End-to-end speaker identification research based on multi-scale SincNet and CGAN
Neural Comput. Appl.(2023)
摘要
Deep learning has improved the performance of speaker identification systems in recent years, but it has also presented significant challenges. Typically, data-driven modeling approaches based on DNNs rely on large-scale training data, but due to environmental constraints, large amounts of user speech data are not obtainable. As a result, this work proposes a new SincGAN speaker identification (SI) model that directly recognizes the input’s raw waveform, allowing speaker identification with only a small number of training utterances. Unlike methods that use standard hand-crafted feature recognition, this method is real end-to-end recognition. In this case, a generator is utilized to reconstruct the input samples to enhance the amount of training data, and a discriminator is employed to finish the SI classification task. A multi-scale SincNet layer based on three bespoke filter banks is also added to capture the low-level speech representation of the three channels in the waveform, allowing the model to better catch critical narrowband speaker properties (e.g., pitch and resonance peaks). Experiments reveal that the method achieves better recognition results on the TIMIT and LIBRISPEECH datasets under the constraints of limited training data. Furthermore, the proposed model has a competitive advantage over existing models.
更多查看译文
关键词
Speaker identification, End-to-end, Multi-scale SincNet, Data enhancement, CGAN
AI 理解论文
溯源树
样例
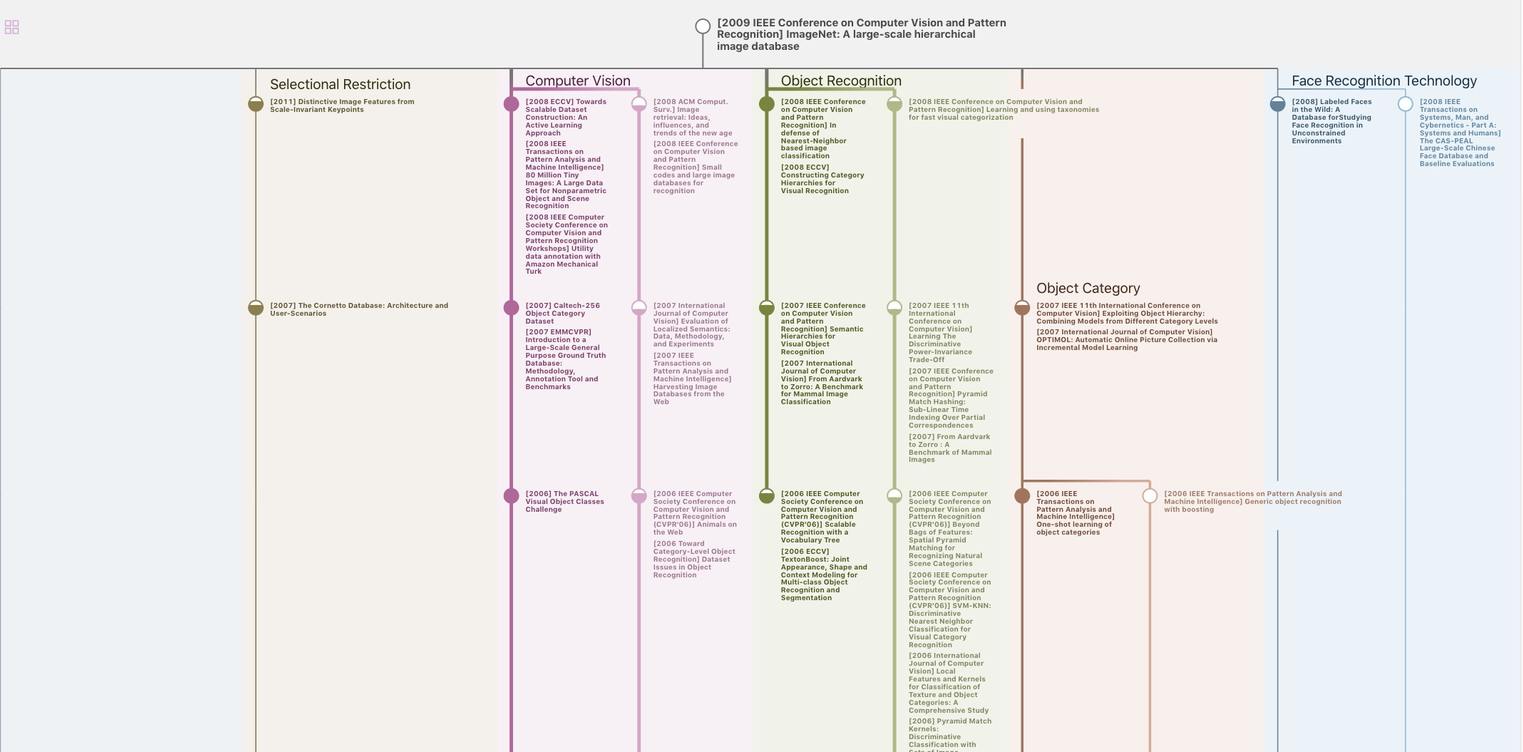
生成溯源树,研究论文发展脉络
Chat Paper
正在生成论文摘要