Exploiting Unfairness With Meta-Set Learning for Chronological Age Estimation.
IEEE Trans. Inf. Forensics Secur.(2023)
摘要
Facial age estimation aims to rank the face aging data by taking in the correlation among age categories. Conventional age estimation models are trained based on assumed high-quality training annotations in a totally-supervised manner. However, noisy data in a sparse distribution collected from unconstrained environment usually account for the corruption of produced gradients and ordinal relationships, which may fail to fairly describe the correlated face aging data. In this paper, we propose a meta-set learning (MSL) approach for exploiting the unfairness of face aging datasets, thus achieving unbiased age classification in unconstrained conditions. To address this, we elaborately create an unfairness filtration network under the meta-learning paradigm, which exceeds a reliable margin-reweighting initialization suffering from class variance, simultaneously exploiting the meta-reweighting intervention to minimize the training bias caused by class imbalance. Moreover, our proposed model leverages the learned instance-level margin between logits and develops a unimodal constrained logits loss, further surviving age regression models from unfairness. Experimental results on multiple in-the-wild datasets demonstrate that our proposed method achieves superior results compared to existing state-of-the-art methods.
更多查看译文
关键词
Training, Estimation, Noise measurement, Aging, Face recognition, Task analysis, Metalearning, Facial age estimation, meta-learning, learning from noisy labels, long-tail learning, biometrics
AI 理解论文
溯源树
样例
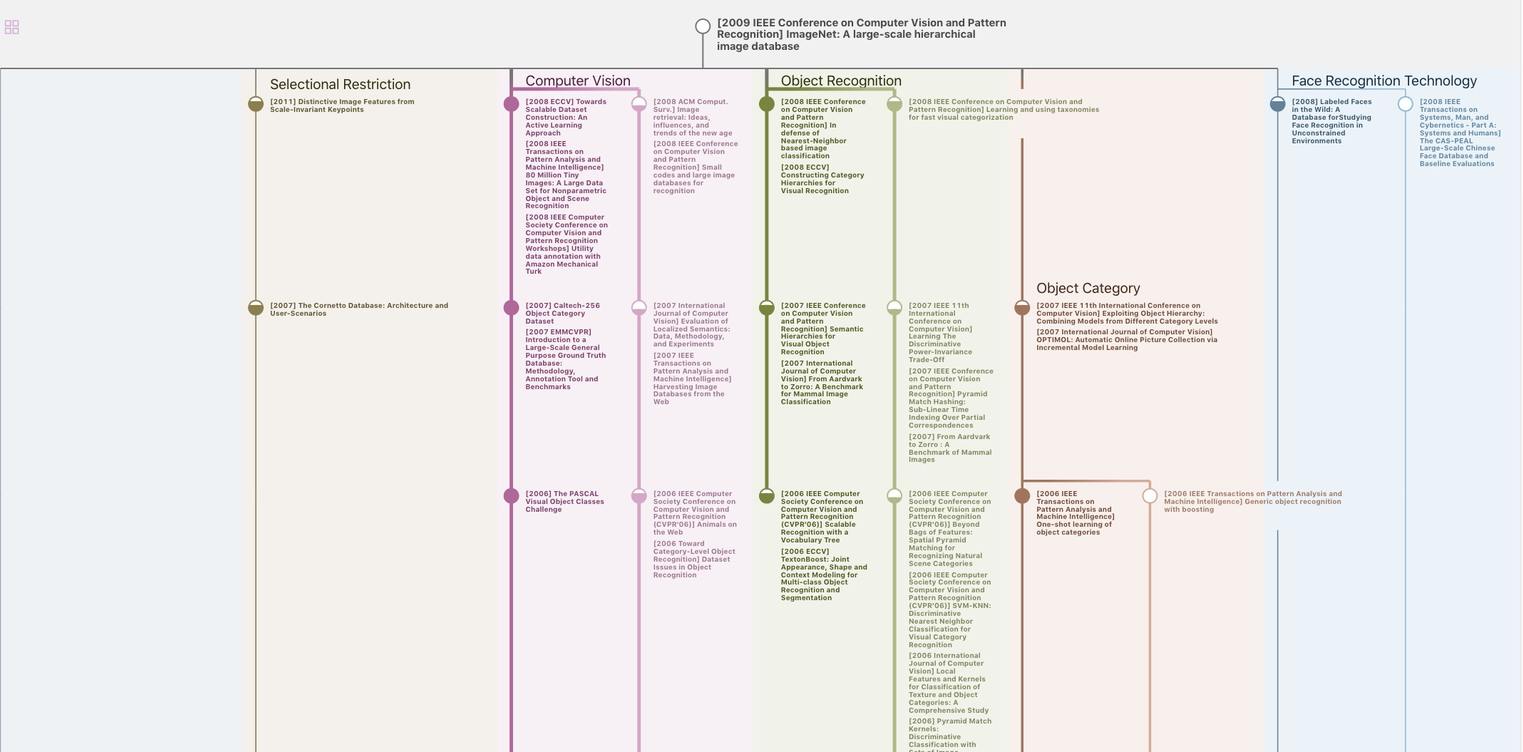
生成溯源树,研究论文发展脉络
Chat Paper
正在生成论文摘要