Co-Evolution With Deep Reinforcement Learning for Energy-Aware Distributed Heterogeneous Flexible Job Shop Scheduling
IEEE TRANSACTIONS ON SYSTEMS MAN CYBERNETICS-SYSTEMS(2024)
摘要
Energy-aware distributed heterogeneous flexible job shop scheduling (DHFJS) problem is an extension of the traditional FJS, which is harder to solve. This work aims to minimize total energy consumption (TEC) and makespan for DHFJS. A deep Q-networks-based co-evolution algorithm (DQCE) is proposed to solve this NP-hard problem, which includes four parts: First, a new co-evolutionary framework is proposed, which allocates sufficient computation to global searching and exe-cutes local search surrounding elite solutions. Next, nine problem features-based local search operators are designed to accelerate convergence. Moreover, deep Q-networks are applied to learn and select the best operator for each solution. Furthermore, an efficient heuristic method is proposed to reduce TEC. Finally, 20 instances and a real-world case are employed to evalu-ate the effectiveness of DQCE. Experimental results indicate that DQCE outperforms the six state-of-the-art algorithms for DHFJS.
更多查看译文
关键词
Co-evolution,deep Q-networks (DQNs),distributed heterogeneous flexible job shop scheduling (DHFJS) problem,energy-saving,multiobjective optimization
AI 理解论文
溯源树
样例
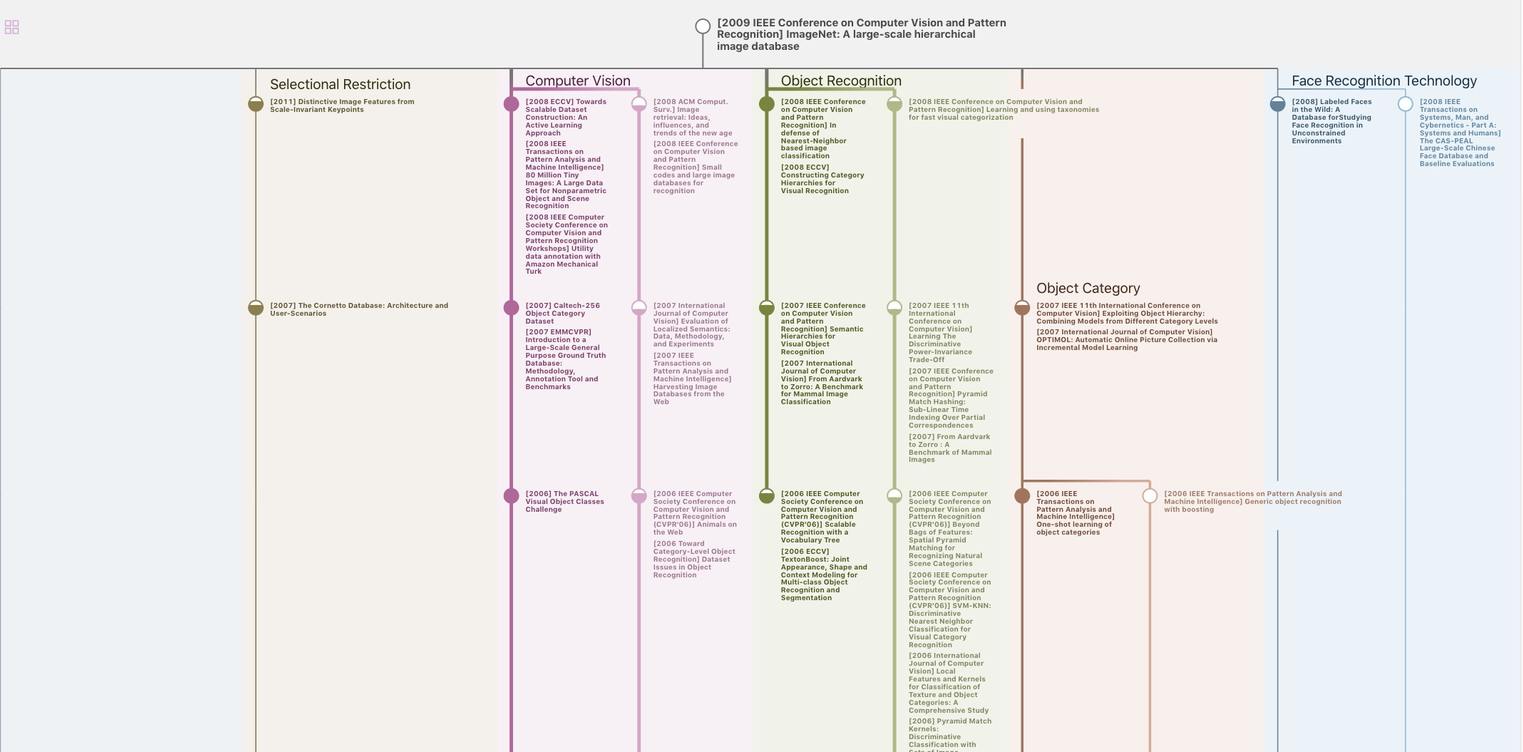
生成溯源树,研究论文发展脉络
Chat Paper
正在生成论文摘要