Cooperative Lidar Sensing for Pedestrian Detection: Data Association Based on Message Passing Neural Networks.
IEEE Trans. Signal Process.(2023)
摘要
This paper considers the problem of cooperative lidar sensing in vehicular networks. We focus on the task of associating the vehicle-generated measurements by lidars to enable a cooperative detection of vulnerable road users. The considered measurements are the three-dimensional bounding boxes extracted from the lidar point cloud. Focusing on a centralized architecture which aggregates and processes all the sensing information, we design a graph formulation of the association problem and we propose a novel solution based on Message Passing Neural Networks (MPNNs). The method has the advantage of accurately learning the associations and the measurement statistics directly from data. We validate the proposed approach on a cooperative sensing scenario simulated by CARLA, an open-source high-fidelity simulator for automated driving scenarios. For the generation of bounding boxes related to pedestrian detections, we consider both artificially-generated and realistic measurements obtained by employing the PointPillars model. We validate the performance by comparing the proposed MPNN model with the Sum-Product Algorithm for Data Association (SPADA), a common approach for data association in multisensor systems. The proposed data-driven MPNN model achieves an association accuracy above 99% and outperforms SPADA in case of moderate sensing errors, as foreseen by automated driving scenarios. We also assess the efficacy of data association in case of mis-modeling between training and testing datasets, observing good generalization capabilities when dealing with untrained conditions.
更多查看译文
关键词
cooperative lidar sensing,pedestrian detection
AI 理解论文
溯源树
样例
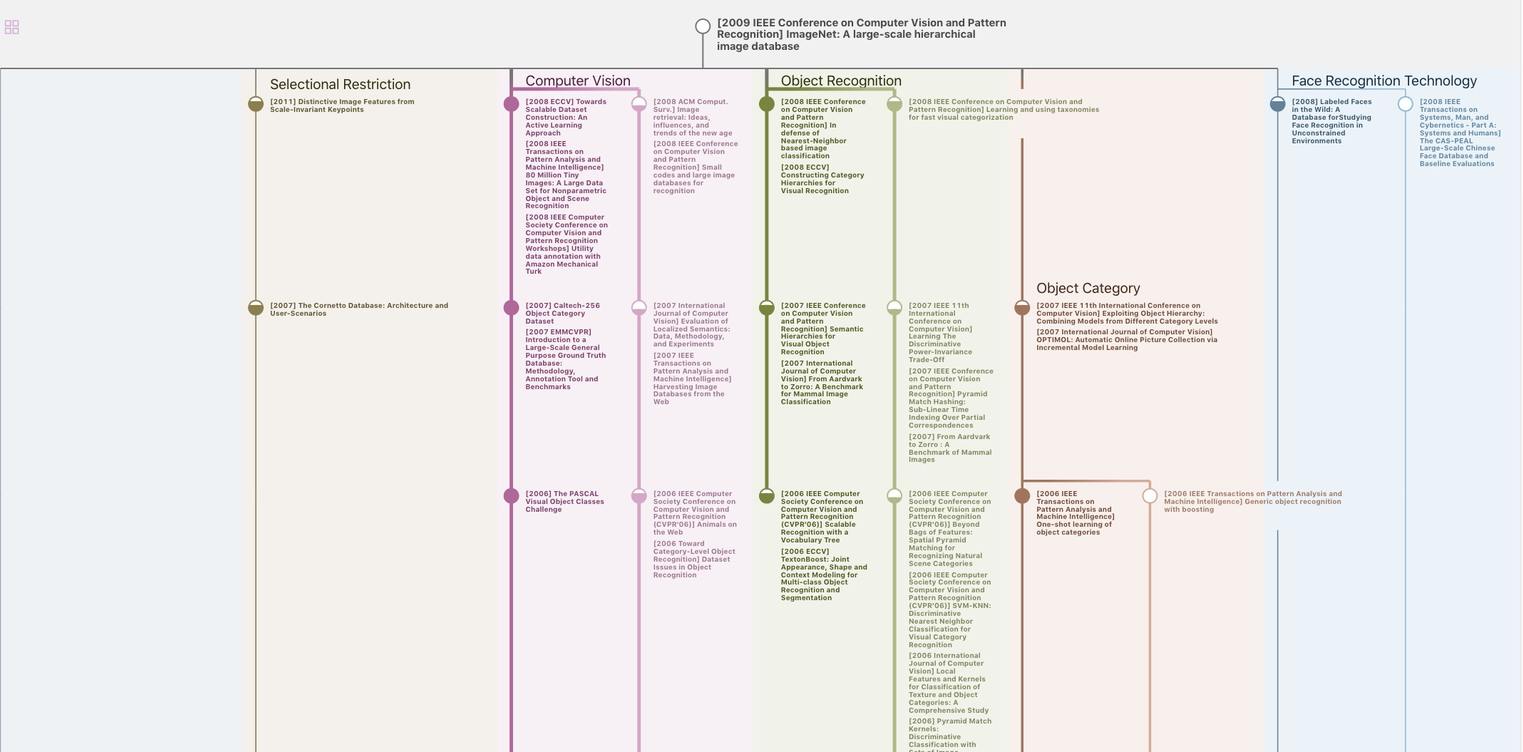
生成溯源树,研究论文发展脉络
Chat Paper
正在生成论文摘要