Qualitative Models of Data Generation Processes: Facilitating Data-Intensive AI Solutions.
FUSION(2023)
摘要
AI-based decision support solutions require life cycles that adequately address critical steps, such as (i) finding suitable machine learning (ML) methods for the problem at hand, (ii) preparing and executing adequate data acquisition processes and (iii) tractable evaluation of the overall solution. Understanding the data generating processes is key in achieving this. Training and test data can be seen as a result of a causal data generation process, a sampling process in which the data is collected from different sources that are influenced by multiple interdependent phenomena. This is represented by a Qualitative Model of Data Generation Processes (QM-DGP), a causal graphical model. QM-DGP facilitates analysis of the complexity of the underlying data generating processes that can inform the development of trustable ML-based solutions in multiple ways. Firstly, this analysis is the basis for the determination of the required complexity of the ML models. Secondly, it facilitates the determination of the quantities of training data supporting good learning results. Thirdly, it can provide guidance for a systematic simplification of the models, supporting tractable solutions without significantly reduced performance. The construction of QM-DGP and the analysis benefit from sound theoretical concepts, such as d-separation and I-Maps. Experimental results with simulated data indicate that the approach can be effective in predicting the required quantities of training data and the determination of the modelling complexity using different types of models.
更多查看译文
AI 理解论文
溯源树
样例
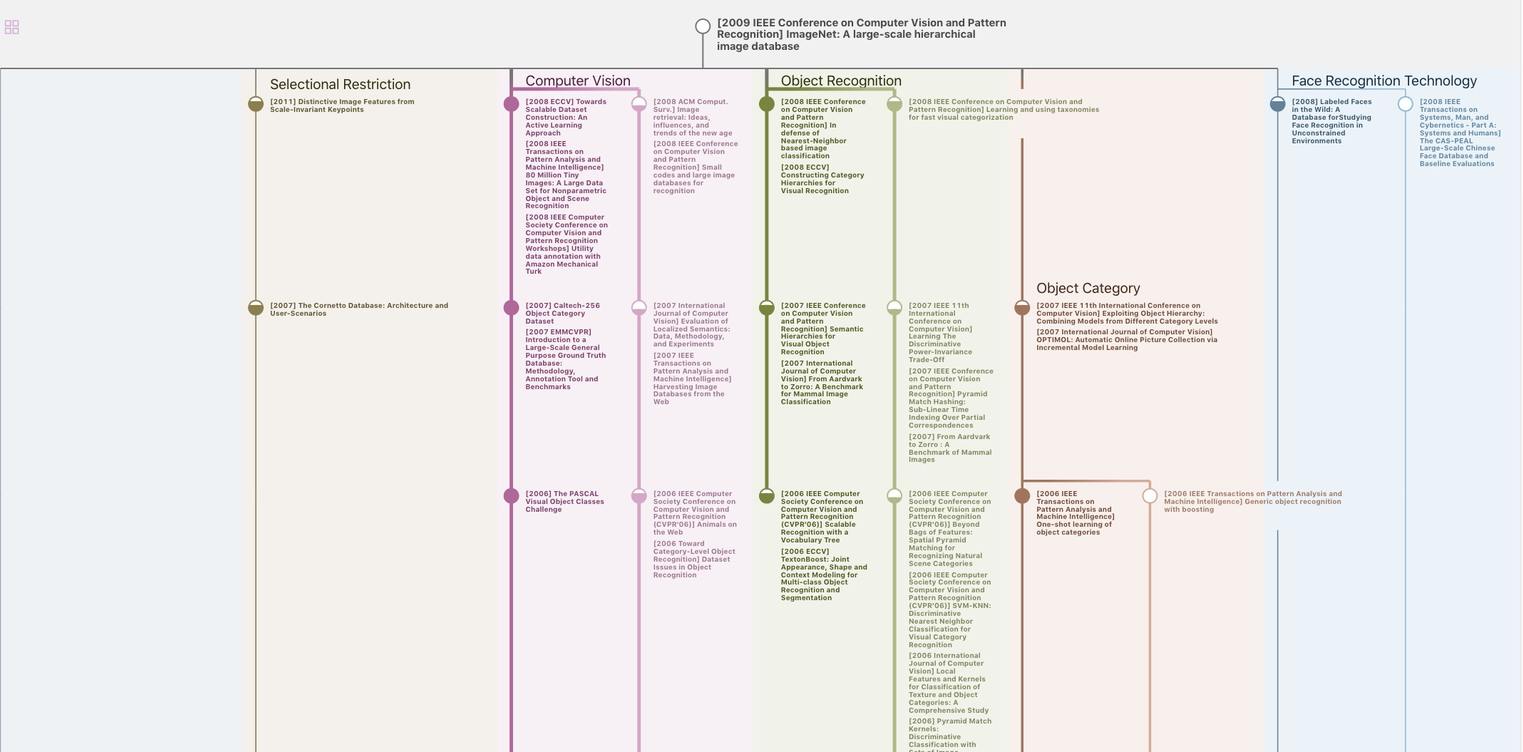
生成溯源树,研究论文发展脉络
Chat Paper
正在生成论文摘要