Pushing the Limits of an FCN and A CRF Towards Near-Ideal Vertebrae Labelling.
ISBI(2023)
摘要
In this work, we propose a simple pipeline for labelling vertebrae in a spine CT image composed of a fully convolutional neural network (FCN) and a conditional random field (CRF). Firstly, we adapt the high-resolution network to work on three-dimensional spine CT images and train them with recent advances in deep learning to regress spatial likelihood maps of the vertebral locations. This sets a strong baseline performance for fully automated identification, resulting in a performance comparable to prior state-of-art. Secondly, we employ a prior-informed CRF conditioned on the predicted likelihood maps of the HRNet, thus refining the location predictions. Our custom FCN-CRF solution produces state-of-the-art results in automated labelling tasks for three benchmark datasets achieving identification rates higher than 97%. Finally, we design an interaction module to perform drag-and-drop correction on the CRF output graph. This semi-automated solution achieves near-100% identification with minimal interaction (measured in actions per scan). Code for this work is published at https://github.com/JannikIrmai/interactive-fcn-crf.
更多查看译文
关键词
landmark detection,spine,vertebrae,fully convolutional neural network,conditional random fields
AI 理解论文
溯源树
样例
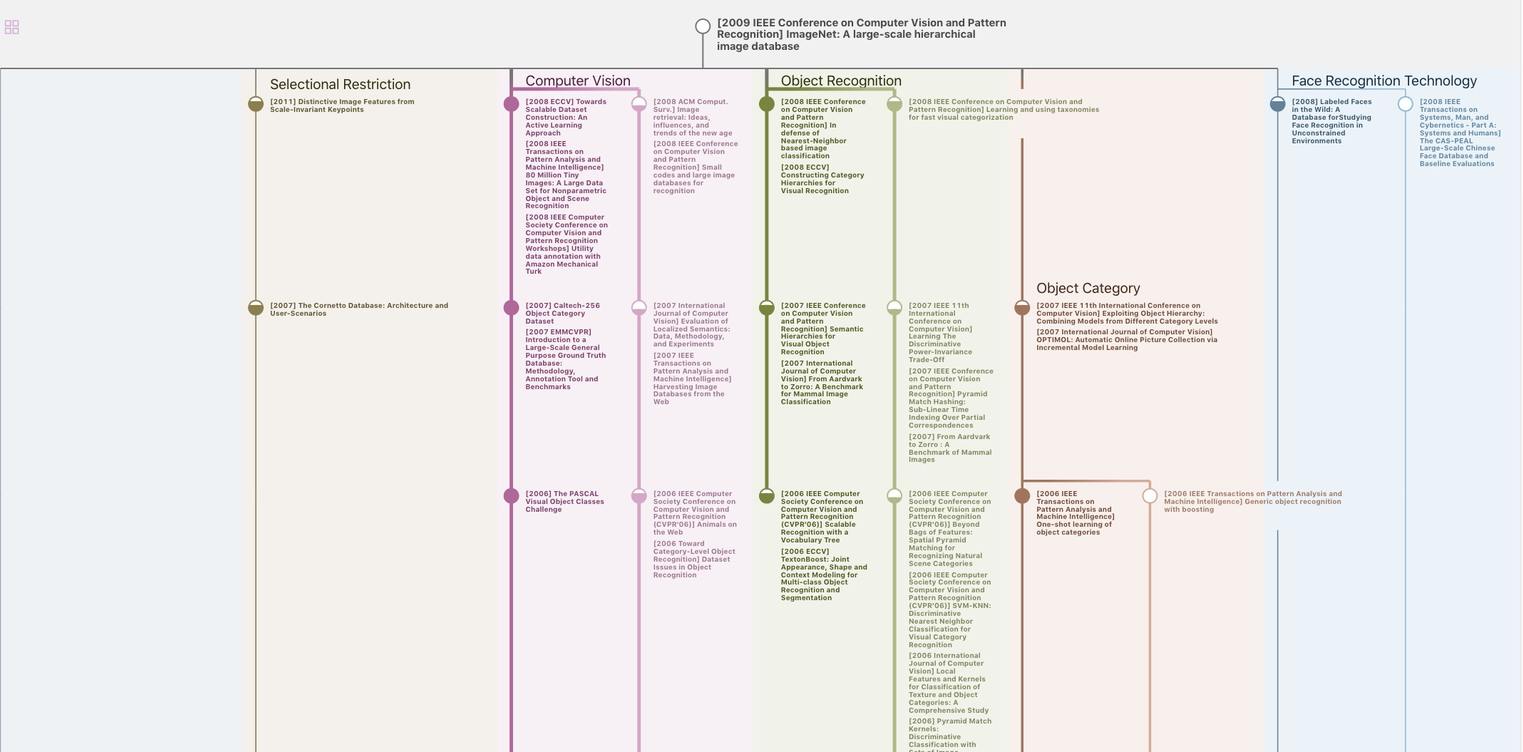
生成溯源树,研究论文发展脉络
Chat Paper
正在生成论文摘要