Research on Energy Management of Hydrogen Fuel Cell Bus Based on Deep Reinforcement Learning Considering Velocity Control
SUSTAINABILITY(2023)
摘要
In the vehicle-to-everything scenario, the fuel cell bus can accurately obtain the surrounding traffic information, and quickly optimize the energy management problem while controlling its own safe and efficient driving. This paper proposes an energy management strategy (EMS) that considers speed control based on deep reinforcement learning (DRL) in complex traffic scenarios. Using SUMO simulation software (Version 1.15.0), a two-lane urban expressway is designed as a traffic scenario, and a hydrogen fuel cell bus speed control and energy management system is designed through the soft actor-critic (SAC) algorithm to effectively reduce the equivalent hydrogen consumption and fuel cell output power fluctuation while ensuring the safe, efficient and smooth driving of the vehicle. Compared with the SUMO-IDM car-following model, the average speed of vehicles is kept the same, and the average acceleration and acceleration change value decrease by 10.22% and 11.57% respectively. Compared with deep deterministic policy gradient (DDPG), the average speed is increased by 1.18%, and the average acceleration and acceleration change value are decreased by 4.82% and 5.31% respectively. In terms of energy management, the hydrogen consumption of SAC-OPT-based energy management strategy reaches 95.52% of that of the DP algorithm, and the fluctuation range is reduced by 32.65%. Compared with SAC strategy, the fluctuation amplitude is reduced by 15.29%, which effectively improves the durability of fuel cells.
更多查看译文
关键词
fuel cell bus,deep reinforcement learning,vehicle velocity control,energy management strategy
AI 理解论文
溯源树
样例
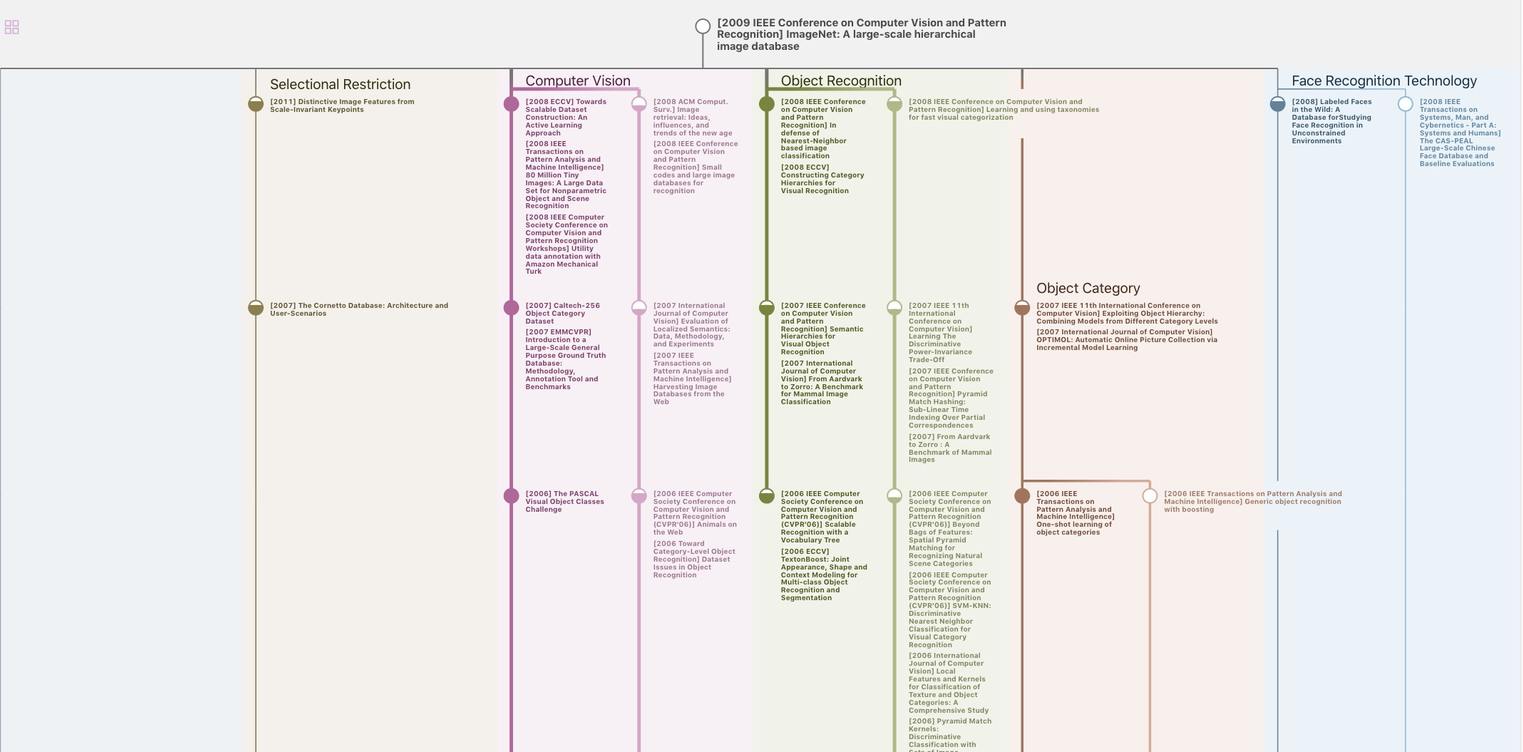
生成溯源树,研究论文发展脉络
Chat Paper
正在生成论文摘要