Deep learning with whole slides images to predict histological response to neoadjuvant chemotherapy in patients with resectable high grade soft-tissue sarcomas: A multicenter study
JOURNAL OF CLINICAL ONCOLOGY(2023)
摘要
11512 Background: Two recent randomized trials have confirmed the potential benefit of neoadjuvant anthracycline-based chemotherapy in patients with high-grade soft-tissue sarcomas (STS) (Issels et al 2018, Gronchi et al 2017). However, as for adjuvant chemotherapy, the main issue with neoadjuvant treatment of STS patients is the identification of predictive factors of response to chemotherapy that could avoid for a majority of patients ineffective and potentially toxic treatment with impaired quality of life. Histological response, defined as the percentage of stainable tumor cells on the surgical specimen, has been shown to be a reliable surrogate for metastasis-free survival and overall survival in bone but also in soft-tissue sarcomas (Crombe et al JCO Clin Inf 2021). Developing a tool to help medical oncologists predict the potential benefit of neoadjuvant chemotherapy prior to starting the treatment would be of great value for precision medicine in sarcomas. To address this need, we propose a deep learning-based approach to predict histological response to neoadjuvant chemotherapy patients from routine histological whole slide images sampled at diagnosis. Methods: A cohort of 220 patients with high grade limb and trunk wall STS enrolled in the NEOSARCOMICS study (NCT02789384, sponsor: Institut Bergonié, Bordeaux, France) was used to train a model to predict the binarized histological response from diagnostic biopsies. Responders are defined by a histological response inferior or equal to 10%. Digitized hematoxylin/eosin slides were preprocessed to segment the tissue and decompose the image into patches of smaller resolution. Features were extracted from each patch using a Vision Transformer pretrained in a self-supervised fashion on histology slides. A Multiple Instance Learning model was trained on the resulting features to compute a prediction at the patient level. The algorithm was trained and validated through a 5-fold, 5-repeat cross-validation scheme. Finally, external validation is performed on an unseen cohort from Centre Léon Bérard to assess the robustness of the model. Results: To predict histological response to neoadjuvant chemotherapy, a model using only diagnostic biopsies achieved an AUC of 0.69 (95% confidence interval, 0.60-0.76). External validation is ongoing and shows promising preliminary results with an AUC of 0.67 (95% confidence interval with bootstrapping, 0.45-0.89). Conclusions: This multi-center study demonstrates that deep-learning models can accurately predict the histological response to neoadjuvant chemotherapy from routine histological whole slide images sampled at diagnosis. This model could be used as a pre-screening tool to improve patient selection for neoadjuvant chemotherapy in STS.
更多查看译文
关键词
neoadjuvant chemotherapy,whole slides images,deep,soft-tissue
AI 理解论文
溯源树
样例
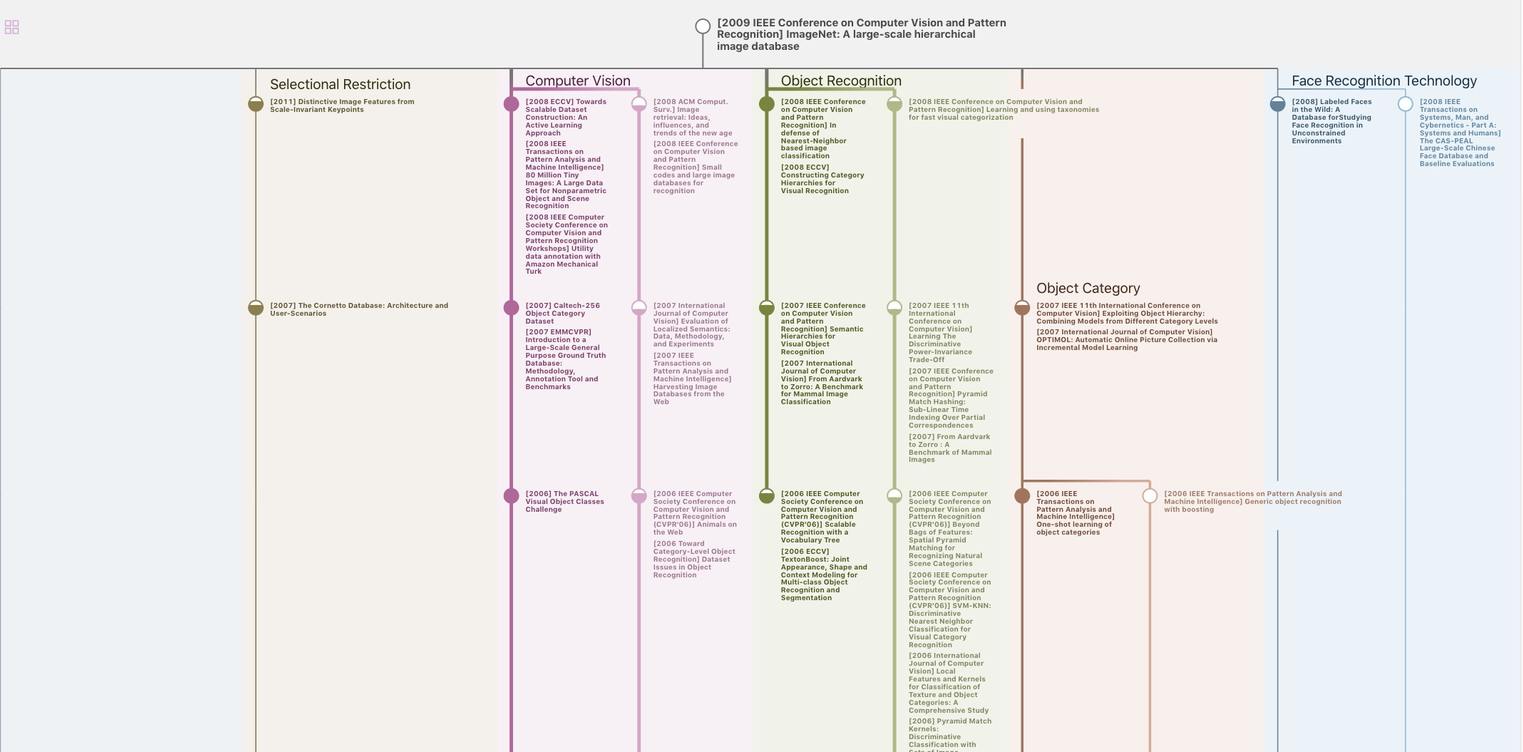
生成溯源树,研究论文发展脉络
Chat Paper
正在生成论文摘要