Review of resampling techniques for the treatment of imbalanced industrial data classification in equipment condition monitoring
Engineering Applications of Artificial Intelligence(2023)
摘要
In an actual industrial scenario, machines typically operate normally for the majority of the time, with malfunctions occurring only occasionally. As a result, there is very little recorded data on defects. Consequently, the fault diagnostic dataset becomes imbalanced, with a significantly lower number of fault samples compared to normal samples. Furthermore, with the rapid development of the manufacturing industry, the increasing complexity of machines and equipment leads to various challenges in collecting fault data, such as noise, within-class imbalance, multi-class imbalance, and time series imbalance. It is worth noting that this study is the first to comprehensively summarize these four specific challenges. Therefore, addressing these issues has become a critical research focus and a pain point in the field of fault diagnosis, and numerous solutions have emerged. This study provides a comprehensive overview of these solutions at three levels: data preprocessing, feature extraction, and classifier improvement. It also describes the applications of imbalanced data classification methods, including pure resampling techniques, as well as sampling techniques that combine resampling algorithms with feature extraction and classifier improvement in industrial scenarios. Finally, we summarize the challenges facing imbalanced data classification research and suggest potential directions for future studies.
更多查看译文
关键词
Fault diagnosis,Imbalanced classification problems,Four specific challenges,Resampling-based classification methods
AI 理解论文
溯源树
样例
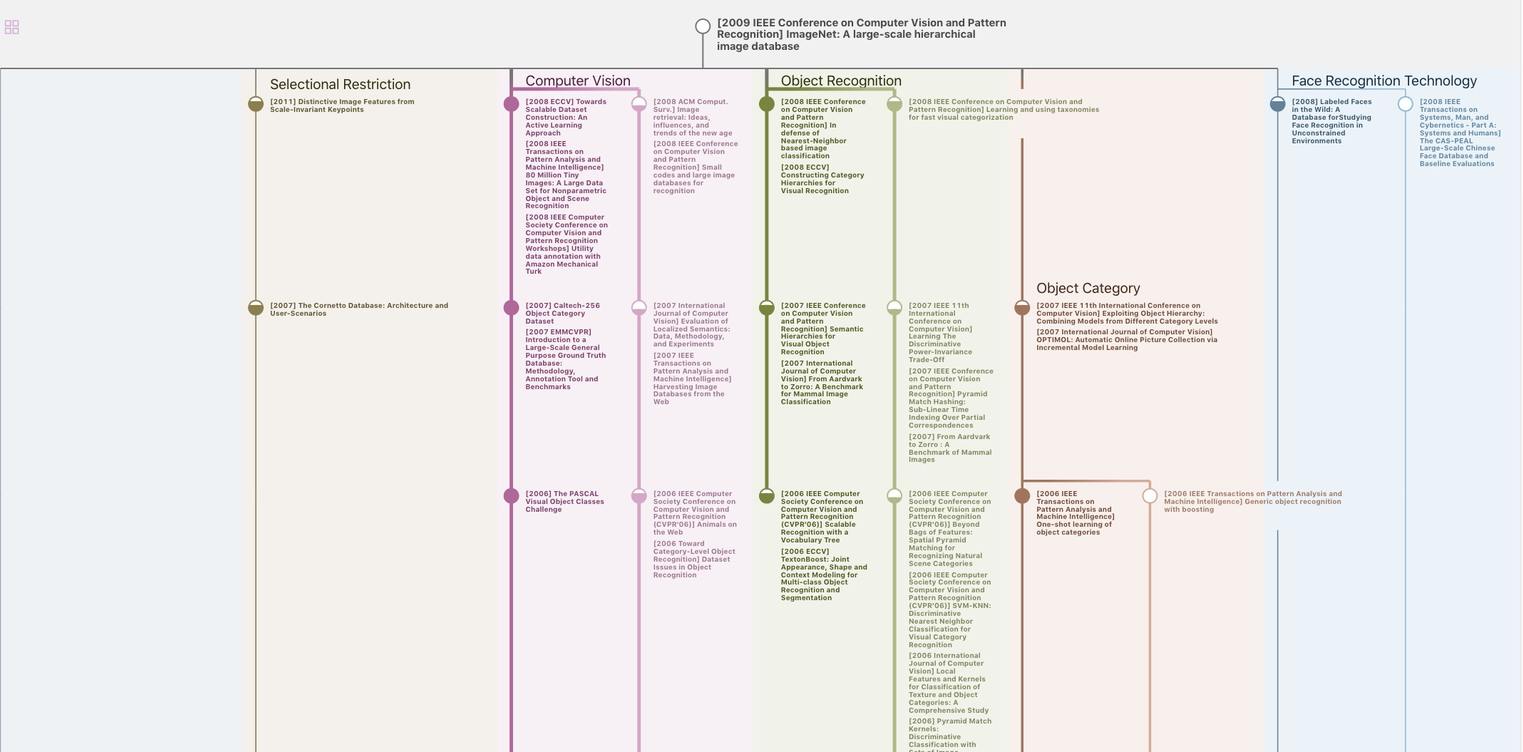
生成溯源树,研究论文发展脉络
Chat Paper
正在生成论文摘要