Cross Pyramid Transformer makes U-net stronger in medical image segmentation
BIOMEDICAL SIGNAL PROCESSING AND CONTROL(2023)
摘要
Accurate auto-medical image segmentation, which is essential in disease diagnosis and treatment planning, has been significantly prospered by recent advances in Convolution Neural Network (CNN) and Transformer. However, pure CNN-based or pure Transformer-base architectures exhibit limitations for segmentation tasks. For example, CNN fails to capture long-range relations due to fix receptive fields, and Transformer ignores pixel-level spatial details of features. To address these challenges, we propose a novel parallel hybrid architecture for medical image segmentation, which is named CPT-Unet (Cross Pyramid Transformer U-shape Network). CPT-Unet may be the first attempt to exploit both the advantages of Pyramid Vision Transformer (PVT) and CNN to the full by integrating them into the standard U-shape network to improve the segmentation performance and inference time. Specifically, we design a parallel dual branch encoder and decoder in CPT-Unet that consists of CNN and PVT. The input image is fed into the encoder of these two branches simultaneously to extract low-level spatial details and global contexts in a much shallower way. We design a novel fusion strategy to adequately utilize the multi-scale features extracted from CNN and PVT. We also add PVT in the decoder to better restore the segmentation map. Experiments on two public segmentation datasets demonstrate the improved performance of the proposed CPT-Unet over the comparison methods. The source code is available at https://github.com/ShengYue007/CPT-Unet.git.
更多查看译文
关键词
Multi scale global context,Pyramid vision transformer,Medical image segmentation,Convolutional neural network,U-Shaped Network,Parallel branch
AI 理解论文
溯源树
样例
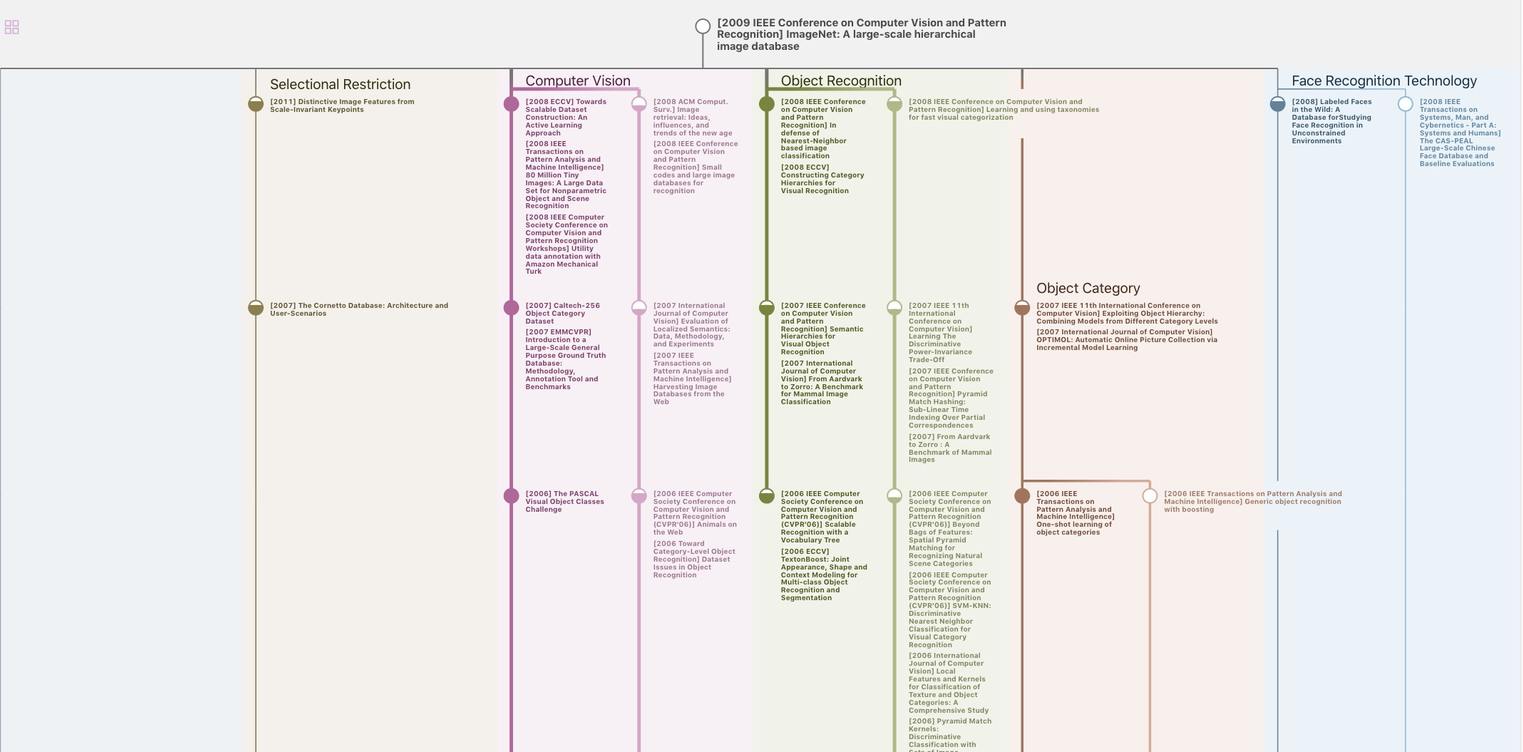
生成溯源树,研究论文发展脉络
Chat Paper
正在生成论文摘要