Fast Hyperspectral Image Classification Combining Transformers and SimAM-Based CNNs.
IEEE Trans. Geosci. Remote. Sens.(2023)
摘要
Convolutional neural networks (CNNs) have been widely employed for hyperspectral image (HSI) classification due to their powerful ability to extract local spatial features. However, CNN-based methods cannot establish long-range dependencies among sequences of pixels. Transformers offer significant advantages when processing sequential data and can establish global relationships, but they still encounter a number of challenges, such as their limited spatial feature extraction ability, or their high computational cost. In order to address the aforementioned issues, we develop a new fast HSI classification approach combining transformers and SimAM-based CNNs. The latter is utilized to extract better spatial features, where the complex spatial characteristics of HSIs are retrieved using an improved hierarchical 2-D dense network structure. A dual attention unit (DAU) mechanism is then utilized to direct the model’s attention to discriminative spatial pixel characteristics and effective feature map channels while suppressing information that is irrelevant for classification purposes. Regarding the spectral features, after extracting hierarchical local characteristics from various convolutional layers (using the hierarchical dense network structure), a squeezed-enhanced axial transformer is employed to establish global long-range dependencies while enhancing the ability of the model to extract local detail features in the HSI. Besides, a new Lion optimizer is utilized to improve the classification performance of our model. Our quantitative and comparative experiments on four benchmark datasets demonstrate that the effectiveness of the proposed approach provides better classification results than other state-of-the-art approaches. Moreover, our FTSCN also achieves better classification results than other methods in practical scenarios.
更多查看译文
关键词
classification,simam-based
AI 理解论文
溯源树
样例
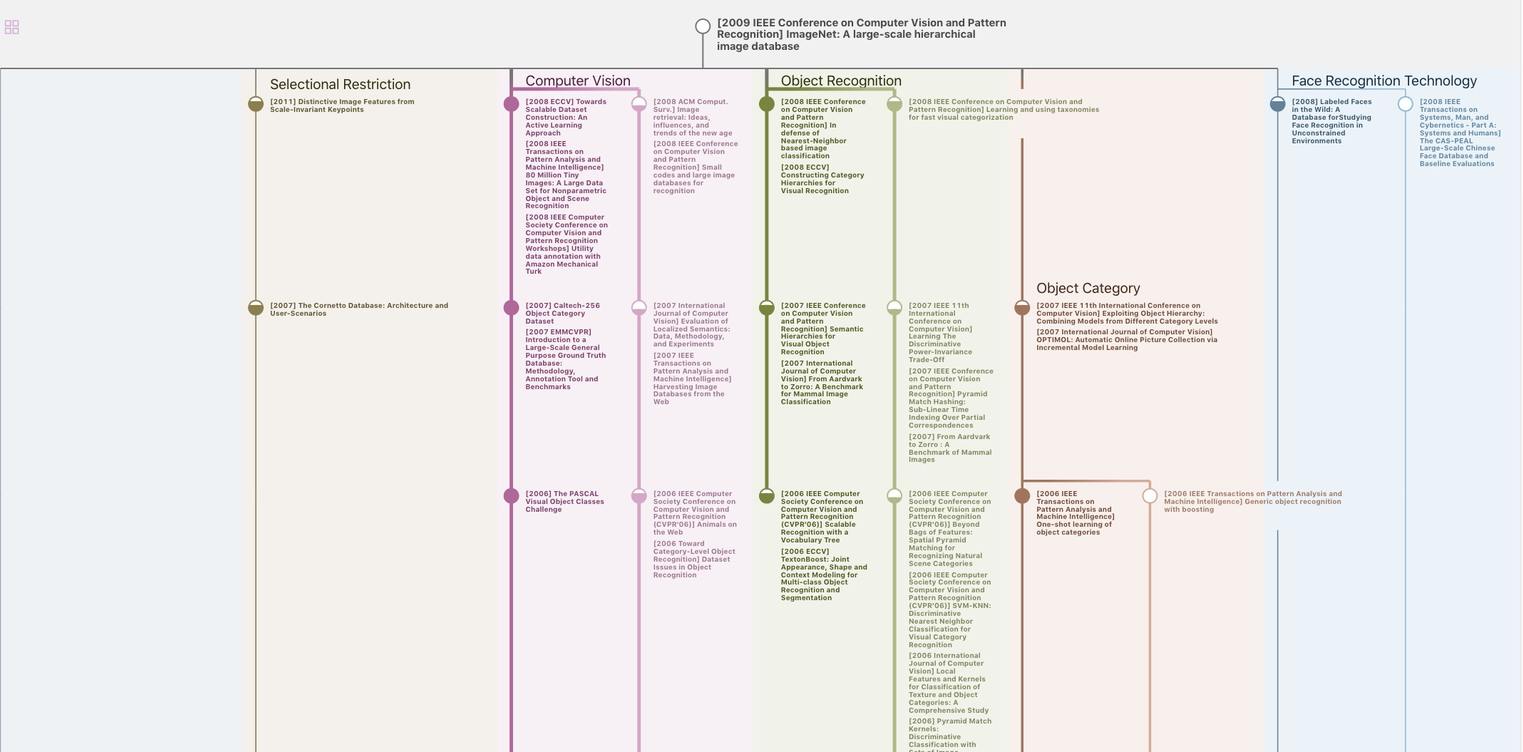
生成溯源树,研究论文发展脉络
Chat Paper
正在生成论文摘要