ACGT-Net: Adaptive Cuckoo Refinement-Based Graph Transfer Network for Hyperspectral Image Classification.
IEEE Trans. Geosci. Remote. Sens.(2023)
摘要
Deep learning (DL) has brought many new trends for hyperspectral image classification (HIC). Graph neural networks (GNNs) are models that fuse DL and structured data. Although GNN-based methods have focused on modeling relations, most of them are susceptible to noise, being adverse to capturing hidden correlations from data. Moreover, the existing related approaches typically adopt changeless graph structures, which might lead to poor generalization. To solve the problems mentioned above, this article develops an adaptive cuckoo refinement-based graph transfer network (ACGT-Net) that introduces a meta-heuristic optimization strategy to refine the graph structure. Specifically, we first pretrain a graph convolutional network (GCN) to learn transferable weight parameters. In the undirected graph, nodes are associated with pixels, and edges correspond to similarities between nodes. Afterward, we integrate a cuckoo search strategy (CSS) into the trained GCN to adaptively refine the graph structure. The graph structure refinement (GSR) with the CSS can pay more attention to significant channels by global optimization to improve the generalization of the GNN. Several experiments with real datasets verify the effectiveness and competitiveness of our ACGT-Net compared with other state-of-the-art (SOTA) methods.
更多查看译文
关键词
Cuckoo search strategy (CSS),graph structure refinement (GSR),hyperspectral image classification (HIC),meta-heuristic optimization,transfer learning (TL)
AI 理解论文
溯源树
样例
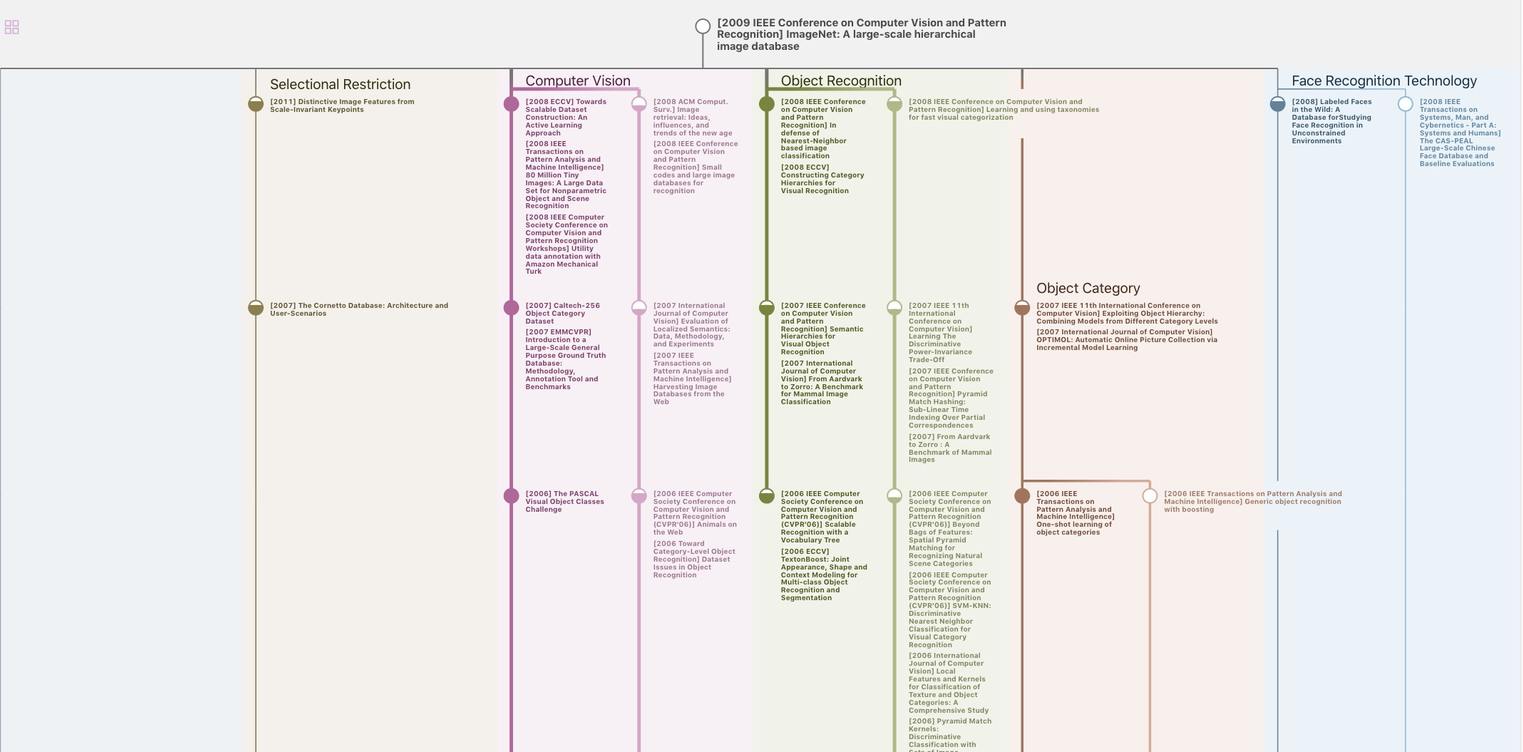
生成溯源树,研究论文发展脉络
Chat Paper
正在生成论文摘要