Meteorological influences on PM2.5 variation in China using a hybrid model of machine learning and the Kolmogorov-Zurbenko filter
ATMOSPHERIC POLLUTION RESEARCH(2023)
摘要
Quantitatively evaluating the contribution of meteorology and emissions to PM2.5 reduction during the Three-year Action Plan to Fight Air Pollution (2018-2020) is important to guide future emission control measures in China. In this study, a Kolmogorov-Zurbenko (KZ) filter and an artificial neural network (ANN) combined model was used to separate the meteorology-influenced PM2.5 concentration by considering seven meteorological factors including boundary layer height, surface pressure, surface net solar radiation, total precipitation, at-mospheric temperature, wind speed and wind direction in China. To compensate for the black-box nature of the ANN, the Shapley additive explanation (SHAP) value was introduced to explain the effect of each meteorological factor on long-term PM2.5 concentration. Results showed that PM2.5 concentration decreased by 14% from 2018 to 2020 in China with annual average concentrations of 37.19, 35.28, and 31.94 & mu;g/m3, respectively. Although the decline in PM2.5 was mainly due to emission reductions (58% for all of China), the contribution of prevailing meteorological conditions should not be ignored, especially in some regions such as Northeast China, which had the highest contribution of meteorology (58%) on PM2.5 reduction. In general, the contributions of meteoro-logical conditions to the long-term trend of PM2.5 varied greatly by region and season. SHAP analysis indicated that temperature, surface pressure and boundary layer height were the dominant meteorological factors affecting long-term PM2.5 with contributions of 65%, 11% and 6% on average. We also found that regions with larger average PM2.5 concentration were more affected by emission changes than by meteorology. Therefore, we recommend the continuation of emission control measures in heavily polluted regions such as North and Central China.
更多查看译文
关键词
PM2.5,Meteorological influence,KZ filter,ANN model,SHAP
AI 理解论文
溯源树
样例
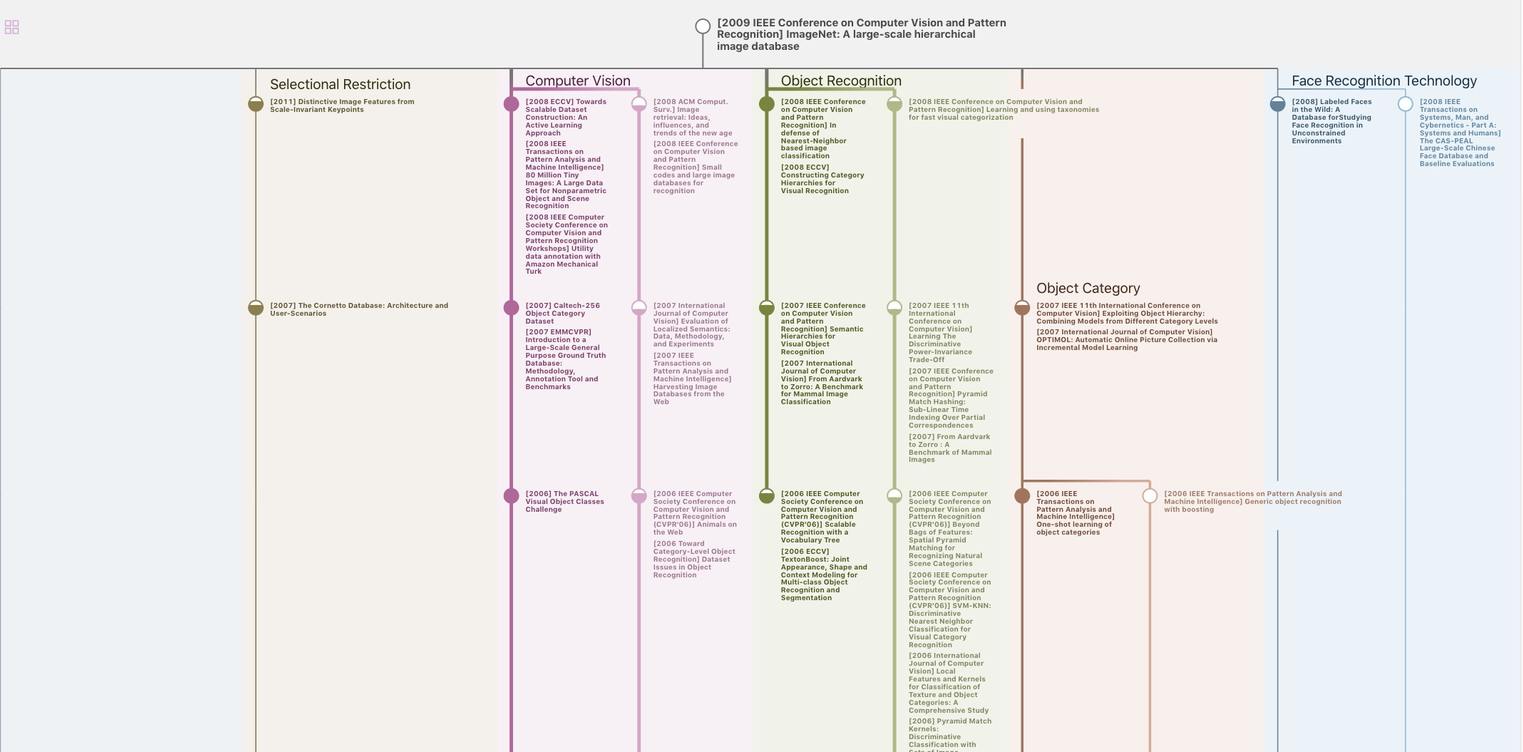
生成溯源树,研究论文发展脉络
Chat Paper
正在生成论文摘要