Ovarian cancer detection in computed tomography images using ensembled deep optimized learning classifier.
Concurr. Comput. Pract. Exp.(2023)
摘要
Ovarian cancer (OC) is one of the most common deadly diseases threatening women worldwide. In day to day life, a challenging task still exists for identifying OC in the early stages. There are different existing deep learning (DL) classification methods applied for OC detection but has some limitations: difficult to locate the exact position of the tumor and more complex. In order to overcome these problems, the proposed ensemble deep optimized classifier-improved aquila optimization (EDOC-IAO) classifier is introduced to detect different types of OC in computed tomography images. The image is resized and filtered in pre-processing using the modified wiener filter (MWF). The pre-processed image is given to the optimized ensemble classifier (ResNet, VGG-16, and LeNet). The IAO is utilized for improving accuracy and overfitting. The fusion is done by average weighted fusion (AWF), and the image features are extracted. Finally, the softmax layer performs the OC classification and detects different ovarian tumor classes. Python is the simulation tool used. The open source TCGA-OV dataset is used for OC classification. The proposed OC classification using the EDOC-IAO model obtained higher accuracy (96.532%) for detecting OC than other methods.
更多查看译文
关键词
aqila optimization, average weighted fusion, computer tomography, deep learning, ensemble classifier, medical imaging, ovarian cancer
AI 理解论文
溯源树
样例
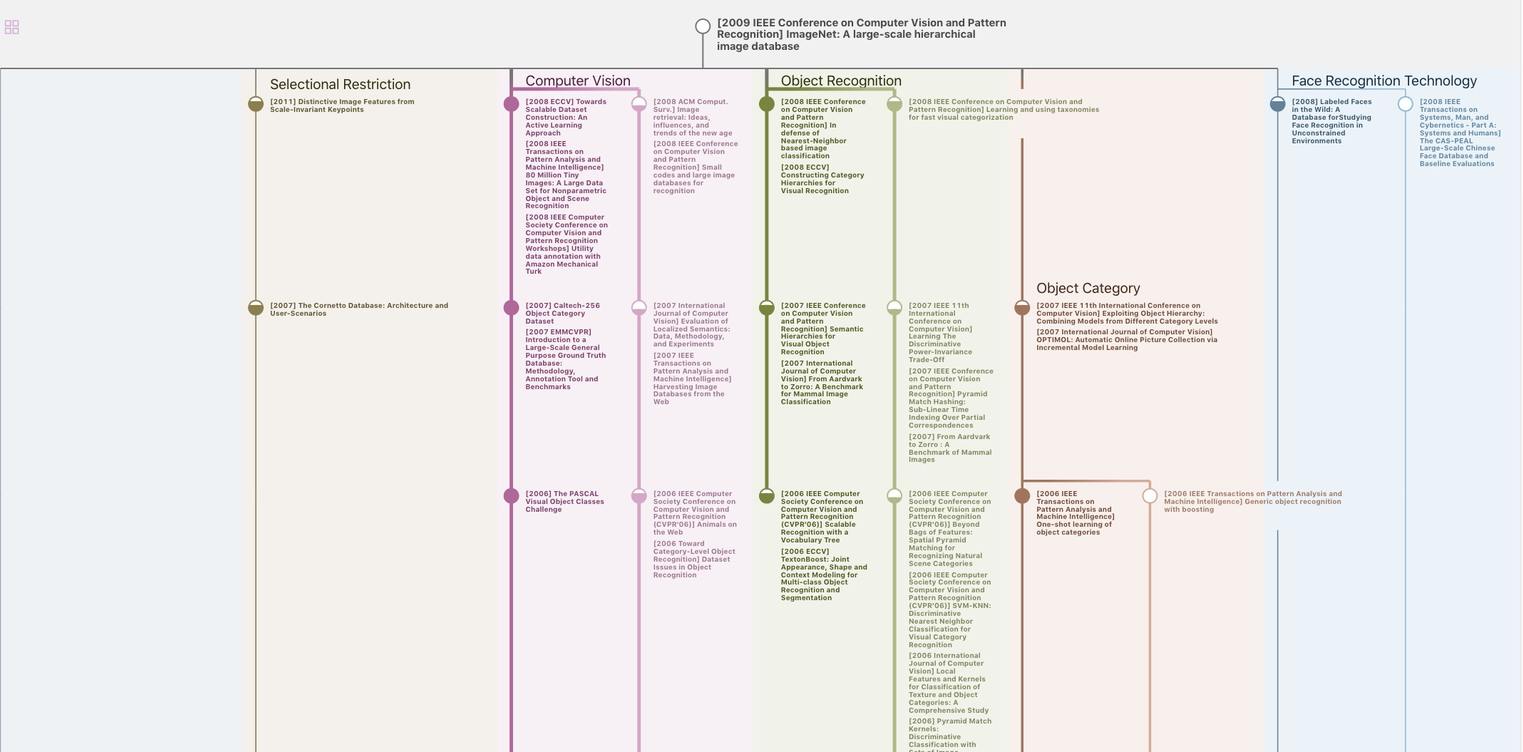
生成溯源树,研究论文发展脉络
Chat Paper
正在生成论文摘要