Fault Diagnosis of Marine Diesel Engines under Partial Set and Cross Working Conditions Based on Transfer Learning
JOURNAL OF MARINE SCIENCE AND ENGINEERING(2023)
摘要
The development of intelligent ships has an urgent demand for intelligent fault diagnosis technology. The working conditions and fault modes of high-power marine diesel engines gradually tend to be diversified and complicated, and the problems of reliability and safety are becoming more and more prominent. There are a lot of working condition data that lack fault labels, and the fault modes are asymmetric among different working conditions, so it is urgent to study effective fault diagnosis methods. Taking a marine diesel engine as the case validation object, we set up cross-condition and partial set fault diagnosis scenarios, proposed transferring knowledge from the source condition to the target condition for the problem of the lack of fault labels in the target condition, and designed a multi-scale and multi-view domain adversarial network (MMDAN) method for experimental validation using 6S50MC-C7 marine diesel engine system operation data. According to the experimental results, the average diagnostic accuracy of this method reached 96.58%, with a short processing time. Furthermore, it exhibits superior diagnostic performance compared to other transfer learning models in the cross-condition partial set transfer task. Additionally, the method proposed in this paper also offers a new approach and reference for the intelligent diagnosis of other equipment in ships.
更多查看译文
关键词
transfer learning,marine diesel engines,fault,cross working conditions
AI 理解论文
溯源树
样例
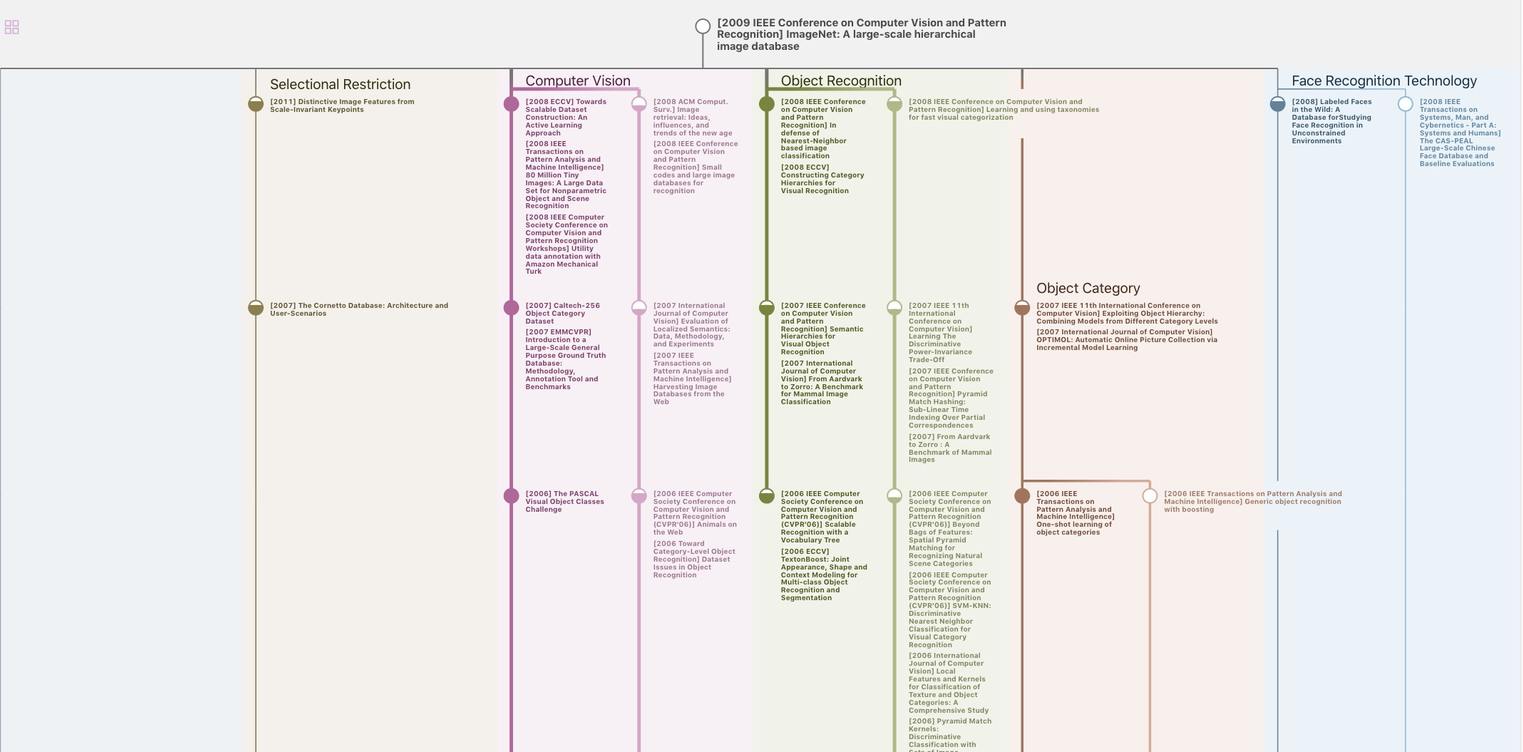
生成溯源树,研究论文发展脉络
Chat Paper
正在生成论文摘要