Adaptive multi-task evolutionary algorithm based on knowledge reuse
Inf. Sci.(2023)
摘要
Multitask optimisation, which aims to simultaneously handle multiple optimisation problems, has attracted considerable attention for solving many complex optimisation problems. However, as the number of tasks increases, selecting similar tasks and transferring useful knowledge become increasingly difficult tasks, further leading to negative transfers and affecting the algorithm performance. In this study, we propose a knowledge-reuse-based multitask evolutionary algorithm (KR-MTEA), that includes two strategies: knowledge-reuse-based knowledge transfer and adaptive strategies. The knowledge-reuse-based knowledge transfer strategy transforms knowledge to be transferred while considering the convergence interval of the decision variables and makes it more suitable for the current task. For the adaptive strategy, the concept of advantage is proposed to adapt to similar tasks and transfer probabilities. In addition, the proposed knowledge transfer strategy is applied to the self-evolution within the task to improve the performance of the KR-MTEA. On four benchmark test suites with 2, 10, 50, and 50 tasks, the KR-MTEA demonstrated its competitiveness in solving multitask and many-task optimisation problems compared with other advanced multitask and many-task algorithms.
更多查看译文
关键词
Multi-task optimization (MTO),Many-task optimisation (MaTO),Adaptive strategy,Knowledge transfer
AI 理解论文
溯源树
样例
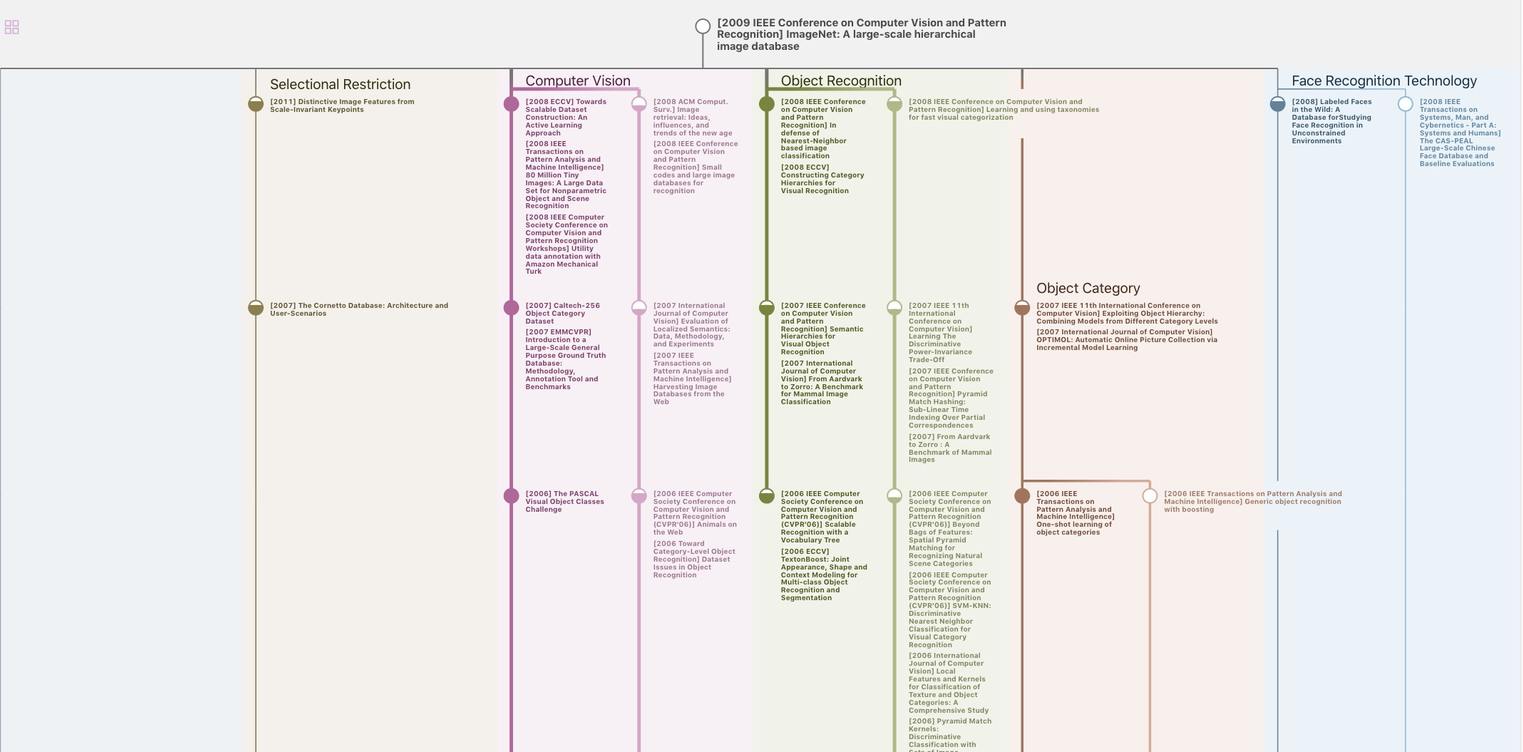
生成溯源树,研究论文发展脉络
Chat Paper
正在生成论文摘要