Graph convolutional network for difficulty-controllable visual question generation
WORLD WIDE WEB-INTERNET AND WEB INFORMATION SYSTEMS(2023)
摘要
In this article, we address the problem of difficulty-controllable visual question generation, which is to generate questions that satisfy the given difficulty levels based on the images and target answer. The existing approach seems to generate questions following templates. For easy questions, the model presents both answers and it becomes a choice question; while for hard questions, the answer set is not part of the question. In fact, question difficulty should be reflected by the objects and their relationships in the question. Towards this end, we propose a graph-based model with three concrete modules: Difficulty-controllable Graph Convolutional Network (DGCN) module, fusion module and difficulty-controllable decoder, to generate questions with a controllable level of difficulty. We first define a difficulty label based on the difficult index from the education area to represent the difficulty of a question. Next, a DGCN module is proposed to learn image representations that capture relations between objects in an image conditioned on a given difficulty label. Then, we use a fusion module to jointly attend the image representations and answer representations to capture answer-related image features. Finally, a difficulty-controllable decoder combines difficulty information into decoder initialization and input at each time step to control the difficulty of generated questions. Experimental results demonstrate that our framework not only achieves significant improvements on several automatic evaluation metrics, but also can generate difficulty-controllable questions.
更多查看译文
关键词
Miltimodal,Visual question generation,Difficulty-controllable,Graph convolutional network
AI 理解论文
溯源树
样例
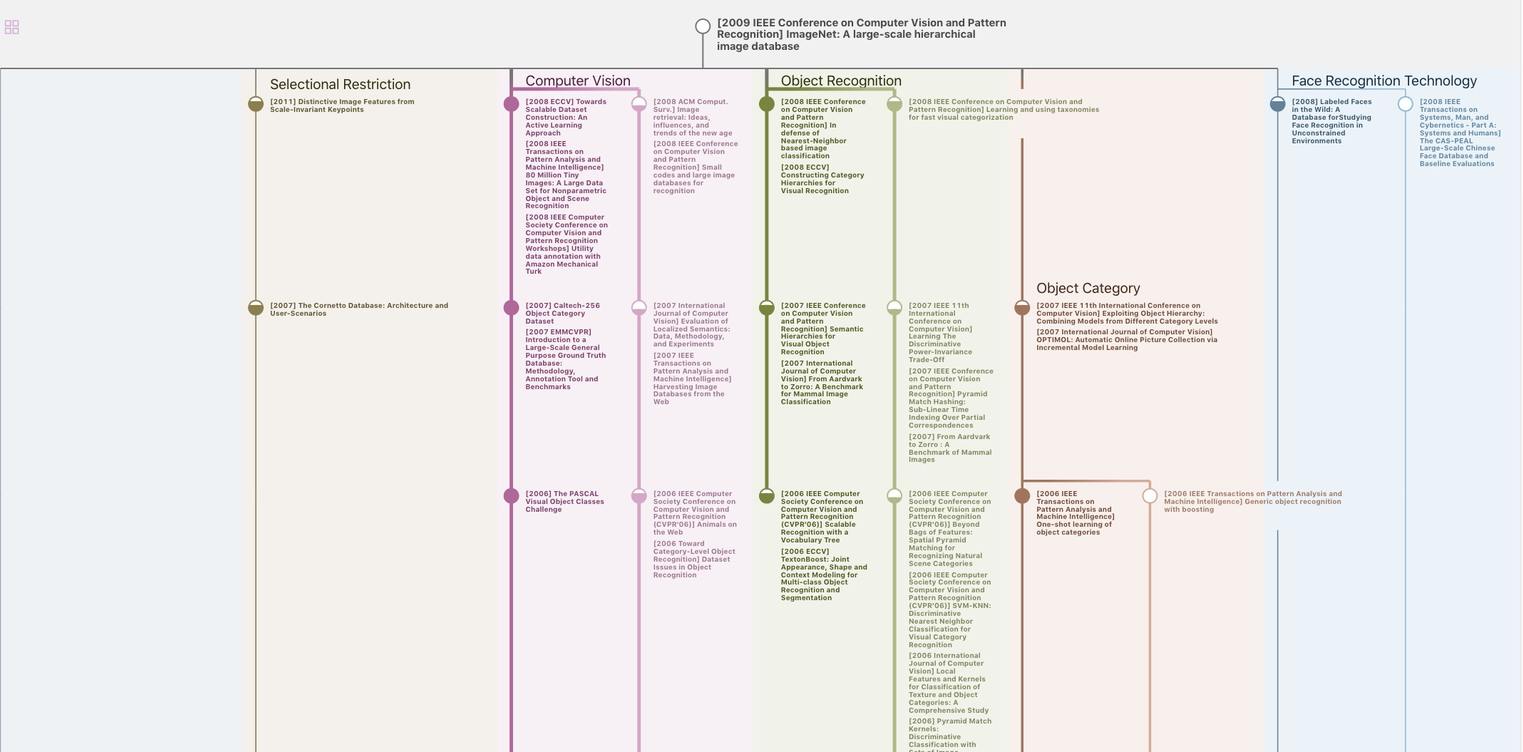
生成溯源树,研究论文发展脉络
Chat Paper
正在生成论文摘要