Deep Representation of EEG Signals Using Spatio-Spectral Feature Images
APPLIED SCIENCES-BASEL(2023)
摘要
Modern deep neural networks (DNNs) have shown promising results in brain studies involving multi-channel electroencephalogram (EEG) signals. The representations produced by the layers of a DNN trained on EEG signals remain, however, poorly understood. In this paper, we propose an approach to interpret deep representations of EEG signals. Our approach produces spatio-spectral feature images (SSFIs) that encode the EEG input patterns that activate the neurons in each layer of a DNN. We evaluate our approach using the PhyAAt dataset of multi-channel EEG signals for auditory attention. First, we train the same convolutional neural network (CNN) architecture on 25 separate sets of EEG signals from 25 subjects and conduct individual model analysis and inter-subject dependency analysis. Then we generate the SSFI input patterns that activate the layers of each trained CNN. The generated SSFI patterns can identify the main brain regions involved in a given auditory task. Our results show that low-level CNN features focus on larger regions and high-level features focus on smaller regions. In addition, our approach allows us to discern patterns in different frequency bands. Further SSFI saliency analysis reveals common brain regions associated with a specific activity for each subject. Our approach to investigate deep representations using SSFI can be used to enhance our understanding of the brain activity and effectively realize transfer learning.
更多查看译文
关键词
brain–computer interface,EEG,spatio-spectral feature image,deep neural network,convolutional neural network,deep representation
AI 理解论文
溯源树
样例
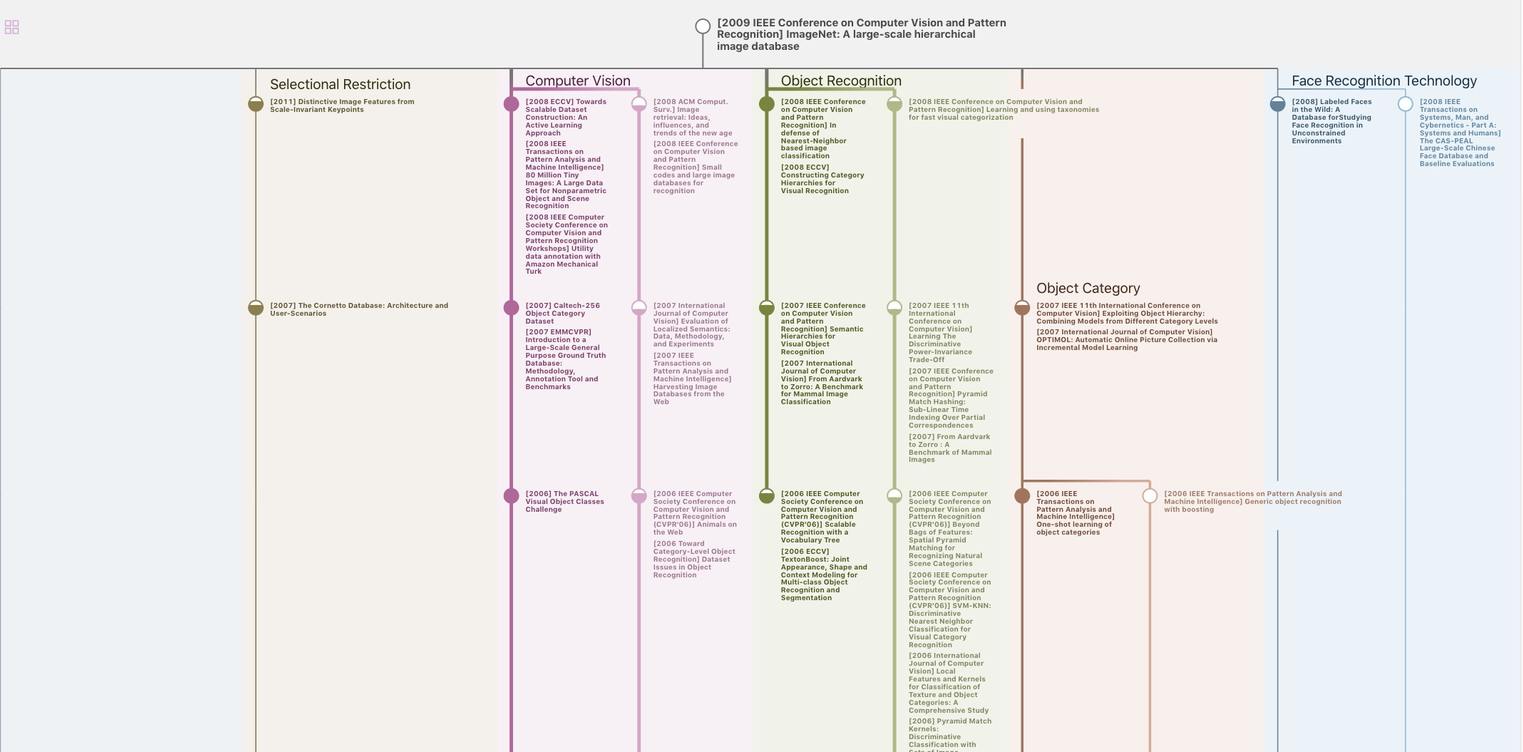
生成溯源树,研究论文发展脉络
Chat Paper
正在生成论文摘要