrates the global modeling capability of the transformer and the local sensitivity of the convolutional neural network to represent structure and texture. First, the global branch, which is comprised of transformer blocks, performs feature extraction under the global receptive field, while the local branch constructs multi-scale features to provide local fine-grained details. Then, we design a novel adaptive multi-scale feature block (AMSFB) that deploys channel split operation to decrease the calculation amount. To better learn the channel and spatial correlations of intermediate features, we introduce a multi-scale channel attention module (MSCAM) and a pixel attention module (PAM) into the AMSFB. Finally, a frequency-aware interaction module (FAIM) is developed for bidirectional information supplementation, which builds feature descriptors simultaneously covering low-frequency and high-frequency information based on the discrete cosine transform (DCT). Through extensive quantitative and qualitative experiments, our method can achieve competitive results compared with over ten state-of-the-art image enhancement methods on eight benchmark datasets.
更多查看译文
关键词
Low-light image enhancement,Frequency aware,Attention mechanism,Transformer,Multi-scale feature
AI 理解论文
溯源树
样例
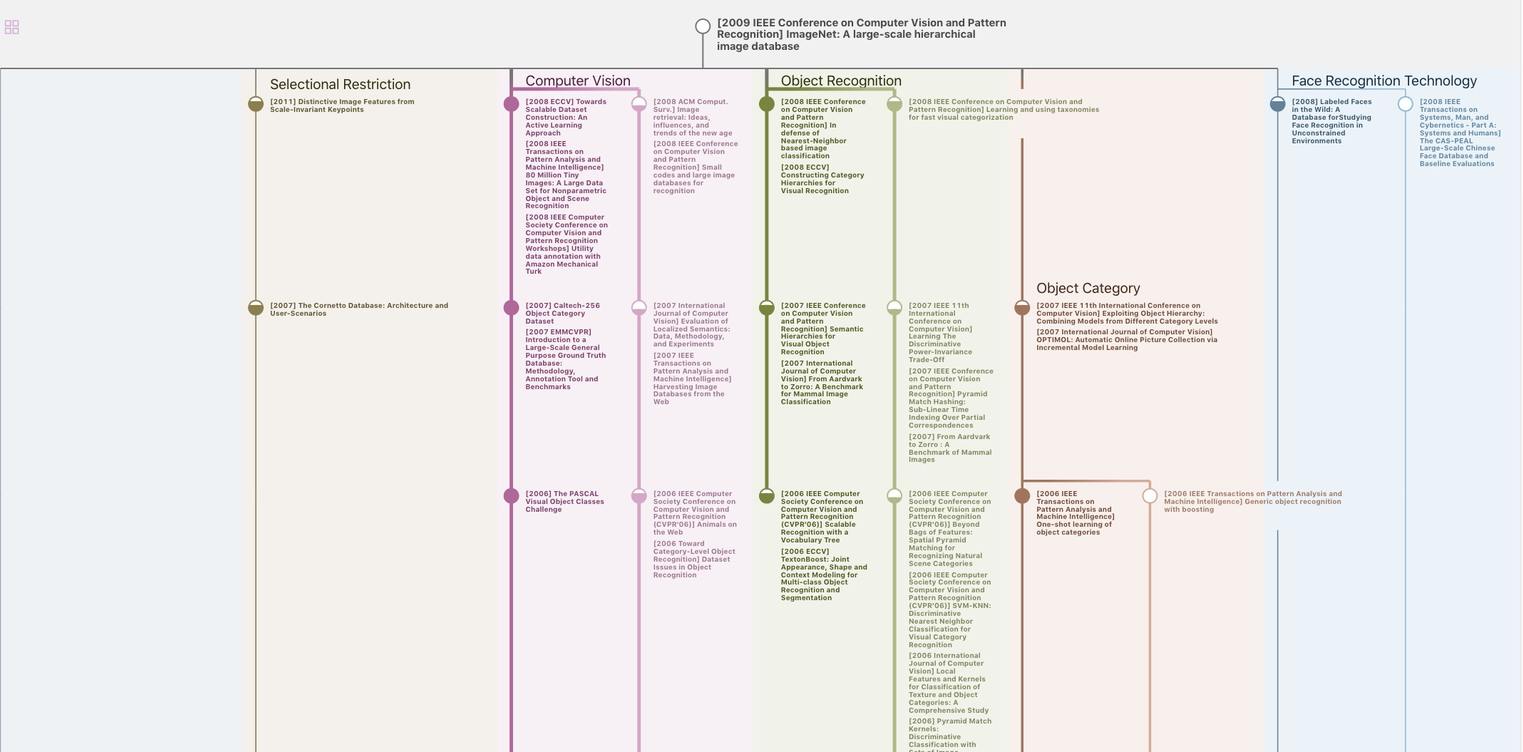
生成溯源树,研究论文发展脉络
Chat Paper
正在生成论文摘要