Impact of Autoencoder Latent Space on IoT CoAP Attack Categorization.
DCAI (3)(2023)
摘要
The Internet of Things (IoT) encompasses a vast network of interconnected devices and systems, usually with limited computational power, memory, and energy resources. These characteristics make IoT ecosystems prone to different cybersecurity problems that must be addressed. Model-based intrusion detection systems (IDS) are one the tools that are being researched and developed to help in the detection of anomalies in IoT networks. This study investigates the behavior and impact of the latent space within an autoencoder, with a focus on developing an optimal decision tree-based classification model integrated into an IoT-specific Intrusion Detection System (IDS). To ensure the effectiveness of the autoencoder, various latent spaces are explored and evaluated. By utilizing a validated IoT dataset that specifically targets the CoAP (Constrained Application Protocol) protocol, we extract an optimized model tailored to detect attacks on this protocol. The results of our comprehensive evaluation demonstrate promising outcomes, validating the effectiveness of the proposed techniques.
更多查看译文
关键词
autoencoder latent space
AI 理解论文
溯源树
样例
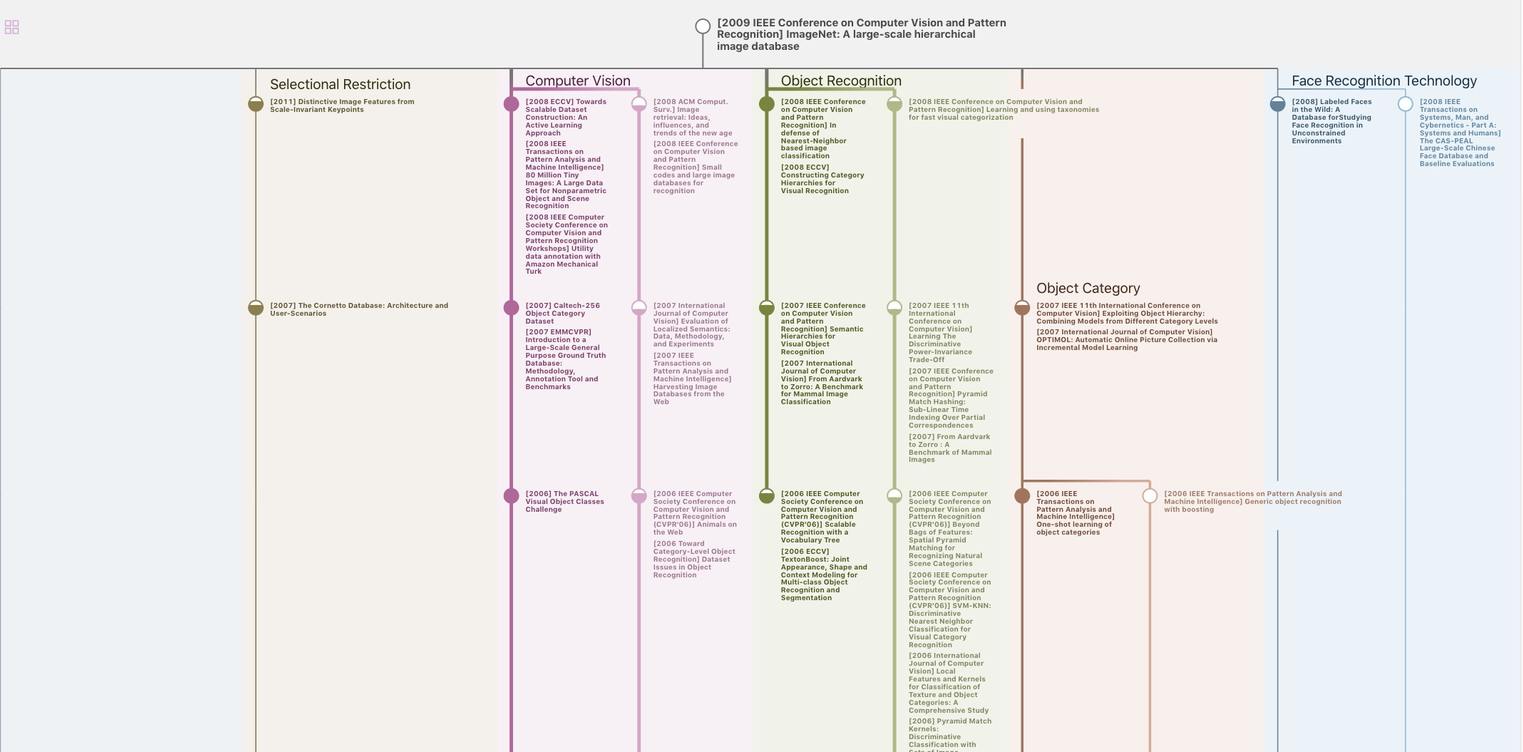
生成溯源树,研究论文发展脉络
Chat Paper
正在生成论文摘要