Comparing Goal-Oriented Analysis Techniques: A Controlled Experiment.
MODELSWARD (Revised Selected Papers)(2022)
摘要
Background: Goal models are usually used during the early phases of requirement elicitation, since they help to understand the motivations underlying the system to be developed. Goal-oriented analysis techniques help analysts reason and make decisions regarding the analyzed goal model. Aims: In this paper, we present an empirical evaluation of two goal-oriented analysis techniques: i) VeGAn, which we proposed in a previous work, and which follows a value-driven approach and a fuzzy logic approach and ii) GRL-Quant, which follows a value-neutral approach and a quantitative approach. Method: We conducted an experiment with a population of 64 Computer Science undergraduate students. The participants were asked to analyze a goal model with one of the techniques and to answer a questionnaire to assess their perceptions. The techniques were compared with respect to the accuracy of goal model element prioritization, the participants’ prioritization time, and their perceptions of the quality of the analysis results (perceived satisfaction), ease of use, usefulness, and intention to use. Results: The results of the experiment show that both techniques are very similar since no significant differences could be found in most of the variables analyzed. However, the participants perceived the results of VeGAn more satisfactorily than those of GRL-Quant, although the prioritization accuracy of GRL-Quant was better for one particular system. Conclusions: This paper provides new insights have emerged from this study, and also opportunities to improve both techniques. The experiment provides preliminary results on the usefulness of both goal-oriented analysis techniques, but further research is required in order to strengthen these results.
更多查看译文
关键词
analysis techniques,controlled experiment,goal-oriented
AI 理解论文
溯源树
样例
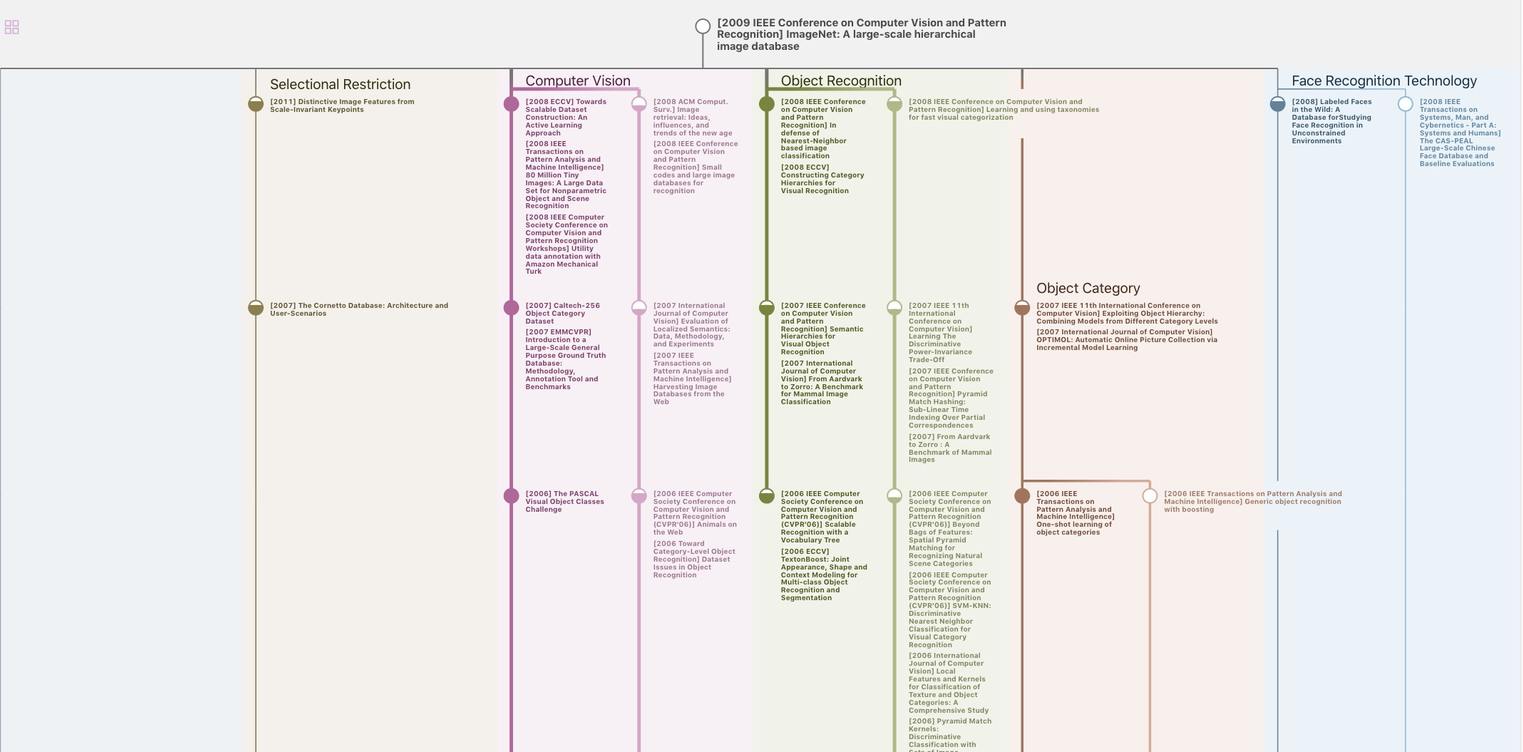
生成溯源树,研究论文发展脉络
Chat Paper
正在生成论文摘要