A novel pre-trained model based on graph-labeling graph neural networks for tool wear prediction under variable working conditions
MEASUREMENT SCIENCE AND TECHNOLOGY(2023)
摘要
In recent years, deep learning has made significant strides and found extensive applications in tool wear prediction. However, most methods based on deep learning rely on large-scale datasets for training and do not consider the method under variable working conditions. To address these limitations, this paper proposes a novel pre-trained fine-tuning model based on the graph-labeling graph neural network (GGPT) specifically designed to train on small-scale datasets. The GGPT model comprises two essential components: a feature extractor and a graph attention module (GAT). The feature extractor uses a residual connection constructed by maximum pooling and average pooling to improve the reliability of local feature learning. The GAT module enhances the global feature learning ability of the model by aggregating local features extracted by the feature extractor. In addition, Improved Pseudo-task Regularization is introduced to enable the model to converge better on small datasets. Experimental results substantiate the superiority of the GGPT model over other deep learning-based methods, particularly when confronted with variable working conditions for tool wear prediction.
更多查看译文
关键词
tool wear prediction, small samples, graph neural network, graph-labeling graph
AI 理解论文
溯源树
样例
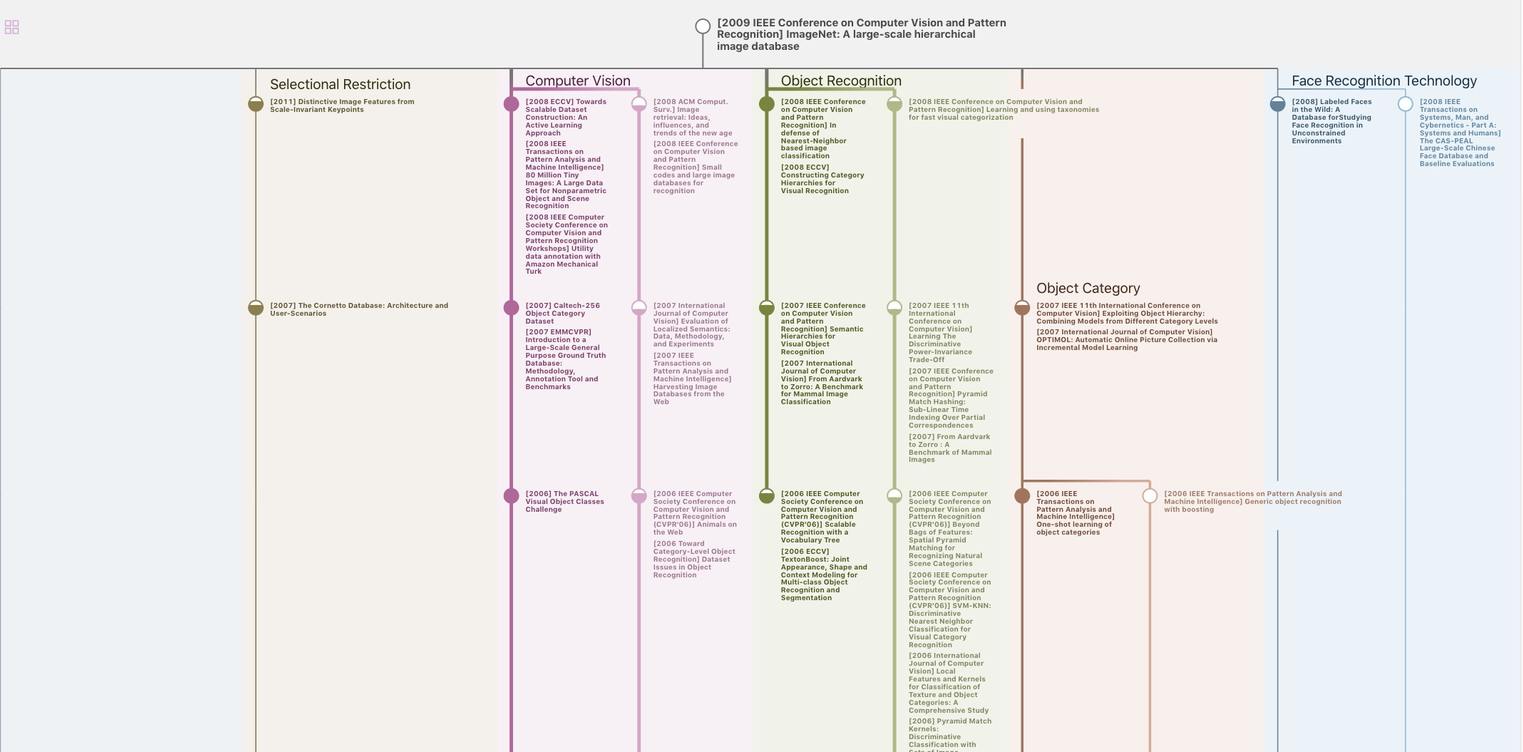
生成溯源树,研究论文发展脉络
Chat Paper
正在生成论文摘要