Multi-Task Curriculum Learning for Partially Labeled Data.
ISBI(2023)
摘要
Incomplete labels are common in multi-task learning for biomedical applications due to several practical difficulties, e.g., expensive annotation efforts by experts, limit of data collection, different sources of data. A naive approach to enable joint learning for partially labeled data is adding self-supervised learning for tasks without ground truths by augmenting an input image and forcing the multi-task model to return the same outputs for both the input and augmented images. However, the partially labeled setting can result in imbalanced learning of tasks since not all tasks are trainable with ground truth supervisions for each data sample. In this work, we propose a multi-task curriculum learning method tailored for partially labeled data. For balanced learning of tasks, our multi-task curriculum prioritizes less performing tasks during training by setting different supervised learning frequencies for each task. We demonstrate that our method outperforms standard approaches on one biomedical and two natural image datasets. Furthermore, our learning method with partially labeled data performs better than the standard multi-task learning methods with fully labeled data for the same number of annotations.
更多查看译文
关键词
balanced learning,biomedical applications,data collection,data sample,different supervised learning frequencies,fully labeled data,imbalanced learning,joint learning,learning method,multitask curriculum learning method,multitask model,naive approach,partially labeled data,self-supervised learning,standard multitask learning methods
AI 理解论文
溯源树
样例
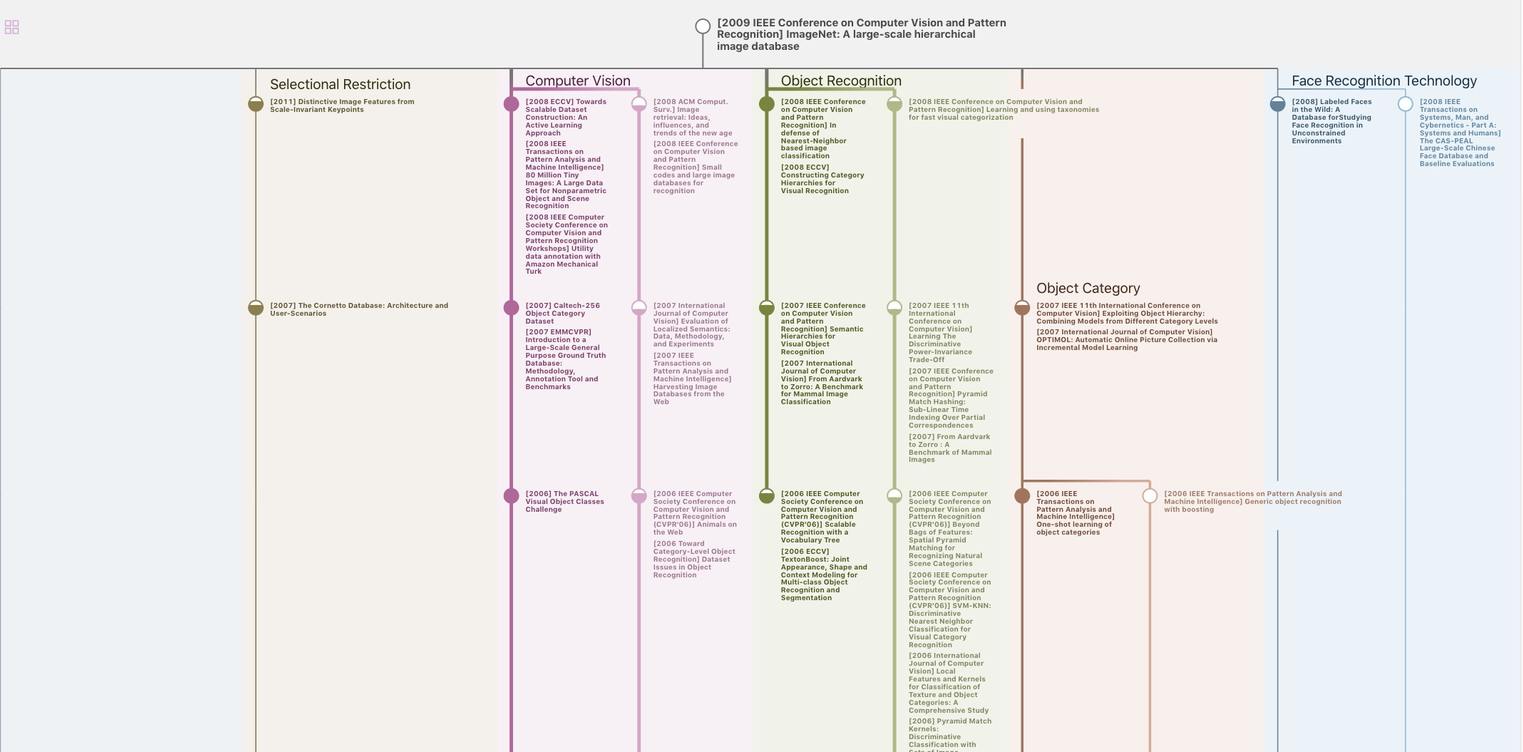
生成溯源树,研究论文发展脉络
Chat Paper
正在生成论文摘要