MulTCIM: Digital Computing-in-Memory-Based Multimodal Transformer Accelerator With Attention-Token-Bit Hybrid Sparsity
IEEE JOURNAL OF SOLID-STATE CIRCUITS(2024)
摘要
Multimodal Transformers are emerging artificial intelligence (AI) models that comprehend a mixture of signals from different modalities like vision, natural language, and speech. The attention mechanism and massive matrix multiplications (MMs) cause high latency and energy. Prior work has shown that a digital computing-in-memory (CIM) network can be an efficient architecture to process Transformers while maintaining high accuracy. To further improve energy efficiency, attention-token-bit hybrid sparsity in multimodal Transformers can be exploited. The hybrid sparsity significantly reduces computation, but the irregularity also harms CIM utilization. To fully utilize the attention-token-bit hybrid sparsity of multimodal Transformers, we design a digital CIM-based accelerator called MulTCIM with three corresponding features: The long reuse elimination dynamically reshapes the attention pattern to improve CIM utilization. The runtime token pruner (RTP) removes insignificant tokens, and the modal-adaptive CIM network (MACN) exploits symmetric modal overlapping to reduce CIM idleness. The effective bitwidth-balanced CIM (EBB-CIM) macro balances input bits across in-memory multiply-accumulations (MACs) to reduce computation time. The fabricated MulTCIM consumes only 2.24 mu J/Token for the ViLBERT-base model, achieving 2.50x-5.91x lower energy than previous Transformer accelerators and digital CIM accelerators.
更多查看译文
关键词
Computing-in-memory (CIM),dataflow,hybrid sparsity,multimodal transformers,reconfigurable architecture
AI 理解论文
溯源树
样例
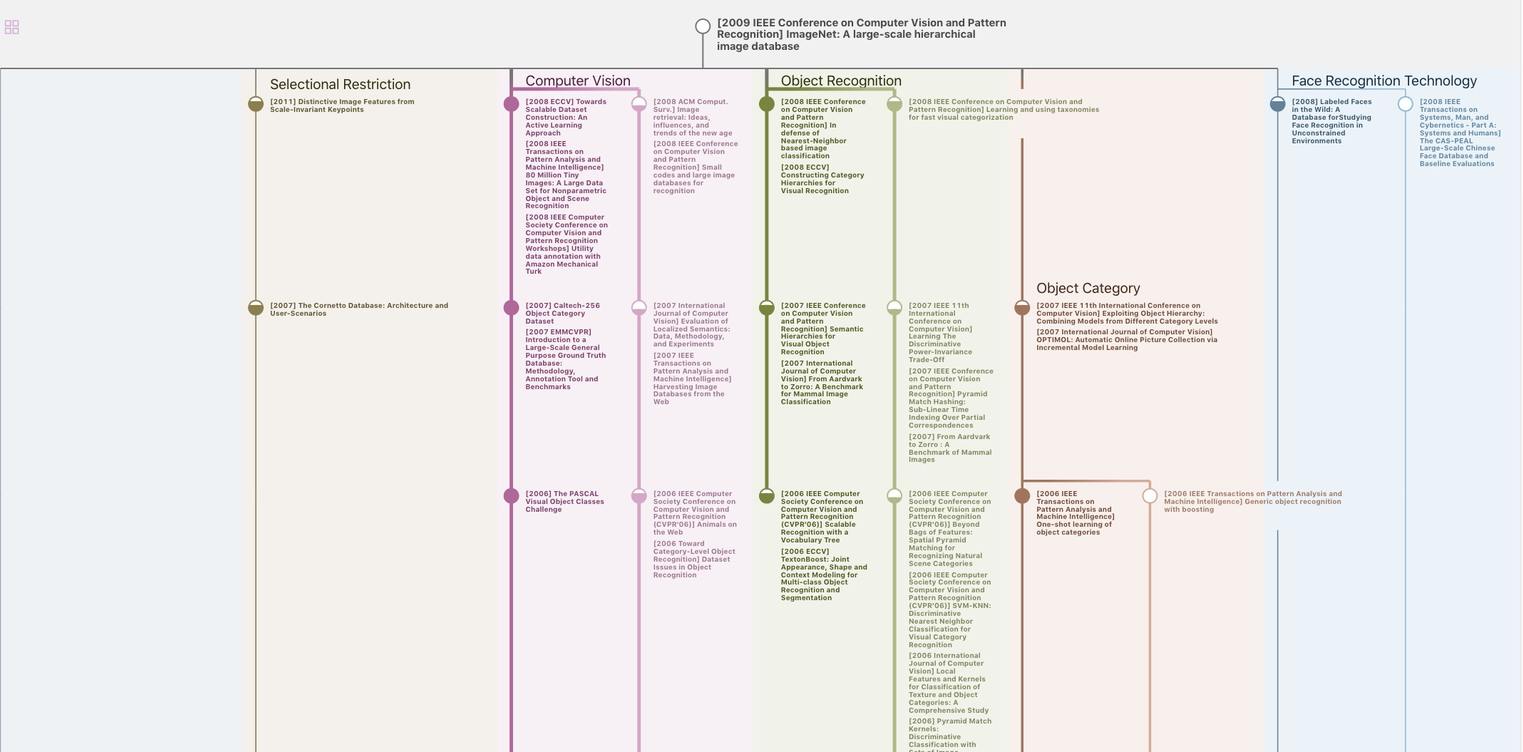
生成溯源树,研究论文发展脉络
Chat Paper
正在生成论文摘要