Physics-informed few-shot deep learning for elastoplastic constitutive relationships
ENGINEERING APPLICATIONS OF ARTIFICIAL INTELLIGENCE(2023)
摘要
Elastoplastic modeling is essential for accurately predicting material behavior in various engineering applications. However, existing approaches to developing intelligent models for elastoplasticity face limitations due to the scarcity of available data, particularly in cases where experiments cannot support full training of these models. To address this challenge, we propose a physics-informed few-shot learning framework that incorporates classic elastoplasticity theory as prior knowledge. Instead of directly solving specific constitutive ordinary differential equations, we utilize general mechanical inequations as physical regularization to confine the optimization space. Our framework is model-agnostic in the uniaxial scenario and free from complicated numerical implementation, preserving the advantages of the data-driven paradigm in terms of efficiency and simplicity. In the multiaxial scenario, the framework can automatically calibrate multiple interwoven material parameters of the underlying constitutive model, facilitating the generation of ample multiaxial data for downstream learning. To further improve convergence, we introduce a novel training strategy. A numerical experiments with only 32 pieces of training data validate the effectiveness of the developed framework. The trained uniaxial model exhibits exceptional generalization capabilities, achieving 44.9% higher accuracy than the purely data-driven model. For multiaxial relationships, our framework demonstrates remarkable efficiency in calibrating material constants compared to conventional machine or manual methods, and the trained model accurately reproduces highly nonlinear multiaxial elastoplastic curves. Our work addresses the pressing need for accurate elastoplastic modeling in the absence of large datasets and provides a promising new solution that can significantly improve generalization capabilities.
更多查看译文
关键词
Few-shot learning,Elastoplastic relationships,Deep learning,Constitutive model,Automatic calibration,Physics-informed machine learning
AI 理解论文
溯源树
样例
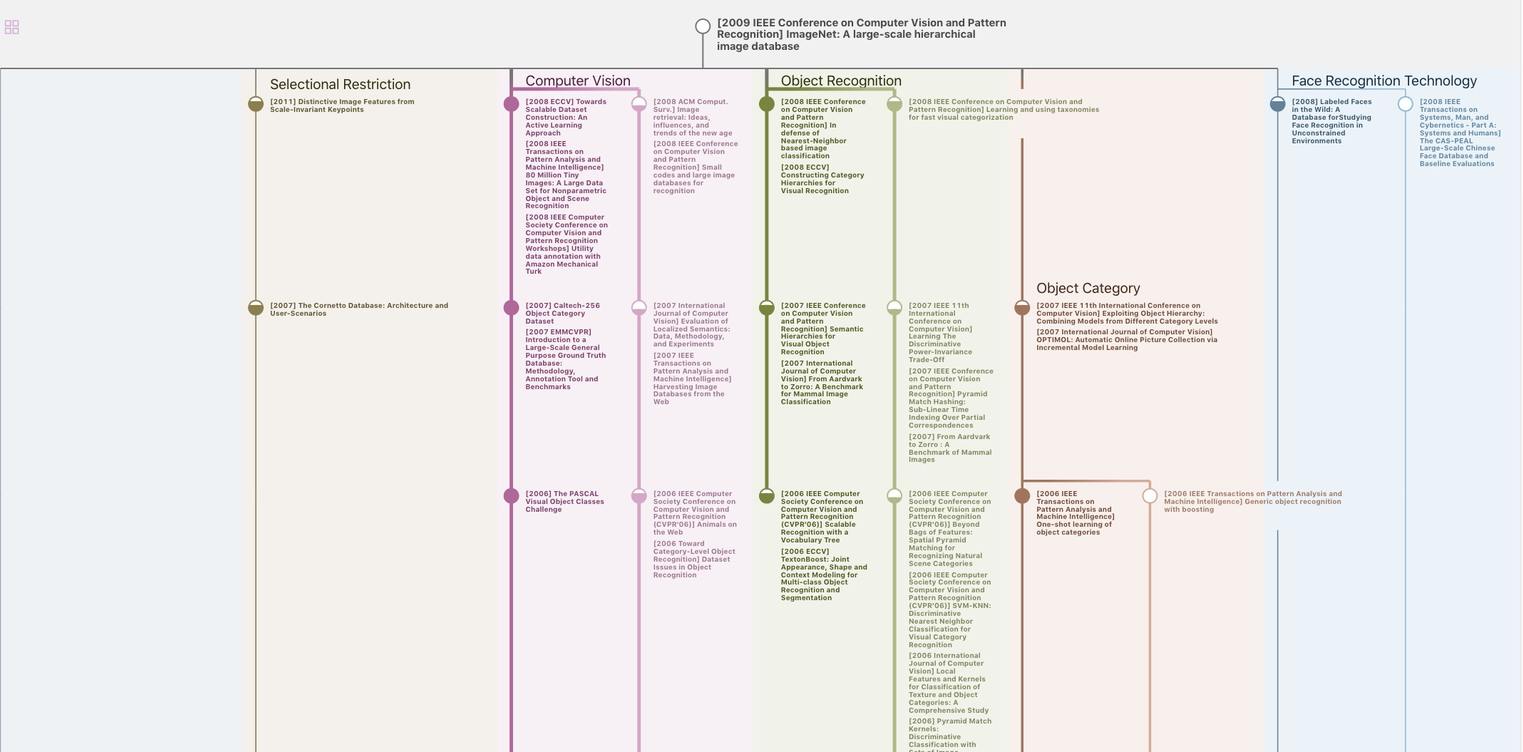
生成溯源树,研究论文发展脉络
Chat Paper
正在生成论文摘要