Deep Hybrid Compression Network for Lidar Point Cloud Classification and Segmentation
REMOTE SENSING(2023)
摘要
Extensive research on deep neural networks for LiDAR point clouds has contributed inexhaustible momentum to the development of computer 3D vision applications. However, storage and energy consumption have always been a challenge for deploying these deep models on mobile devices. Quantization provides a feasible route, of which current primary research is focused on uniform bit-width quantization without considering different layers or filters' sensitivity to different bit-widths. This article proposes a novel hybrid compression method based on relaxed mixed-precision quantization, relaxed weights pruning, and knowledge distillation to overcome the limitations of uniform quantization illustrated above, while further improving model accuracy and reducing model memory consumption. It employs a differentiable searching method to search for the optimal bit allocation and weight sparsity, while conducting feature distillation, accordingly considering the feature degradation by pooling operation in point cloud deep models. The proposed method combines three compression techniques to balance the trade-off between compression accuracy and model size. Pruning alleviates the increasing memory consumption problem caused by mixed-precision quantization, while distillation improves compression accuracy without increasing model size. The experiments validate that the proposed method outperforms state-of-the-art typical uniform quantization methods in terms of accuracy with an acceptable and relatively competitive compression performance.
更多查看译文
关键词
differential, mixed-precision quantization, pruning, knowledge distillation, Lidar, point cloud
AI 理解论文
溯源树
样例
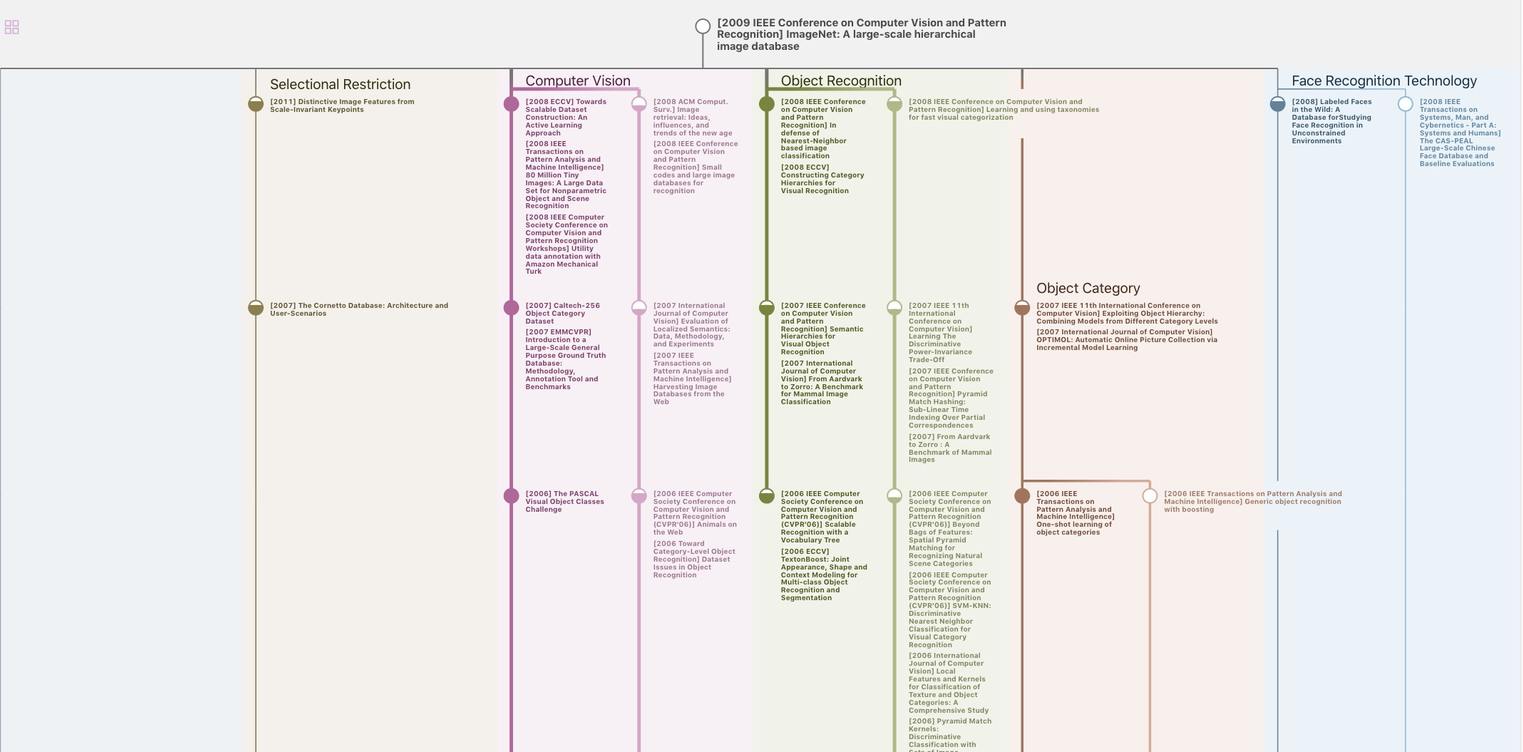
生成溯源树,研究论文发展脉络
Chat Paper
正在生成论文摘要